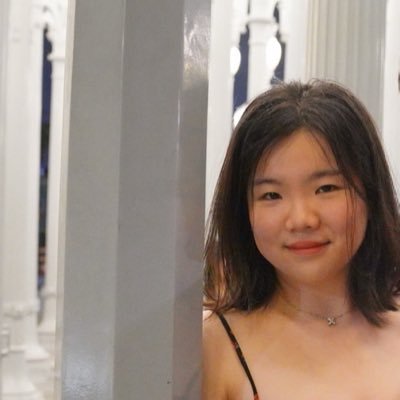
Xinyan Velocity Yu
@XinyanVYu#NLProc PhD @usc, bsms @uwcse | Previously @Meta @Microsoft @Pinterest | Doing random walks in Seattle
Similar User

@jefffhj

@WeijiaShi2

@zhaofeng_wu

@GabrielSaadia

@shangbinfeng

@taoyds

@yizhongwyz

@ChengleiSi

@ZhongRuiqi

@liujc1998

@alisawuffles

@byryuer

@huyushi98

@XiangLisaLi2

@chrome1996
Is the following question directly answerable? We introduce CREPE -- a new QA task for identifying and correcting false presuppositions (backgrounded assumptions) in questions based on world knowledge. arxiv.org/abs/2211.17257 (also @ 12:15 Tuesday @ Metropolitan East #ACL2023)
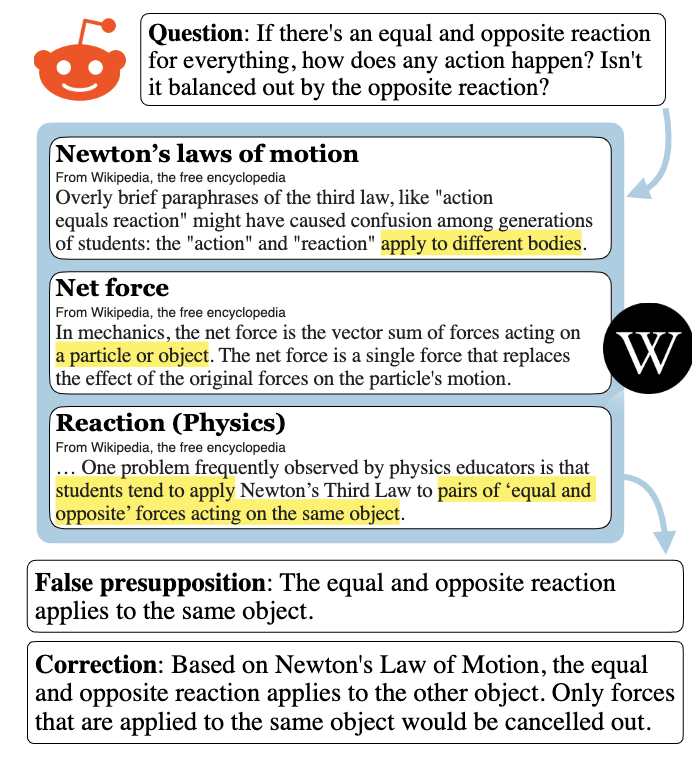
Can't be at EMNLP this year... but can celebrate on being recognized as an outstanding reviewer!! ❤️ I'm so happy!!
We're kicking off the awards session at #EMNLP2024 by announcing our (many) **Outstanding Reviewers**!
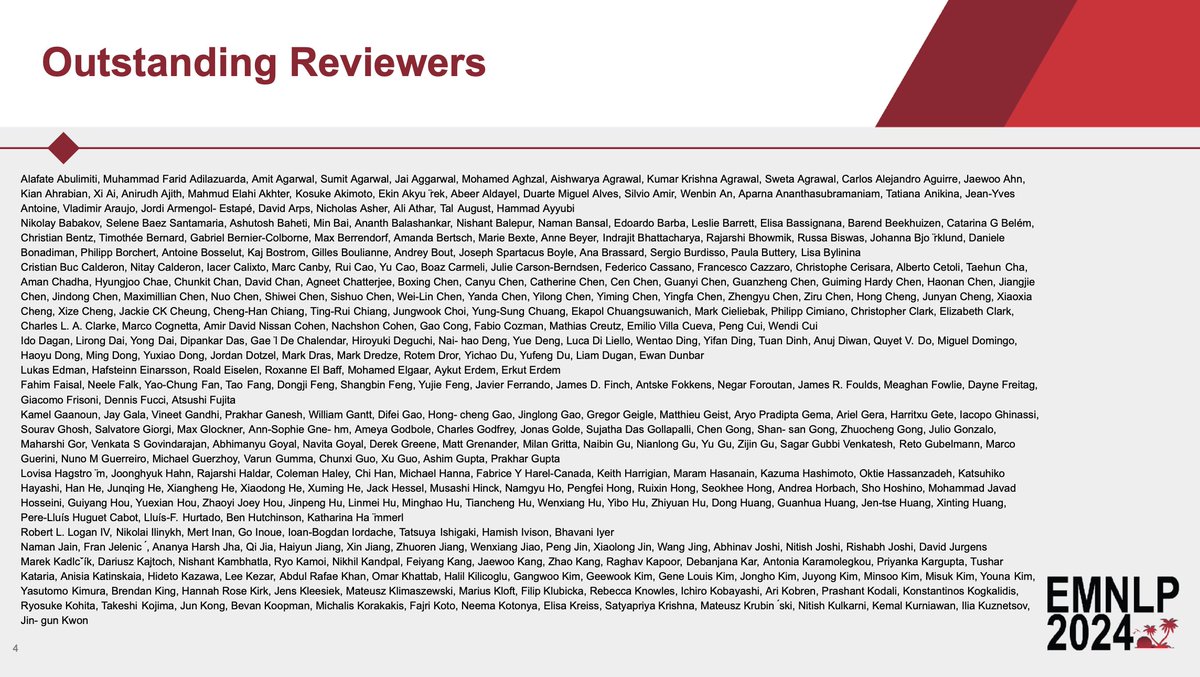
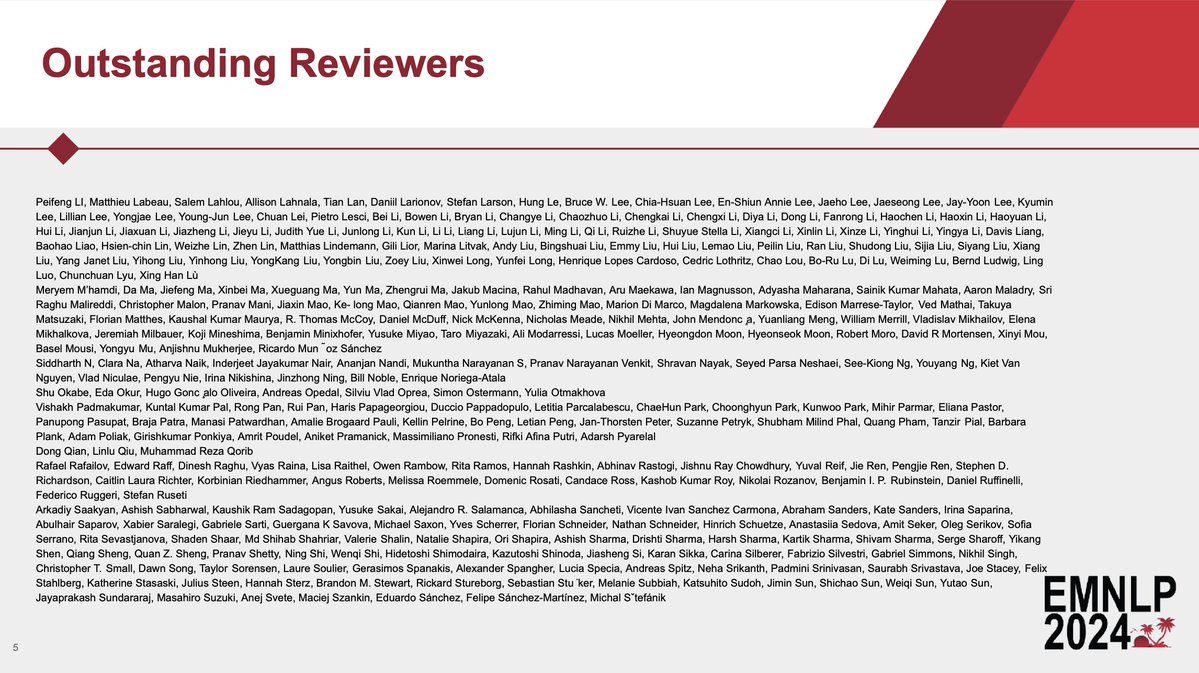
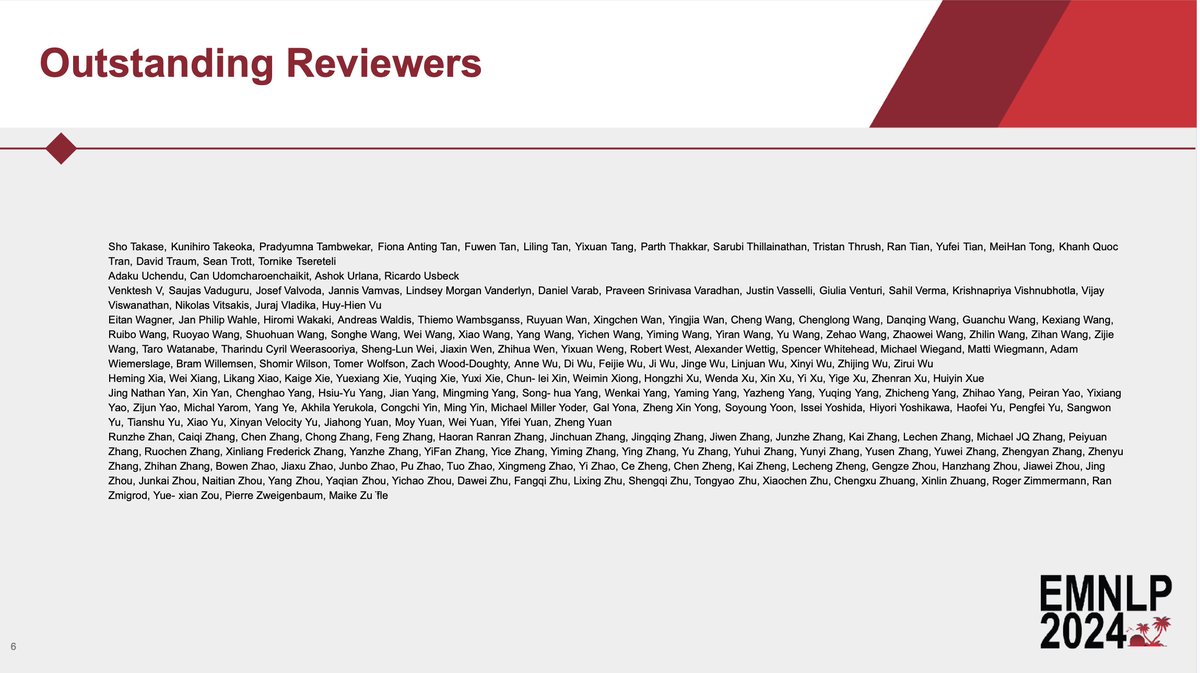
Like how we might have a semantic "hub" in our brain, we find models tend to process🤔non-English & even non-language data (text, code, images, audios.etc) in their dominant language, too! Thank you @zhaofeng_wu for the wonderful collaboration!
💡We find that models “think” 💭 in English (or in general, their dominant language) when processing distinct non-English or even non-language data types 🤯 like texts in other languages, arithmetic expressions, code, visual inputs, & audio inputs ‼️ 🧵⬇️arxiv.org/abs/2411.04986
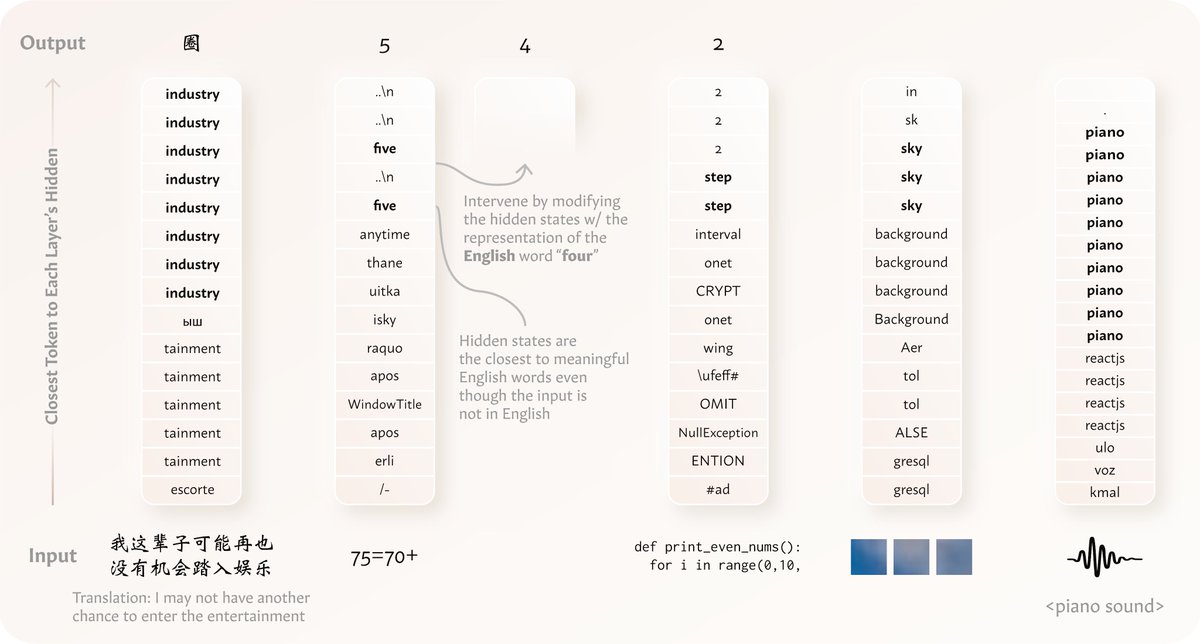
💡We find that models “think” 💭 in English (or in general, their dominant language) when processing distinct non-English or even non-language data types 🤯 like texts in other languages, arithmetic expressions, code, visual inputs, & audio inputs ‼️ 🧵⬇️arxiv.org/abs/2411.04986
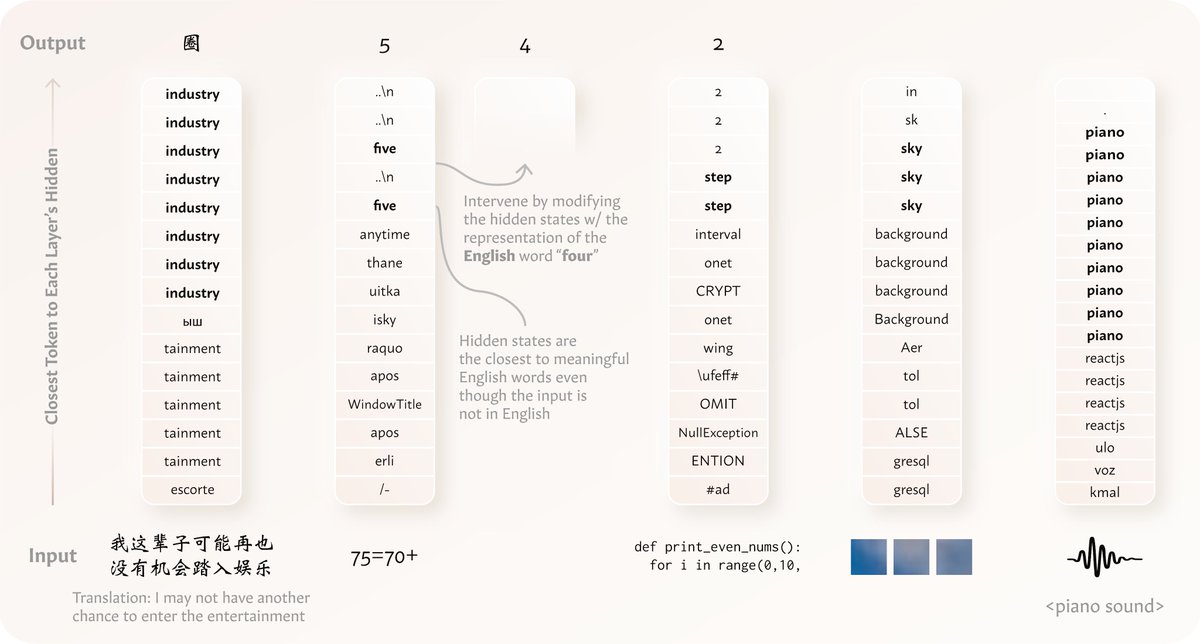
It's cool to see @GoogleDeepMind's new research to show similar findings as we did back in April. IsoBench (isobench.github.io, accepted to @COLM_conf 2024) was curated to show the performance gap across modalities and multimodal models' preference over text modality.…
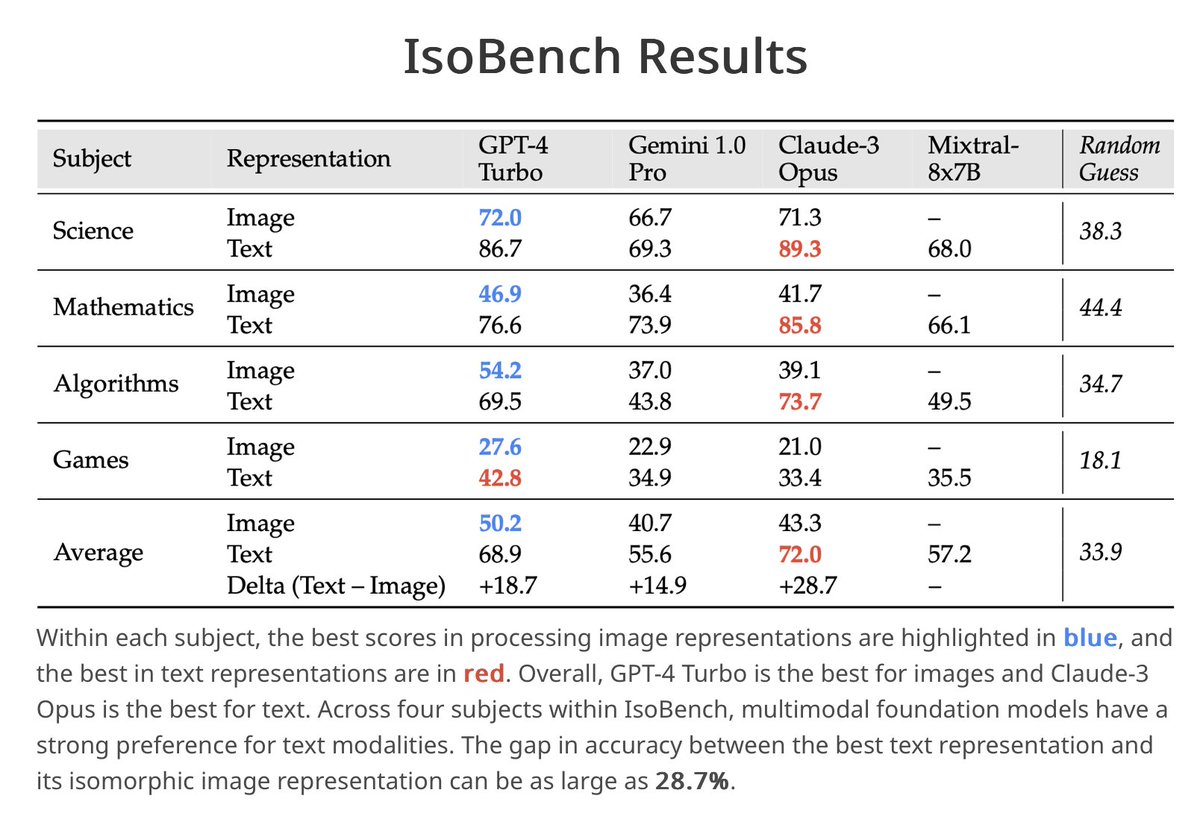
🚨 New research alert! 🚨 Lichang's latest findings reveal a major gap in how OmniLLMs reason and answer the same question when it's presented in different modality (or combinations of them). Even when models understand the question, their performance varies across modalities!…
Do you work in AI? Do you find things uniquely stressful right now, like never before? Haver you ever suffered from a mental illness? Read my personal experience of those challenges here: docs.google.com/document/d/1aE…
Automating AI research is exciting! But can LLMs actually produce novel, expert-level research ideas? After a year-long study, we obtained the first statistically significant conclusion: LLM-generated ideas are more novel than ideas written by expert human researchers.
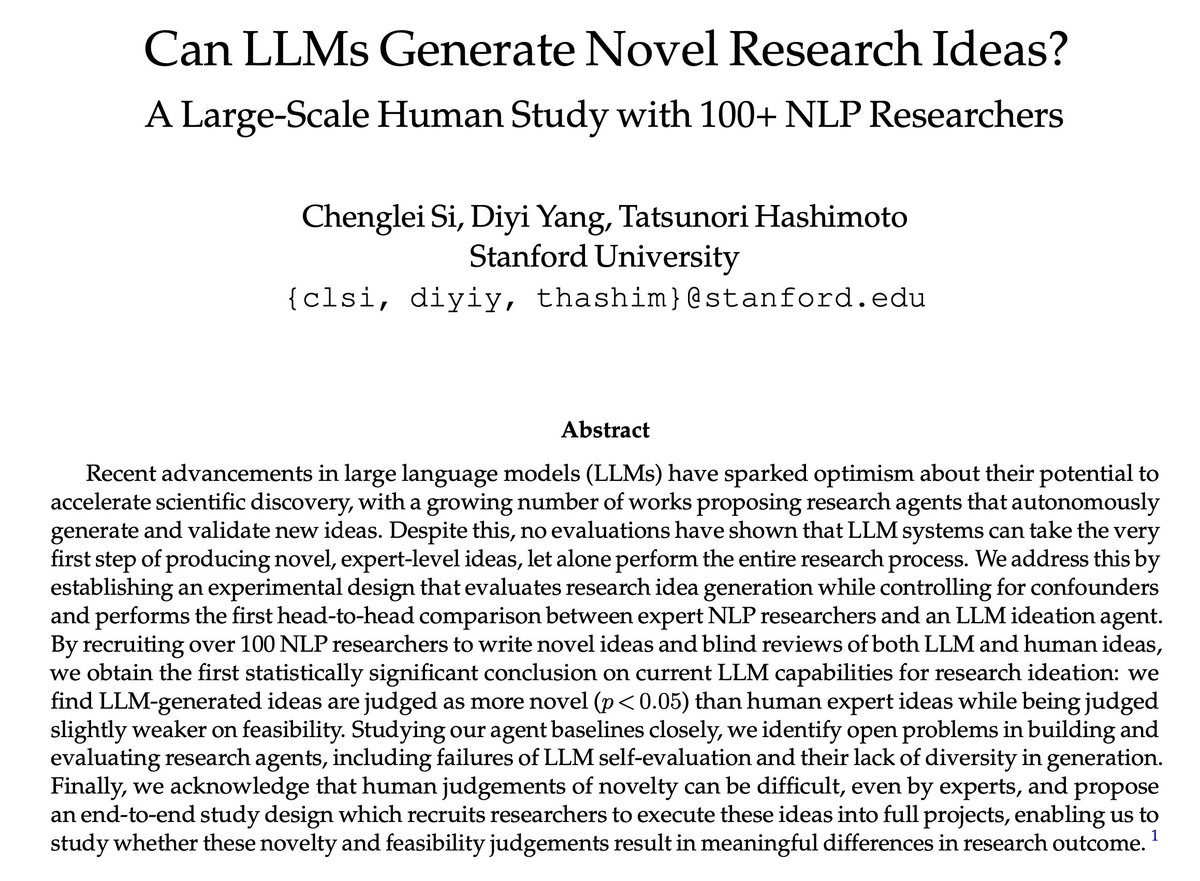
CodeRAG-Bench is extremely meaningful! We experiment with different retrievers, types of retrieval source documents, code generation tasks, and language models to find out how retrieval can help! For more, please read our exciting paper 👉👉
Introducing 🔥CodeRAG-Bench🔥 a benchmark for retrieval-augmented code generation! 🔗arxiv.org/abs/2406.14497 - Supports 8 codegen tasks and 5 retrieval sources - Canonical document annotation for all coding problems - Robust evaluation of retrieval and end-to-end execution
As the world changes, documents go out of date. How can we adapt RAG systems to a stream of changing world data? We introduce ERASE, a way of updating and propagating facts within knowledge bases, and CLARK, a dataset targeting these update problems arxiv.org/abs/2406.11830… 1/
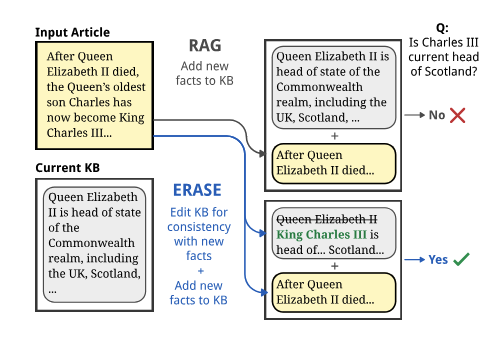
Awesome analysis of what KNN-LM says abt training: Is the seeming "free lunch" of KNN-LM (replacing top LM layers with embedding store and KNN lookup) due to a weakness of the LM objctve? Seems no! Training a replacement MLP on the KNN does better! 🤔 aclanthology.org/2024.naacl-sho…
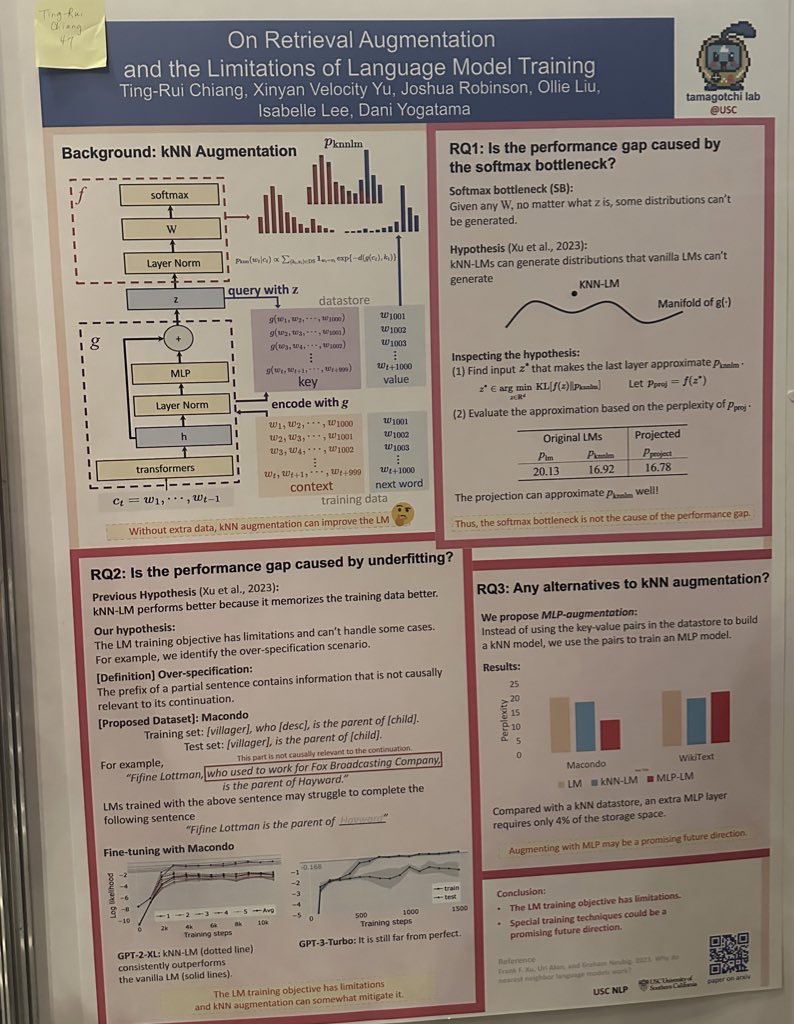
#NAACL2024 @naaclmeeting Reasoning or Reciting? Exploring the Capabilities and Limitations of Language Models Through Counterfactual Tasks Zhaofeng Wu (@zhaofeng_wu) arxiv.org/pdf/2307.02477
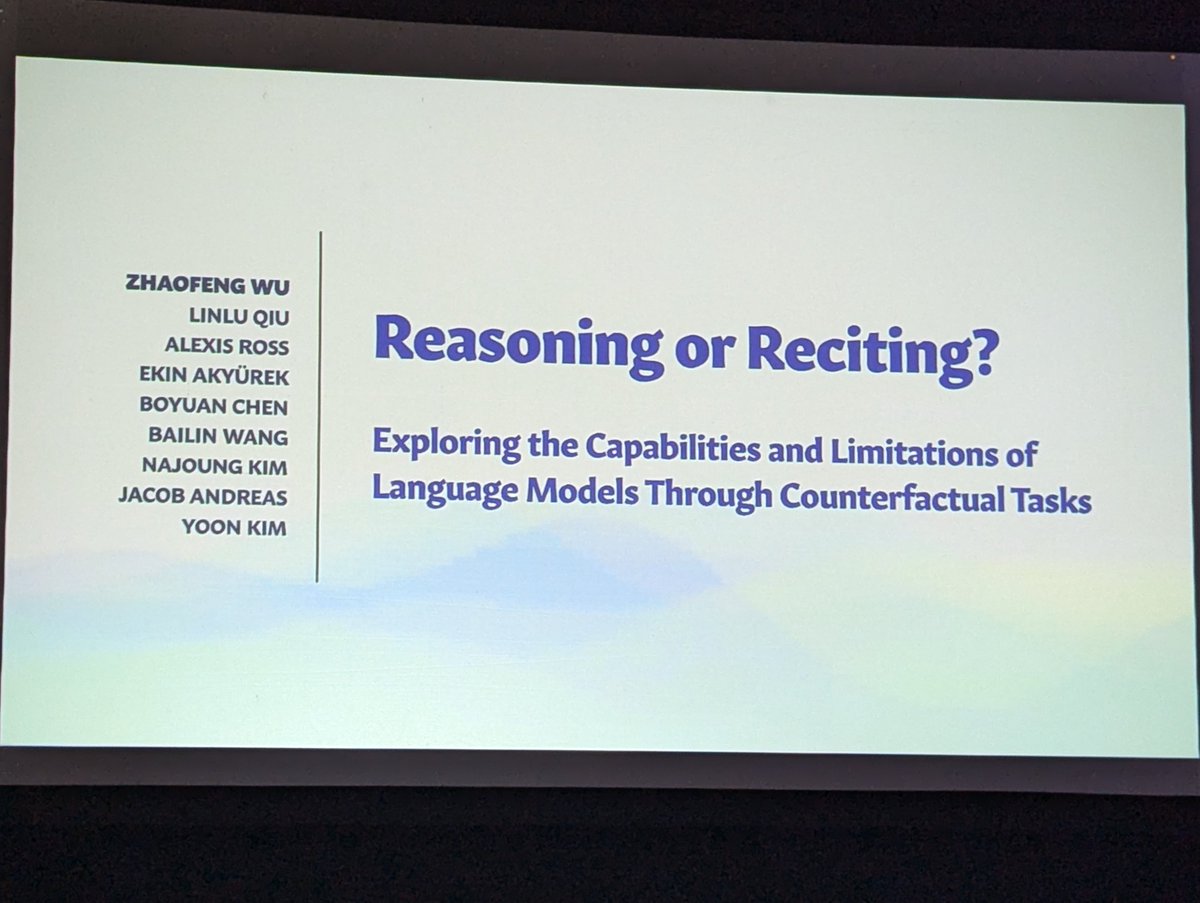
So happy to meet new and old friends in NAACL ❤️! I’ll be presenting our work BUFFET🎉: ⏰ Monday, June 17th at 14:00 📍Don Alberto 4 If you’re into multilinguality and seeking a benchmark for fair comparison of models for both languages & methods, don’t miss it! 🤩 #NAACL2024
New paper 🚨 Can LLMs perform well across languages? Our new benchmark BUFFET enables a fair eval. for few-shot NLP across languages in scale. Surprisingly, LLMs+Incontext learning (incl. ChatGPT) are often outperformed by much smaller fine-tuned LMs 🍽️tinyurl.com/BuffetFS
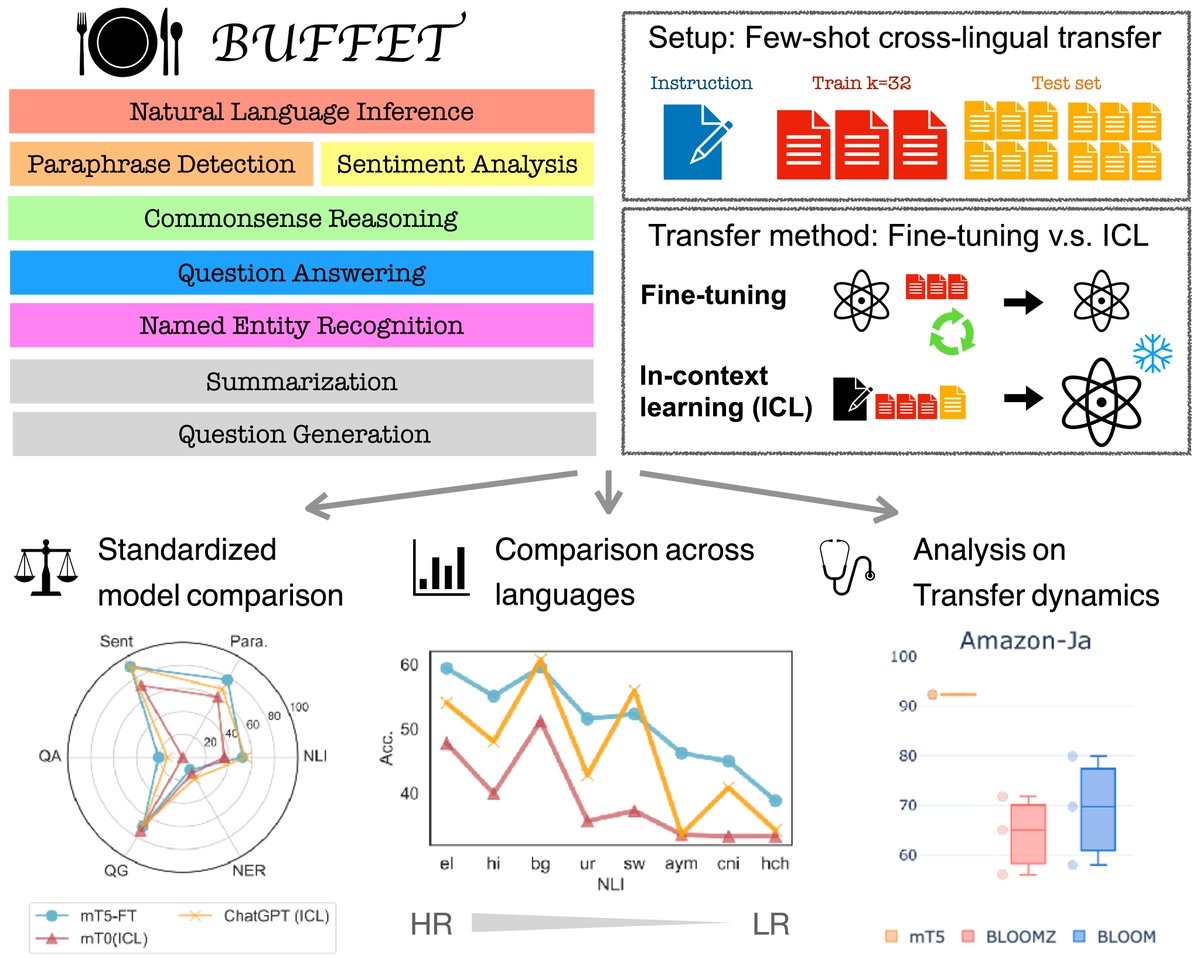
Humans draw to facilitate reasoning and communication. Why not let LLMs do so? 🚀We introduce✏️Sketchpad, which gives multimodal LLMs a sketchpad to draw and facilitate reasoning! arxiv.org/abs/2406.09403 Sketchpad gives GPT-4o great boosts on many vision and math tasks 📈 The…
It is a great pleasure working with Ting-Rui and others on this project to understand retrieval augmentation and LM training a little bit better!
“On Retrieval Augmentation and the Limitations of Language Model Training” (arxiv.org/abs/2311.09615) has been accepted to NAACL 2024! While it is well known that kNN retrieval can decrease LMs’ perplexity, the underlying reason is unclear. We study two hypotheses 👇
My takeaways when figuring out living arrangements: (1) PhD students need to be better paid as 50%-75% of my salary is on rent and commute, (2) accessible and affordable on-campus housing should be given, and (3) learn to drive early and live in less sketchy places. 🥲
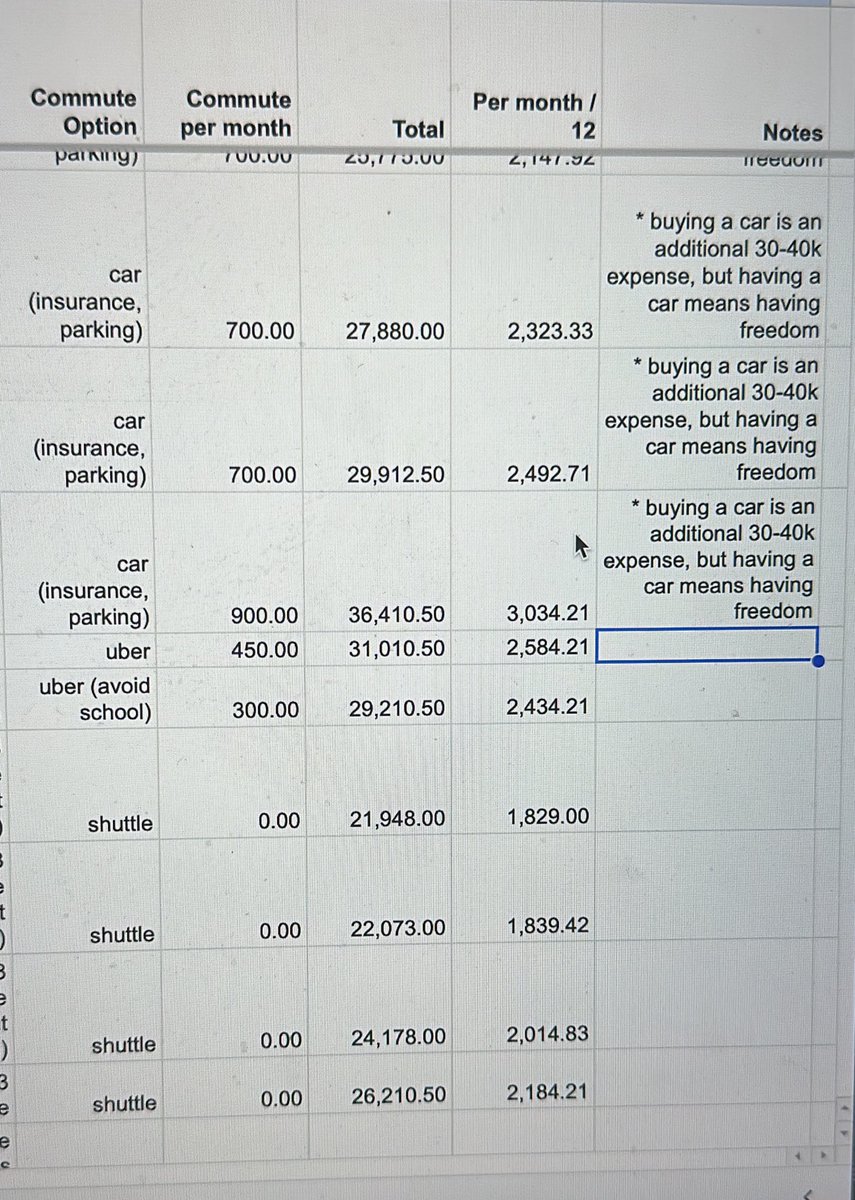
Want to train an aligned LM in a new language 🌏 but don’t have preference data for training the reward model (RM)? 💡 Just use a RM for another language: it often works well, sometimes even BETTER than if you had a RM in your target language! 🤯 arxiv.org/abs/2404.12318
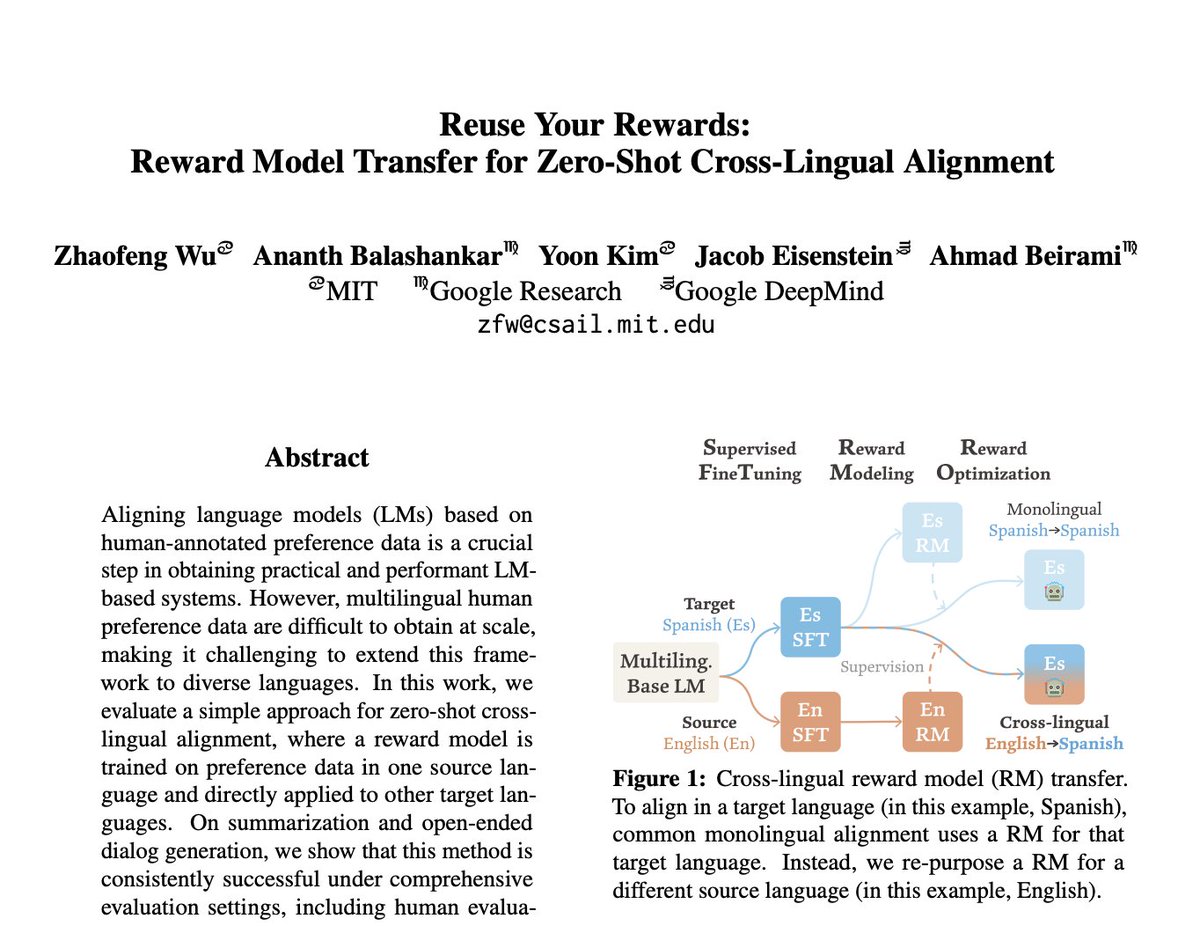
Do multimodal foundation models treat every modality equally? Hint: Humans have picture superiority. How about machines? Introducing IsoBench, a benchmark for multimodal models with isomorphic inputs. 🔗 IsoBench.github.io
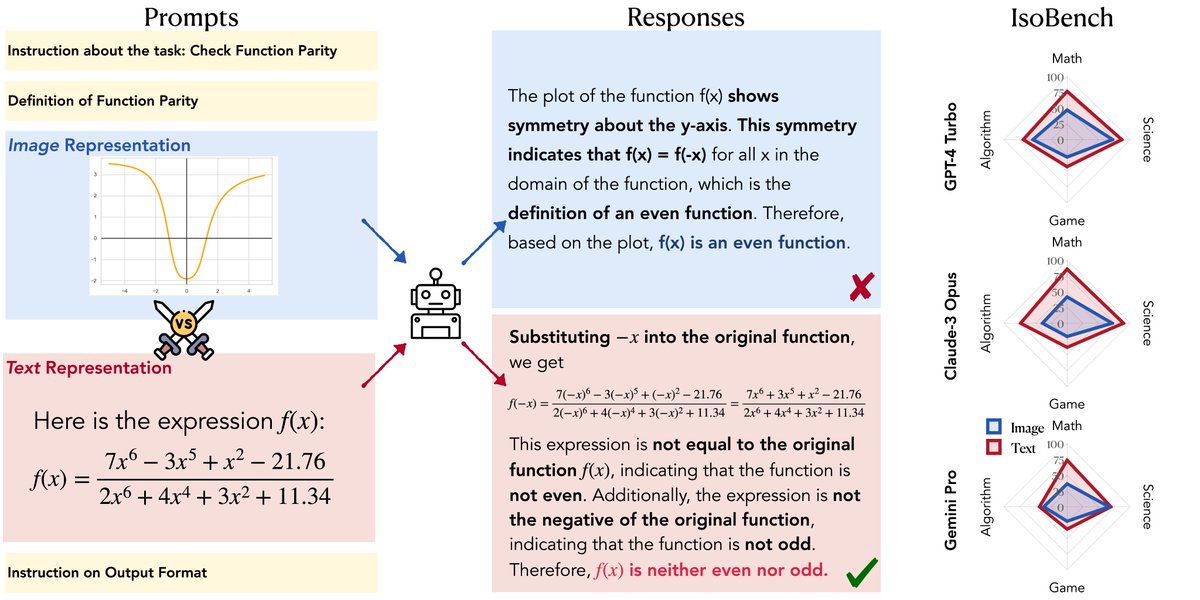
🤔 How much do compositional generalization datasets agree with each other? We compare common compositional generalization benchmarks and find that they rank modeling approaches differently (❗) 🧵👇 #CoNLL2023 arxiv.org/abs/2310.17514
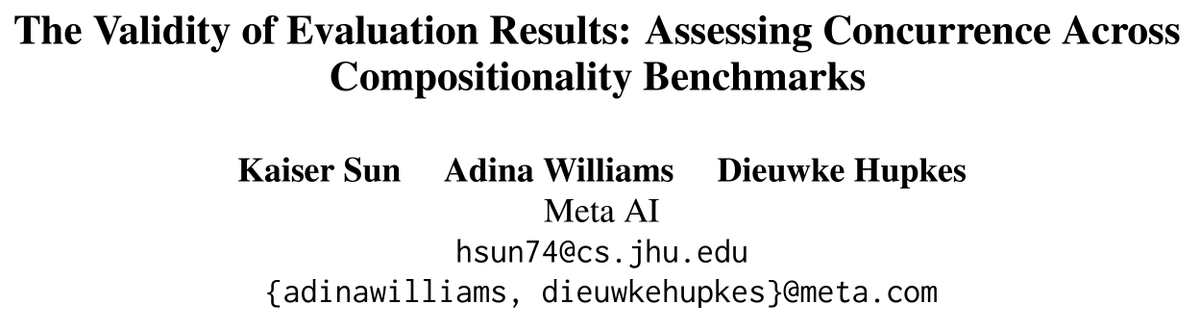
🔌Enhancing language models with retrieval boosts performance but demands more computes for encoding the retrieved documents. Do we need all the documents for the gains? We present 𝐑etrieve 𝐂𝐨𝐦press 𝐏repend (𝐑𝐄𝐂𝐎𝐌𝐏) arxiv.org/abs/2310.04408 (w/@WeijiaShi2, @eunsolc)
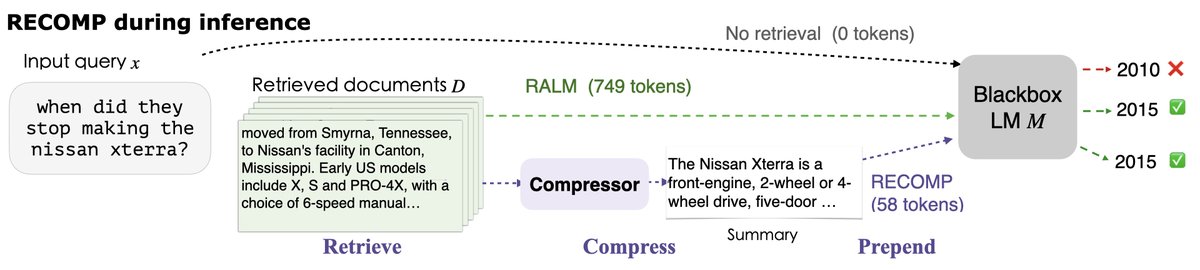
United States Trends
- 1. Chandler 60,8 B posts
- 2. #UFC309 173 B posts
- 3. Bo Nickal 7.876 posts
- 4. #MissUniverse 406 B posts
- 5. Do Bronx 6.901 posts
- 6. Tatum 25,9 B posts
- 7. Tennessee 53,3 B posts
- 8. Beck 21,1 B posts
- 9. Oregon 33,4 B posts
- 10. Paul Craig N/A
- 11. #GoDawgs 11 B posts
- 12. Georgia 96,7 B posts
- 13. Keith Peterson N/A
- 14. Nigeria 273 B posts
- 15. Locke 5.847 posts
- 16. Dinamarca 35 B posts
- 17. #discorddown 2.017 posts
- 18. Wisconsin 46,9 B posts
- 19. Mike Johnson 44,3 B posts
- 20. Dan Lanning 1.327 posts
Who to follow
-
Jie Huang
@jefffhj -
Weijia Shi
@WeijiaShi2 -
Zhaofeng Wu ✈️ EMNLP
@zhaofeng_wu -
Saadia Gabriel
@GabrielSaadia -
Shangbin Feng
@shangbinfeng -
Tao Yu
@taoyds -
Yizhong Wang
@yizhongwyz -
CLS
@ChengleiSi -
Ruiqi Zhong
@ZhongRuiqi -
Jiacheng Liu
@liujc1998 -
Alisa Liu
@alisawuffles -
Shiyue Zhang
@byryuer -
Yushi Hu
@huyushi98 -
Xiang Lisa Li
@XiangLisaLi2 -
Chenghao Yang
@chrome1996
Something went wrong.
Something went wrong.