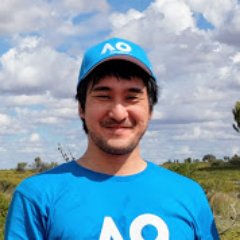
Naoto Usuyama
@naotousMicrosoft Health Futures @ Redmond 🧬| Biomedical NLP/Vision, Genomics | opinions are my own 🎾
Similar User

@sla

@toshi_k_datasci

@fukumimi014

@carolina_qwerty
@mhangyo

@Isa_rentacs

@hakobayato

@kyfwfw

@iBotamon

@kawatea03

@mohikashitarara

@hmikisato

@Prof_hrk

@yahagi1989

@Nobu575
正答率 98.2% OpenAI の o1-preview が医師国家試験で正答率 98.2% を記録しました 日本語の問題を翻訳せず、そのまま読ませた結果です knowledge cutoff date の後に行われた今年の試験問題でもこの性能です ぜひ論文をチェックしてください: arxiv.org/pdf/2411.03590
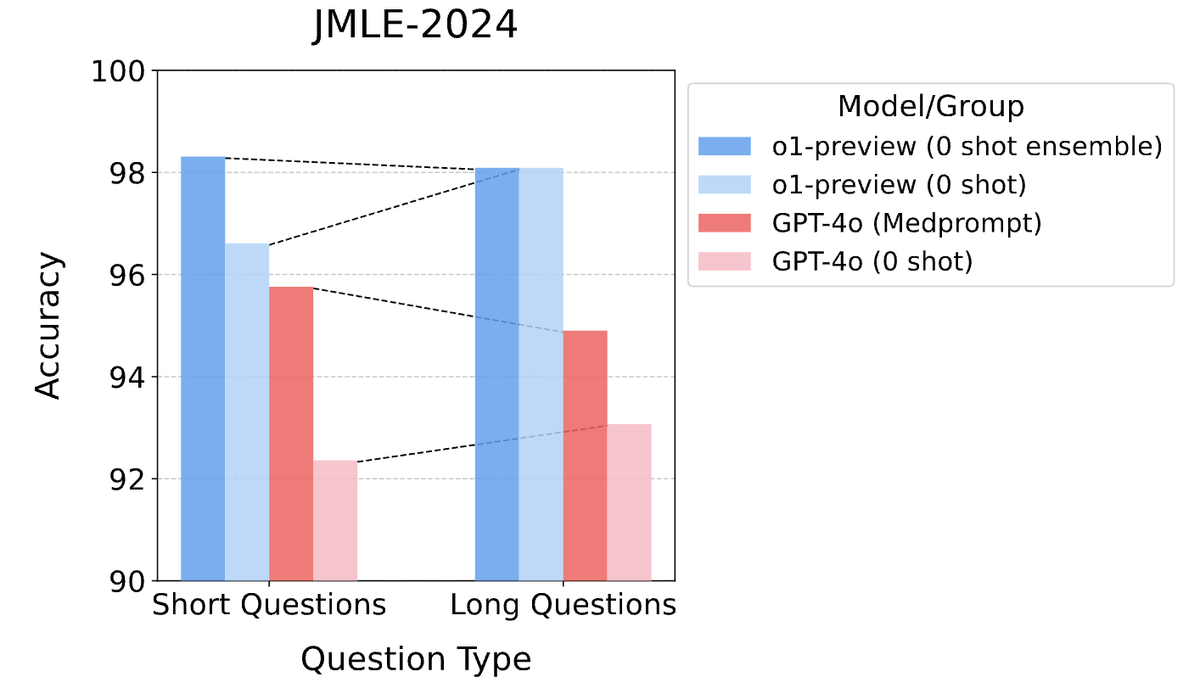
Breaking medical AI records🚀 OpenAI’s o1-preview scores 96% on MedQA and sets new SOTA across multiple benchmarks⚡ Read more in our paper: arxiv.org/pdf/2411.03590
Here's a comparative analyses of the performance of different models on the MedQA benchmark, showing the top performance of o1 preview, beating our prior SOTA report on the power of Medprompt with GPT-4.
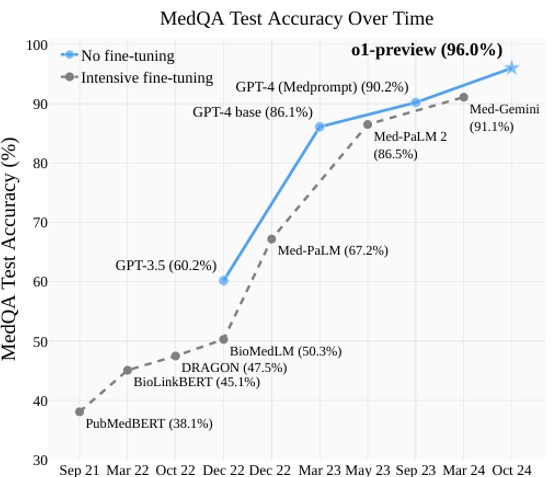
A couple of findings🍓that might interest you: 1. zero-shot outperforms few-shot, CoT, etc. 2. ensemble still works but can be pricey! 3. gpt-4o w. medprompt already does a good job if your budget is limited. 4. control o1's thinking time by prompting. Find more in the paper!
Cost-benefit tradeoffs: We explore the Pareto frontier as a function of compute costs, considering accuracy vs total API cost (log scale) on MedQA, comparing o1-preview (Sep 2024), GPT-4o (Aug 2024), GPT-4 Turbo (Nov 2023) with various run-time steering strategies.
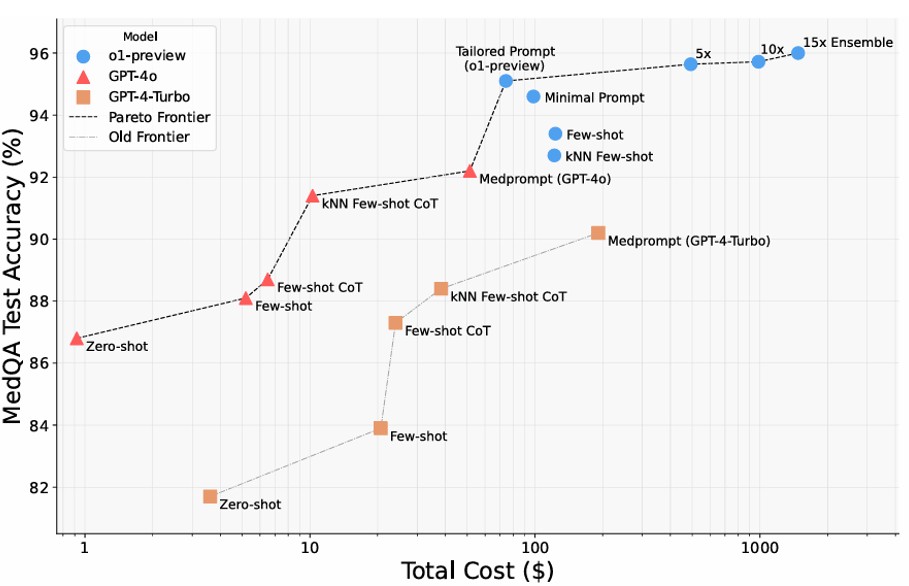
Internship applications are live for next summer! 🏞️
Interested in real-world evidence (RWE), LLMs, multimodal models, and research at the intersection of ML and Health? Join us next summer in advancing health at the speed of AI @MSFTResearch Health Futures: jobs.careers.microsoft.com/global/en/job/…
Analysis of o1-preview on medical benchmarks, comparing o1's native inference-time powers to prior work on Medprompt, a different approach to inference-time analysis. Assessment: o1 reaches new heights--and shows great promise for medical applications. tinyurl.com/9yspcpm6
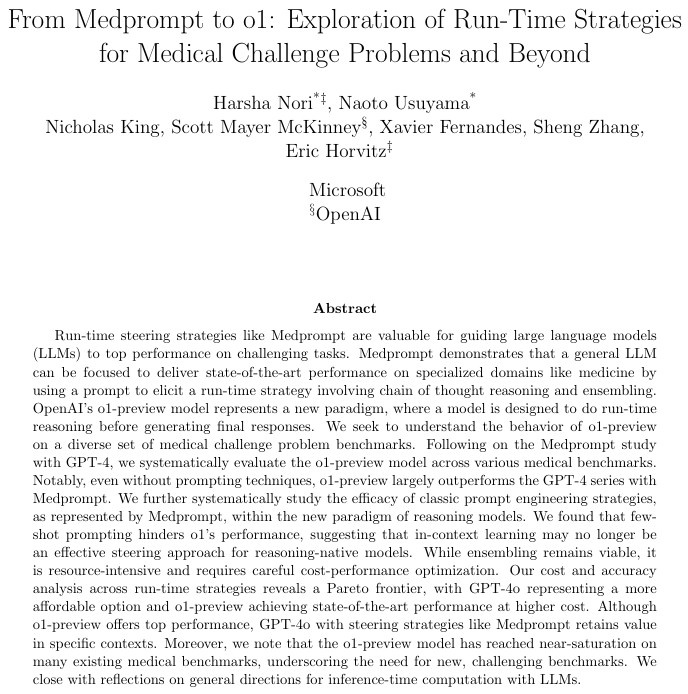
@elonmusk this tends to be a much more efficient approach to bringing medical imaging competencies into general reasoners - we have released some open source medical imaging embedding models that you and the team can explore with Grok microsoft.com/en-us/industry…
日本マイクロソフト公式サイトでGigaPathプロジェクトが紹介されました! コード: github.com/prov-gigapath/… モデル: huggingface.co/prov-gigapath/… 論文: nature.com/articles/s4158… 今後もさらにコードやモデルをリリース予定です。…
【 GigaPath:デジタル病理学のための基盤モデル】 デジタル病理のための新しいビジョン トランスフォーマー「GigaPath」をご紹介します。 マイクロソフトが協力する、個別化医療マルチモーダル生成 AI の実現に向けた「GigaPath」プロジェクトの詳細をご覧ください。 msft.it/6014mK3Pw
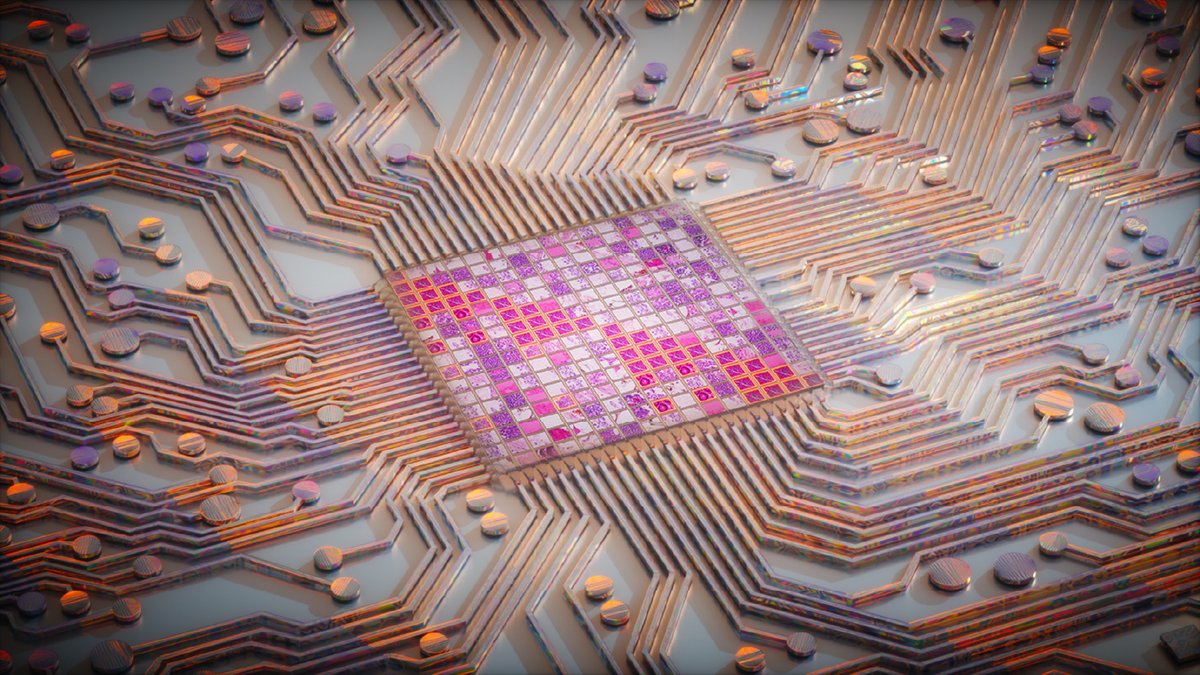
中学からの親友と共著で論文出せる日が来るとは...! マルチモーダルなオミクスデータをCNNで解析する、かなり先進的なプロジェクトです。 お手伝いさせて頂いてありがとうございます! @MichiKyo2 @hellofuture100 @KHasegawa
新たな論文をnpj Systems Biology and Applicationsから出版しました。 この研究では、複数のオミクス情報を画像化してAIモデルを構築し、重要なオミクスネットワークを抽出することで細気管支炎の病態解明と治療Target同定に繋げました。Microsoftの@naotous との共著です! nature.com/articles/s4154…
We might be at the pivotal moment for AI in biomedical imaging! Excited to share our work at @CVPR! GigaPath: nature.com/articles/s4158… @Nature BiomedParse: microsoft.github.io/BiomedParse/
I think we may be approaching a tipping point in AI for medical imaging. Incredible progress at the @CVPR workshop on foundation models for medical imaging. Our teams were pleased to share work @MSFTResearch on #Biomeparse and #GigaPath to standing-room only audience.
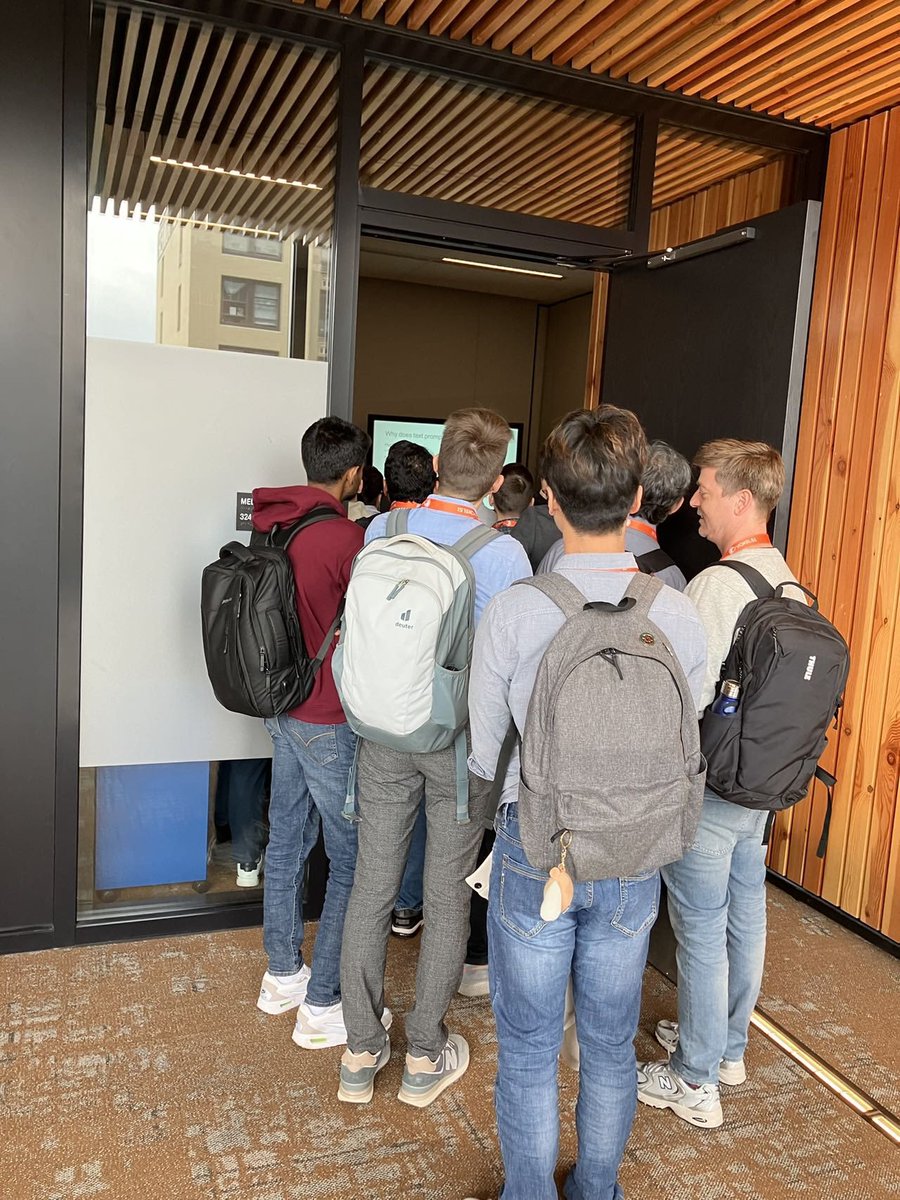
I’m thrilled to share that our GigaPath paper has been published in Nature! This is a crucial step towards precision medicine. Our AI model, trained on over 1 billion pathology images from Providence, learns the “deep representations” of cancer histopathology. The representations…
Just out @Nature The 1st whole-slide digital pathology #AI foundation model pre-trained on large-scale real-world data, from over 1.3 billion images, 30,000 patients nature.com/articles/s4158… @hoifungpoon @HanwenXu6 @Microsoft @UW @naotous @MSFTResearch
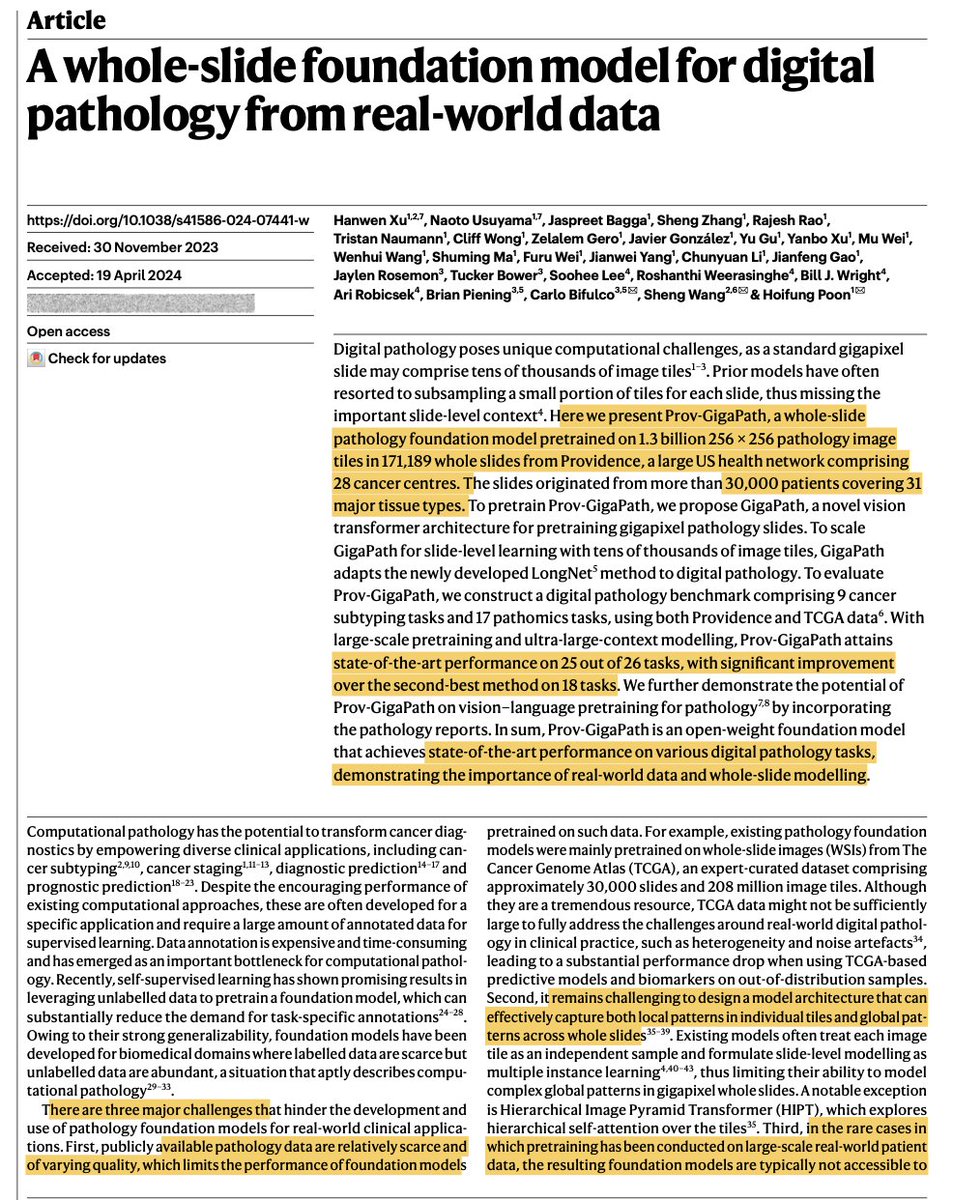
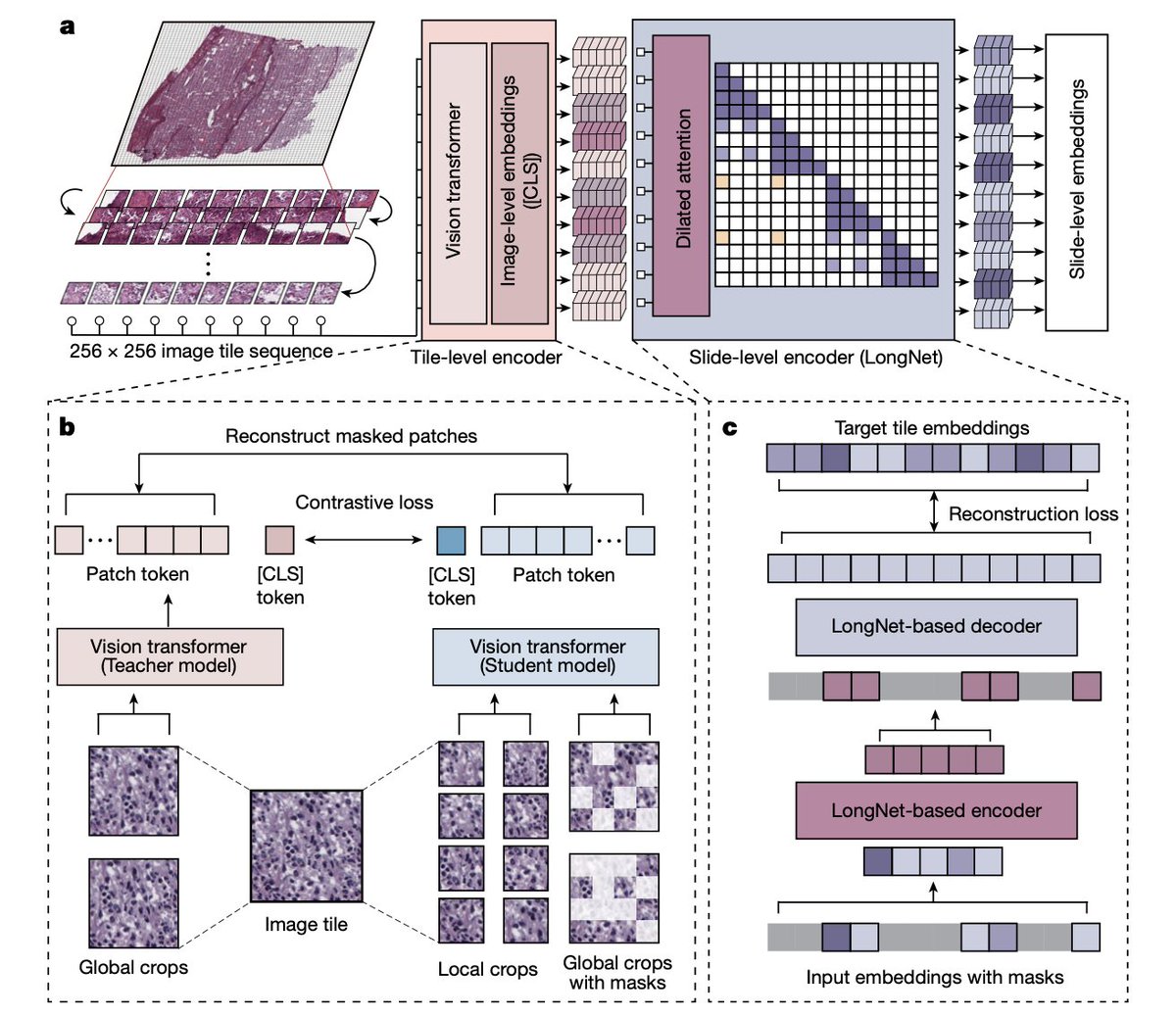
🌟 Prev-GigaPath is out @Nature Witness the success of image self-supervised learning in healthcare to tackle unique challenges amidst language-image training dominance? Kudos to visionaries @HanwenXu6 @naotous for leading this remarkable effort! 👏🔥
Just out @Nature The 1st whole-slide digital pathology #AI foundation model pre-trained on large-scale real-world data, from over 1.3 billion images, 30,000 patients nature.com/articles/s4158… @hoifungpoon @HanwenXu6 @Microsoft @UW @naotous @MSFTResearch
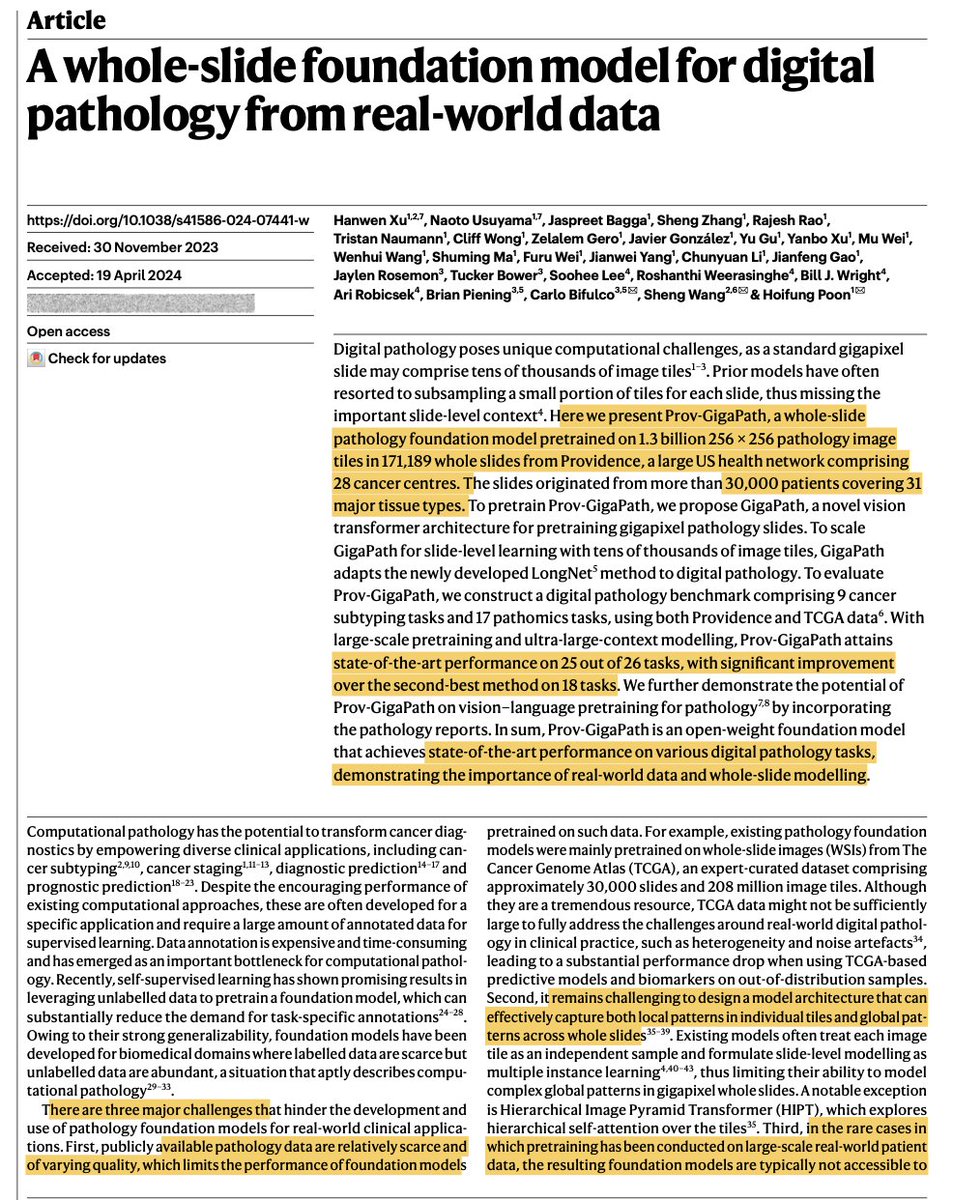
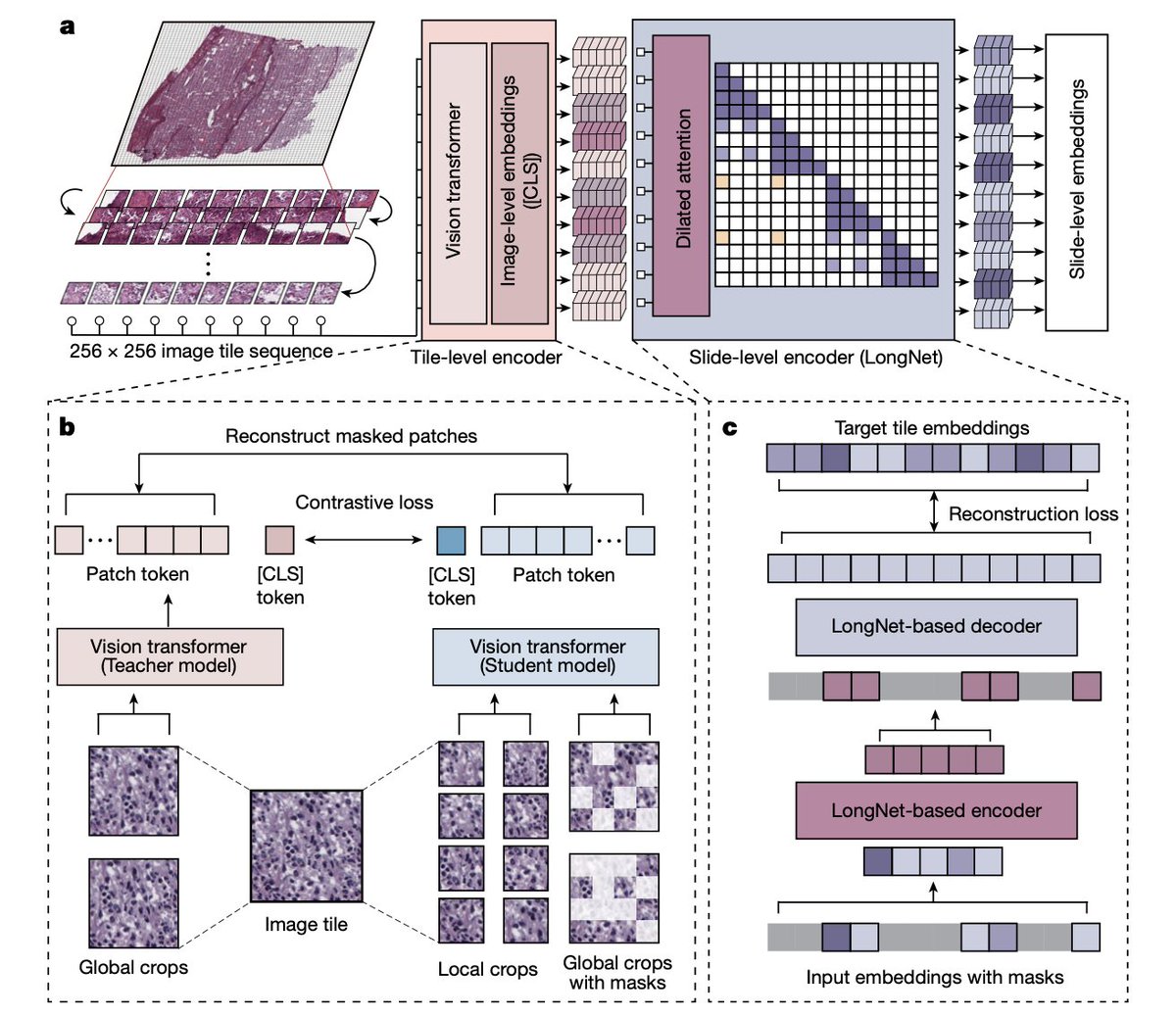
BiomedParse: a biomedical foundation model for image parsing of everything everywhere all at once abs: arxiv.org/abs/2405.12971 project page: microsoft.github.io/BiomedParse/ Microsoft Research introduce BiomedParse, a biomedical foundation model for imaging parsing that can jointly…
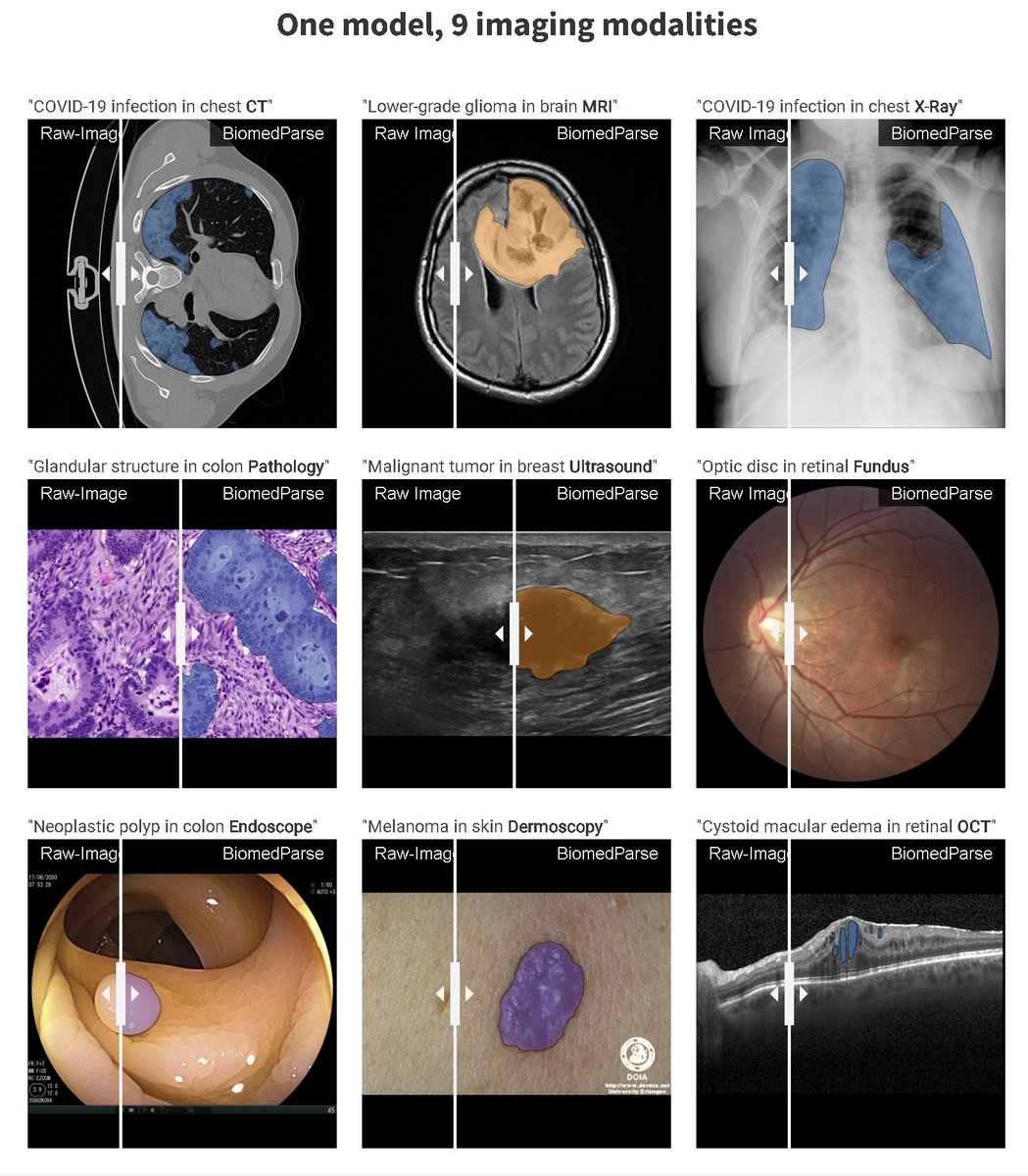
Just out @Nature: Whole-slide foundation model for pathology. Gigapath trained on 170K whole slides, >1billion image tiles. Scale delivers! SOTA reached on cancer subtyping, gene mutation prediction, language tasks aka.ms/gigapath @MSFTResearch @WHOSTP @hoifungpoon
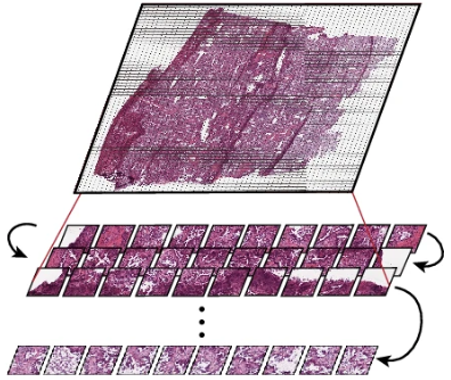
We've made a ton of progress on medical imaging with AI. What is most important here is the possibility that there is strong possibility of useful scaling at play.
Just out @Nature The 1st whole-slide digital pathology #AI foundation model pre-trained on large-scale real-world data, from over 1.3 billion images, 30,000 patients nature.com/articles/s4158… @hoifungpoon @HanwenXu6 @Microsoft @UW @naotous @MSFTResearch
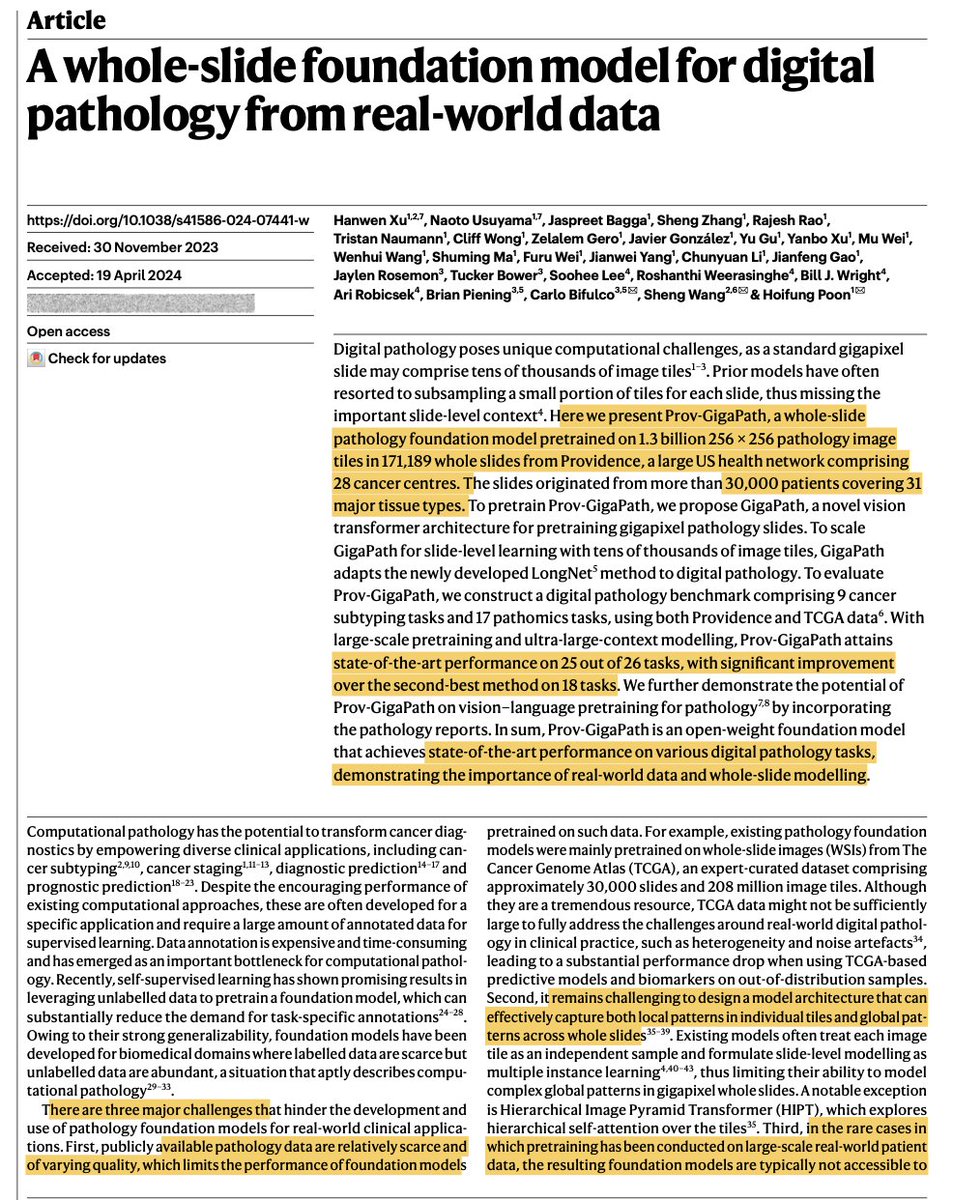
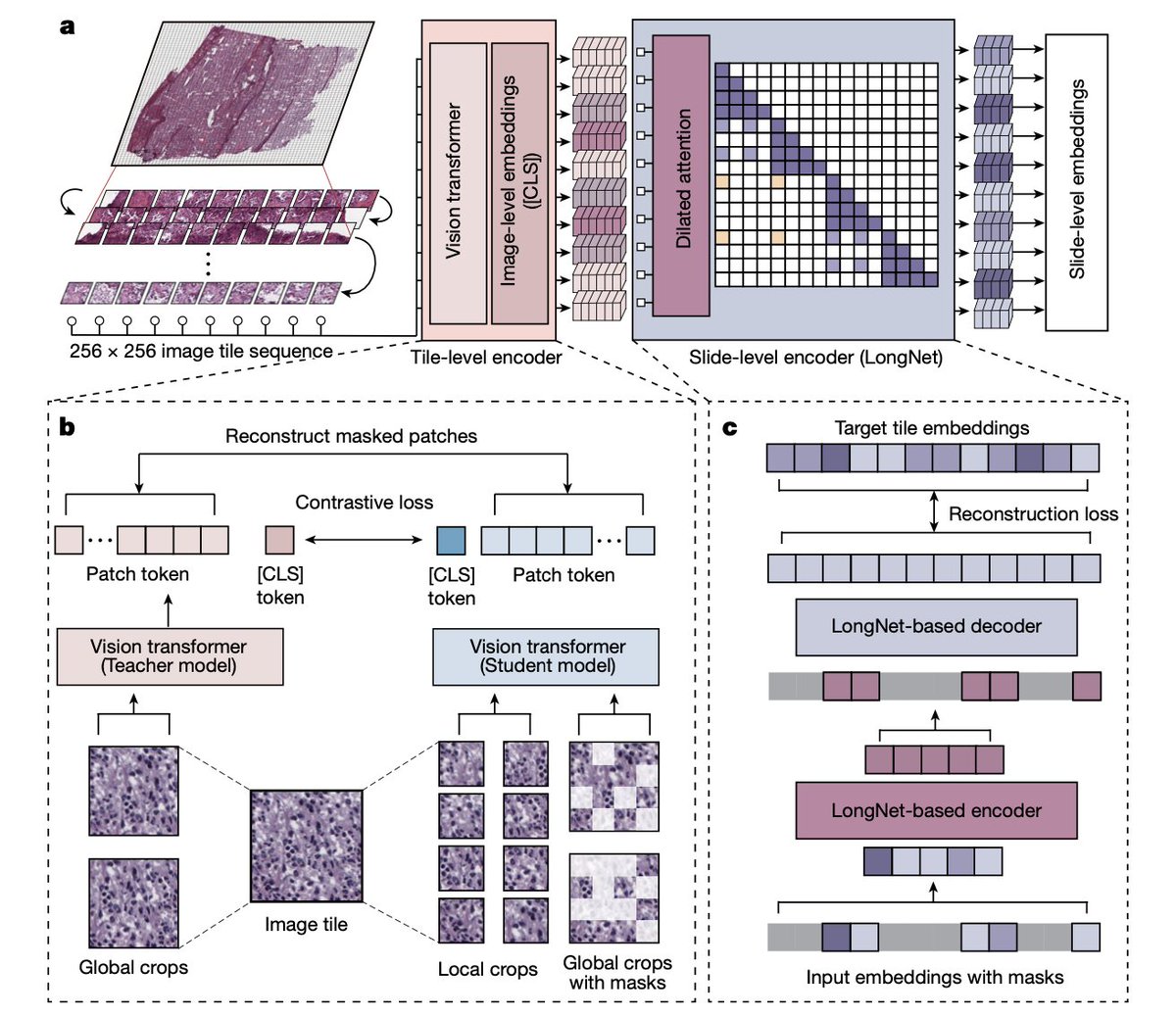
Just out @Nature The 1st whole-slide digital pathology #AI foundation model pre-trained on large-scale real-world data, from over 1.3 billion images, 30,000 patients nature.com/articles/s4158… @hoifungpoon @HanwenXu6 @Microsoft @UW @naotous @MSFTResearch
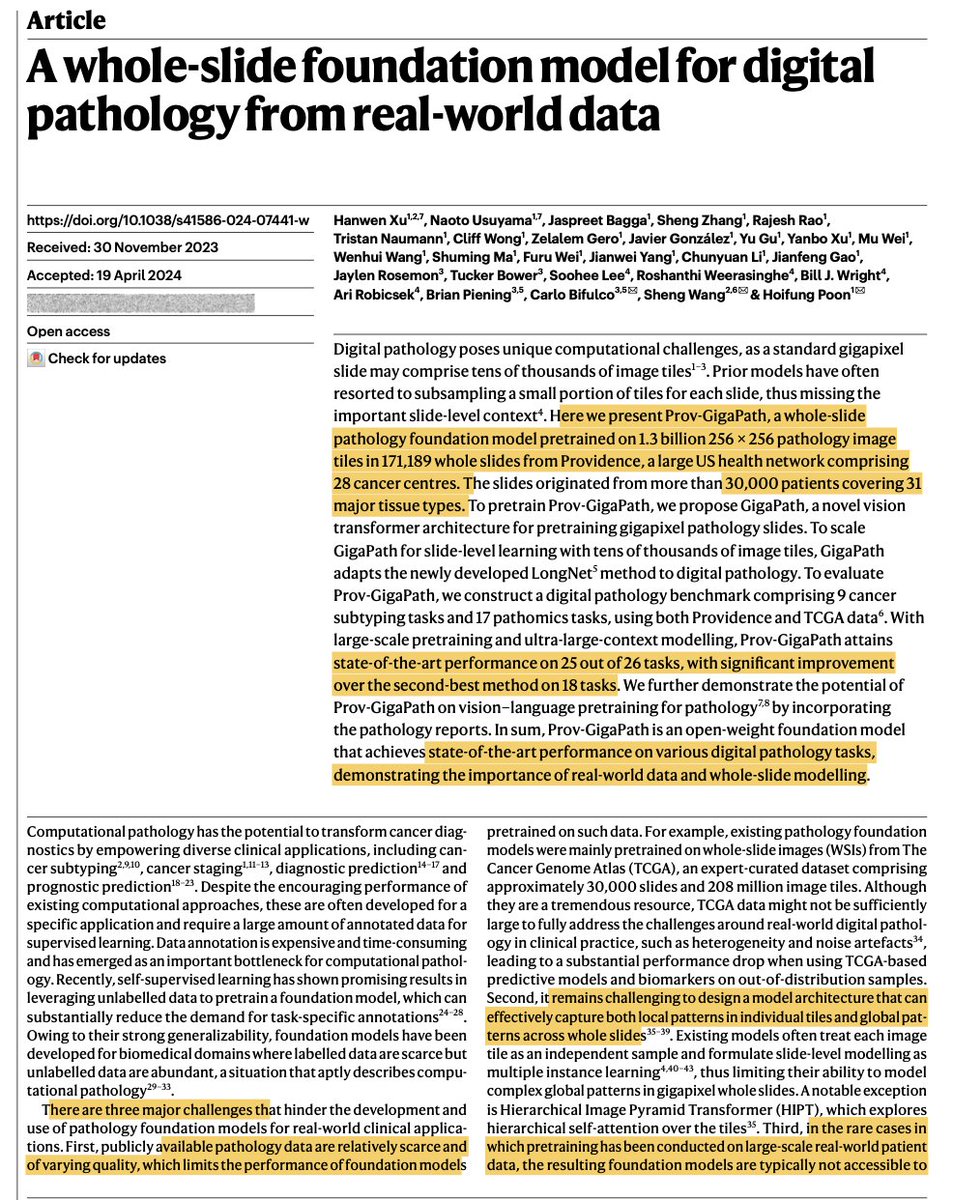
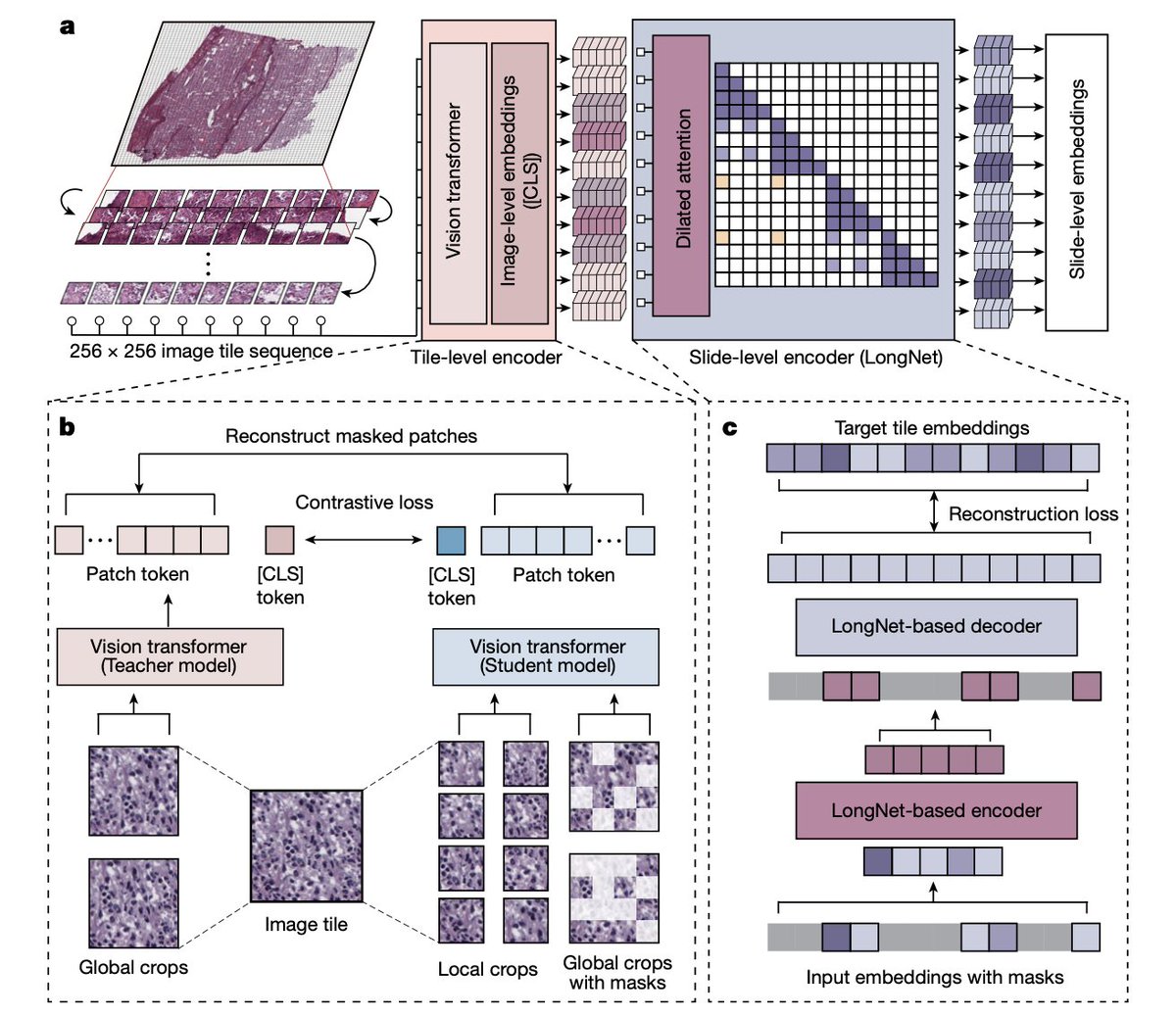
Proud to see our GigaPath Foundation Model project in the spotlight at Microsoft Research✨
Naoto Usuyama, Principal Researcher, Health Futures, proposes GigaPath—a novel approach for training large ViT for gigapixel pathology images, using a diverse real-world cancer patient dataset, with the goal of laying a foundation for cancer pathology AI. msft.it/6010cmXCY
Excited to share 🛤️ RaLEs: a Benchmark for Radiology Language Evaluations at #NeurIPS2023! We introduce a new benchmark to evaluate radiology language understanding and generation, addressing unique challenges in this domain. 🧵 1/n

With Medprompt+, GPT-4 achieves 90.1% on MMLU, outperforming Gemini Ultra and human experts. Code: github.com/microsoft/prom… Medprompt paper: arxiv.org/pdf/2311.16452…
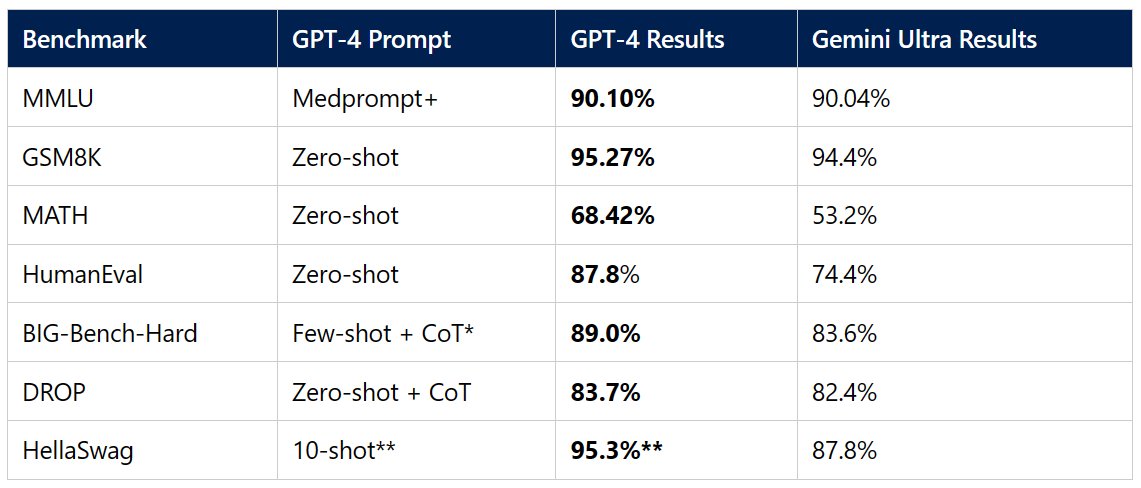
Today, we're sharing several updates on methods for steering foundation models aka.ms/AAo3s1z, including studies that build on our earlier #Medprompt work and a new resource on github with scripts & info #promptbase @MSFTResearch
LLaVA-Med is open-source now 🌋🔥
LLaVA-Med is finally fully open-sourced after 5-month intensive discussions to go through Microsoft release process. Thank all who have helped to make it happen and thank the community for the patience. github.com/microsoft/LLaV…
United States Trends
- 1. Good Sunday 58,8 B posts
- 2. #AskFFT N/A
- 3. Mike Johnson 50,9 B posts
- 4. #sundayvibes 6.984 posts
- 5. #ATEEZ_1stDAESANG 10,2 B posts
- 6. #AskZB N/A
- 7. Jon Jones 267 B posts
- 8. CONGRATULATIONS ATEEZ 19,3 B posts
- 9. #UFC309 347 B posts
- 10. MY ATEEZ 73,5 B posts
- 11. Blessed Sunday 18,6 B posts
- 12. Jones 446 B posts
- 13. Alec Baldwin 11,1 B posts
- 14. Jussie 4.415 posts
- 15. Aspinall 29,6 B posts
- 16. Yosohn N/A
- 17. Chandler 93,1 B posts
- 18. Lord's Day 1.724 posts
- 19. Mickey D N/A
- 20. Jelly Roll 11,3 B posts
Who to follow
-
比戸
@sla -
toshi_k
@toshi_k_datasci -
福田敦史 / Aillis CTO
@fukumimi014 -
Carolina
@carolina_qwerty -
HANGYO, Masatsugu
@mhangyo -
J. Kuroda
@Isa_rentacs -
Hayato Kobayashi
@hakobayato -
小山雄太郎🧠🇺🇸
@kyfwfw -
Yuta Nakamura🐠
@iBotamon -
かわてぃー
@kawatea03 -
mohi
@mohikashitarara -
三木里秀予@おいしいものを食べる
@hmikisato -
hrk先生
@Prof_hrk -
やはぎ@ココグルメ取締役COO・共同創業者
@yahagi1989 -
Nobu
@Nobu575
Something went wrong.
Something went wrong.