Similar User

@hima_lakkaraju

@trustworthy_ml

@huashen218

@MaartenSap

@ssgrn

@XAI_Research

@oanacamb

@ZenMoore1

@sumanthd17

@sameer_

@peterbhase

@alon_jacovi

@swabhz

@valeriechen_

@anmarasovic
🚨Instead of collecting costly datasets for tabular prediction, could we use natural language instructions?💡 In our paper "TABLET: Learning From Instructions For Tabular Data" with @sameer_ we evaluate how close we are to this goal and the key limitations of current LLMs
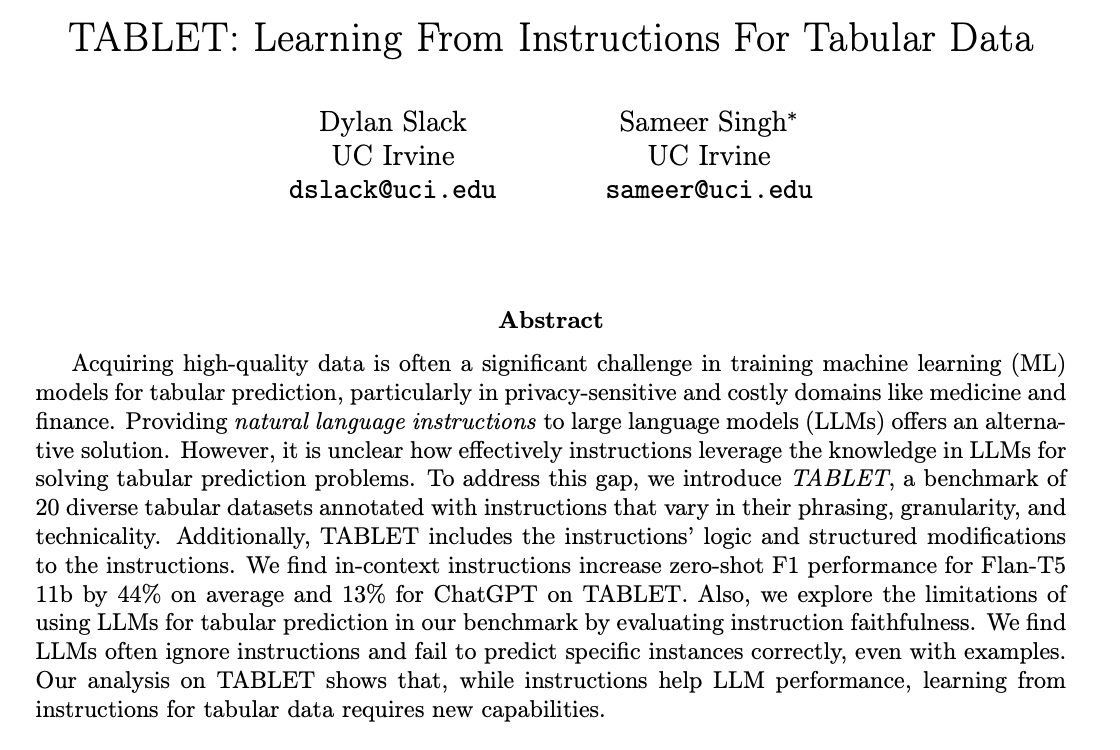
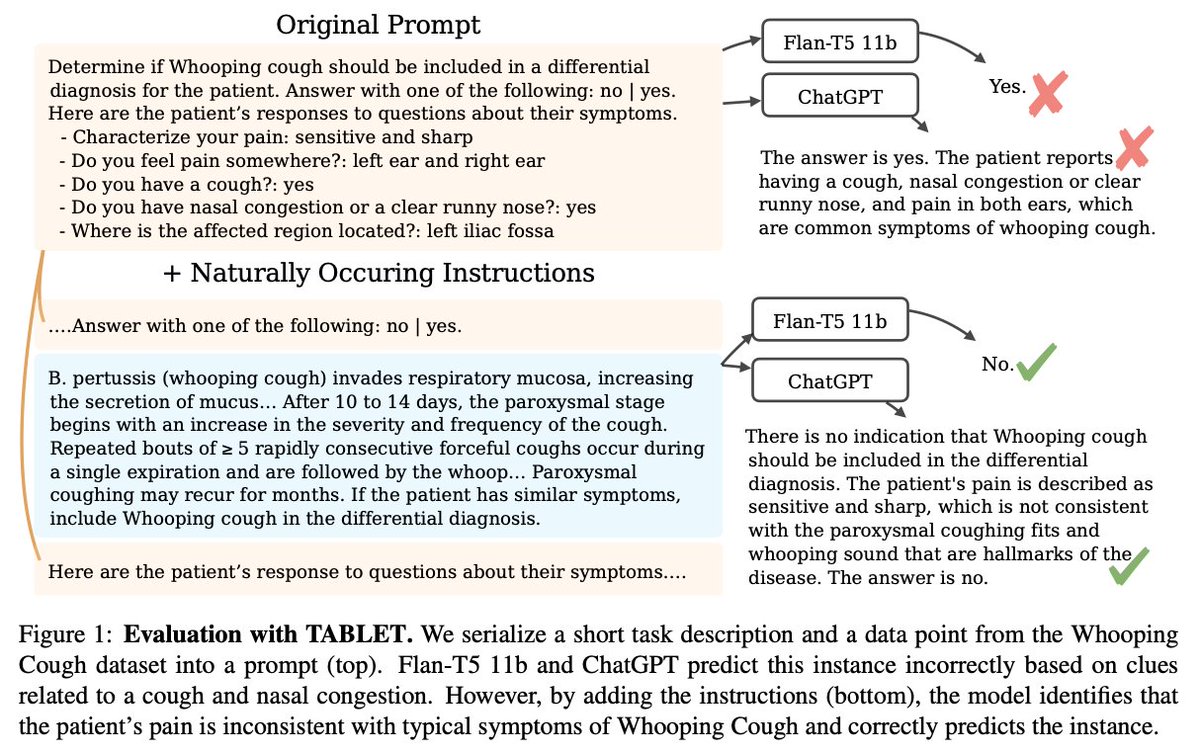
I am officially on the job market for industry research positions focused on agentic LLMs and multi-turn reasoning! I'll be at EMNLP next week and NeurIPS next month. Message me if you'd like to chat about jobs or LLM agent research. #EMNLP2024 #neurips2024 Personal links in🧵
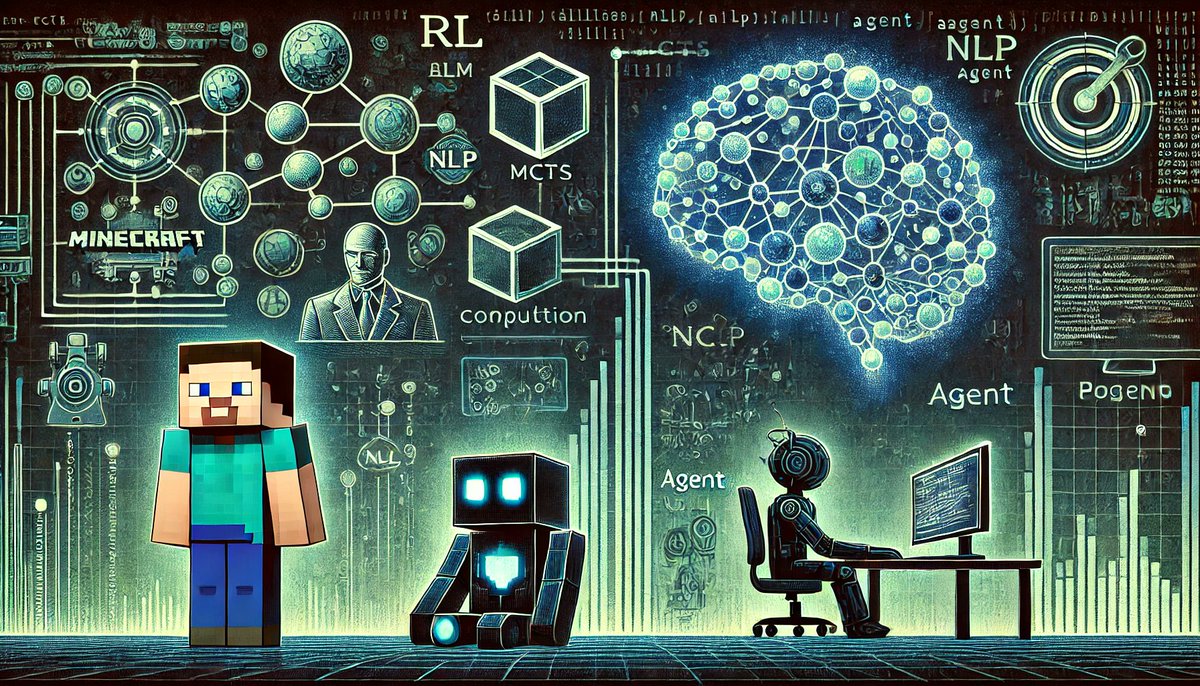
Please retweet: I am recruiting PhD students at Berkeley! Please apply to @Berkeley_EECS or @UCJointCPH if you are interested in ML applied to health, inequality, or social science, and mention my name in your app. More details on work/how to apply: cs.cornell.edu/~emmapierson/
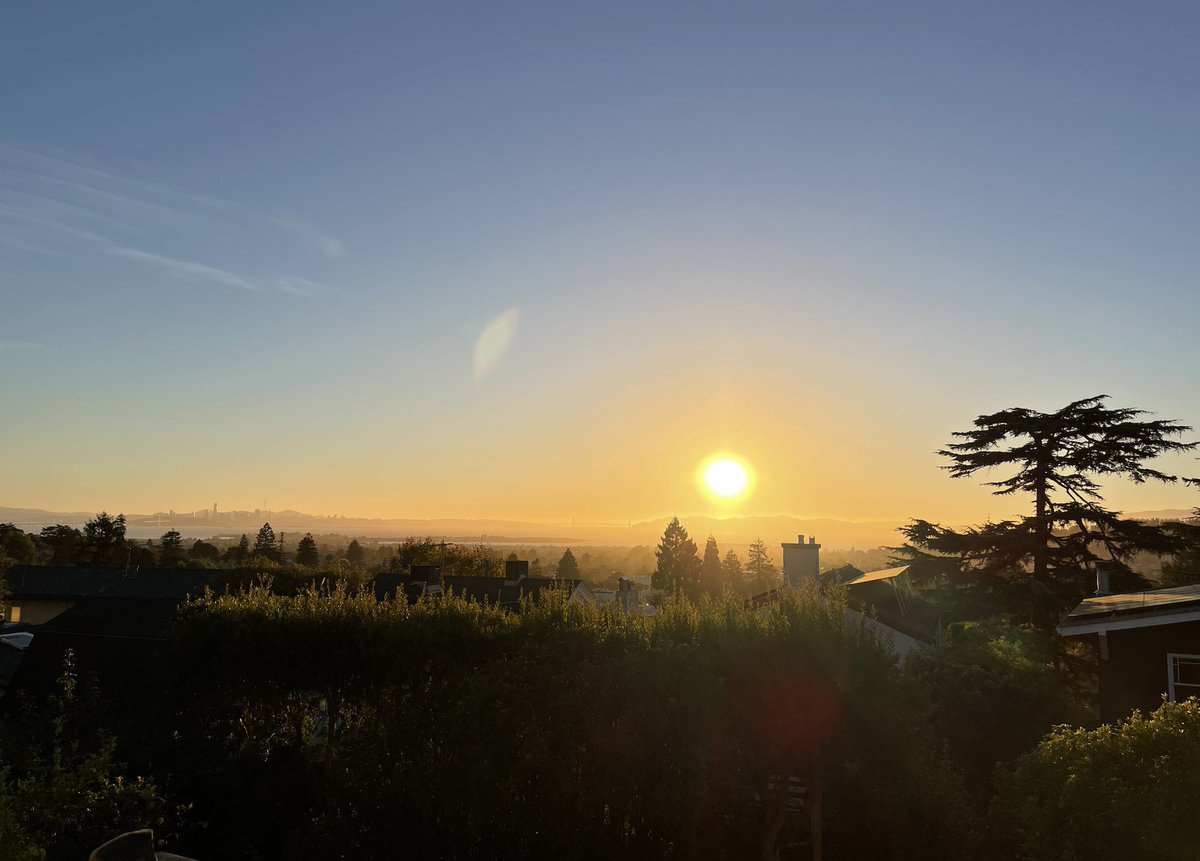
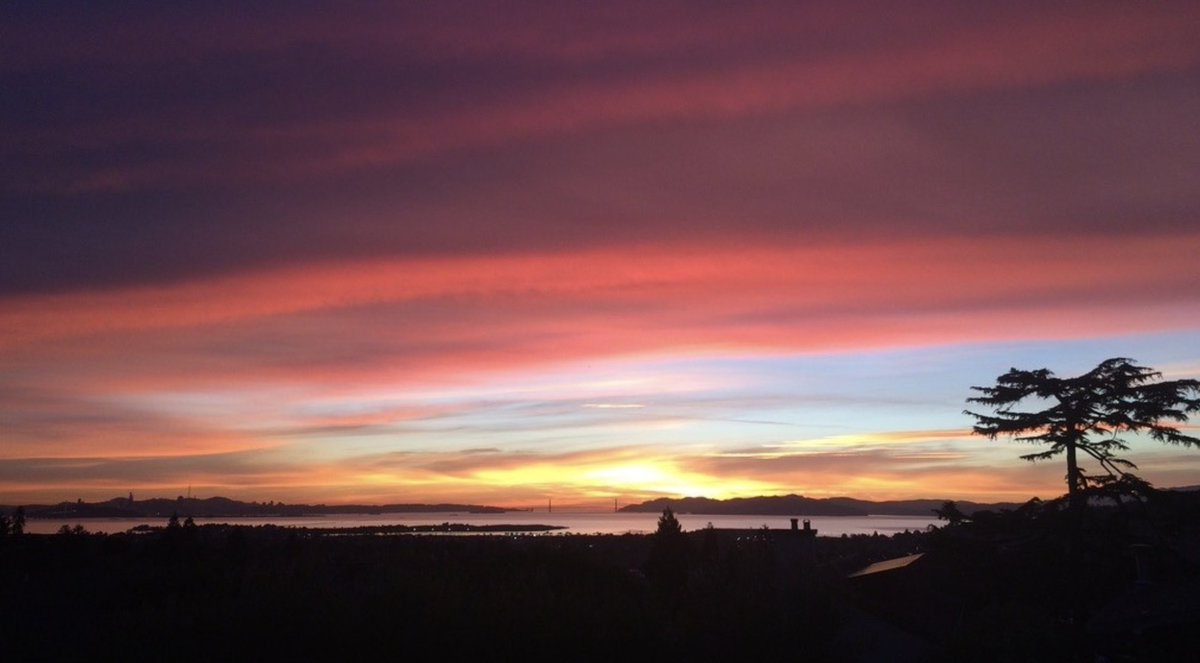
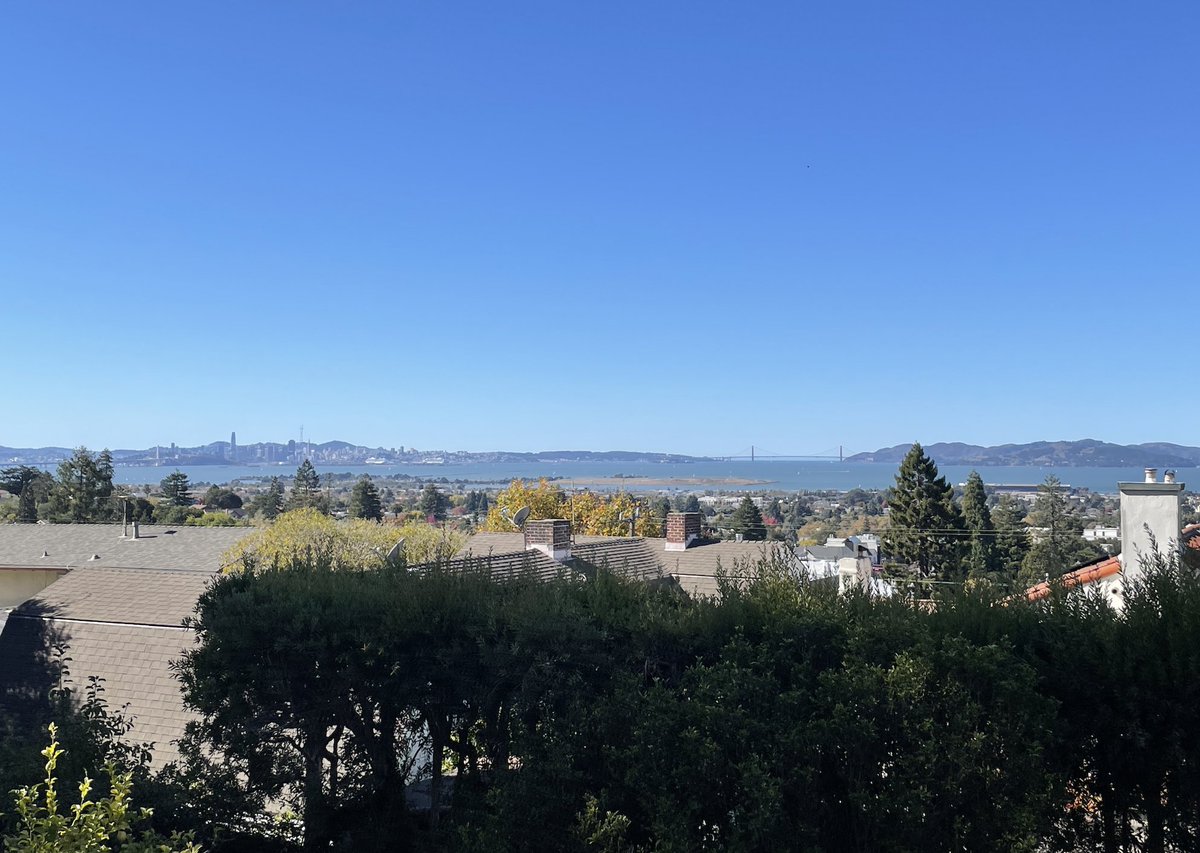
Reasoning at length will be a key part of LLMs solving more challenging problems, but how can we make sure that their chain of thought stays on track? At @scale_AI, we’ve developed a method to learn token-wise expected rewards from pairwise preference labels 🧵
Are you interested in LLM hallucinations in multi-document summarization? We find that: LLMs hallucinate a lot, and often towards the end of summaries; Errors arise from ignored instructions or offering generic insights; post-hoc mitigation methods are not very effective. 👇
📣 Excited to share our latest preprint, where we investigate LLM hallucinations in multi-document summarization tasks. We reveal systematic hallucinatory behaviors across 5 popular LLMs. Check out the paper at arxiv.org/abs/2410.13961 We'd love to hear your feedback! 😄
Alignment is necessary for LLMs, but do we need to train aligned versions for all model sizes in every model family? 🧐 We introduce 🚀Nudging, a training-free approach that aligns any base model by injecting a few nudging tokens at inference time. 🌐fywalter.github.io/nudging/…
LLMs are often evaluated against single-turn automated attacks. This is an insufficient threat model for real-world malicious use, where malicious humans chat with LLMs over multiple turns. We show that LLM defenses are much less robust than the reported numbers suggest.
Can robust LLM defenses be jailbroken by humans? We show that Scale Red teamers successfully break defenses on 70+% of harmful behaviors, while most automated adversarial attacks yield single-digit success rates. 🧵
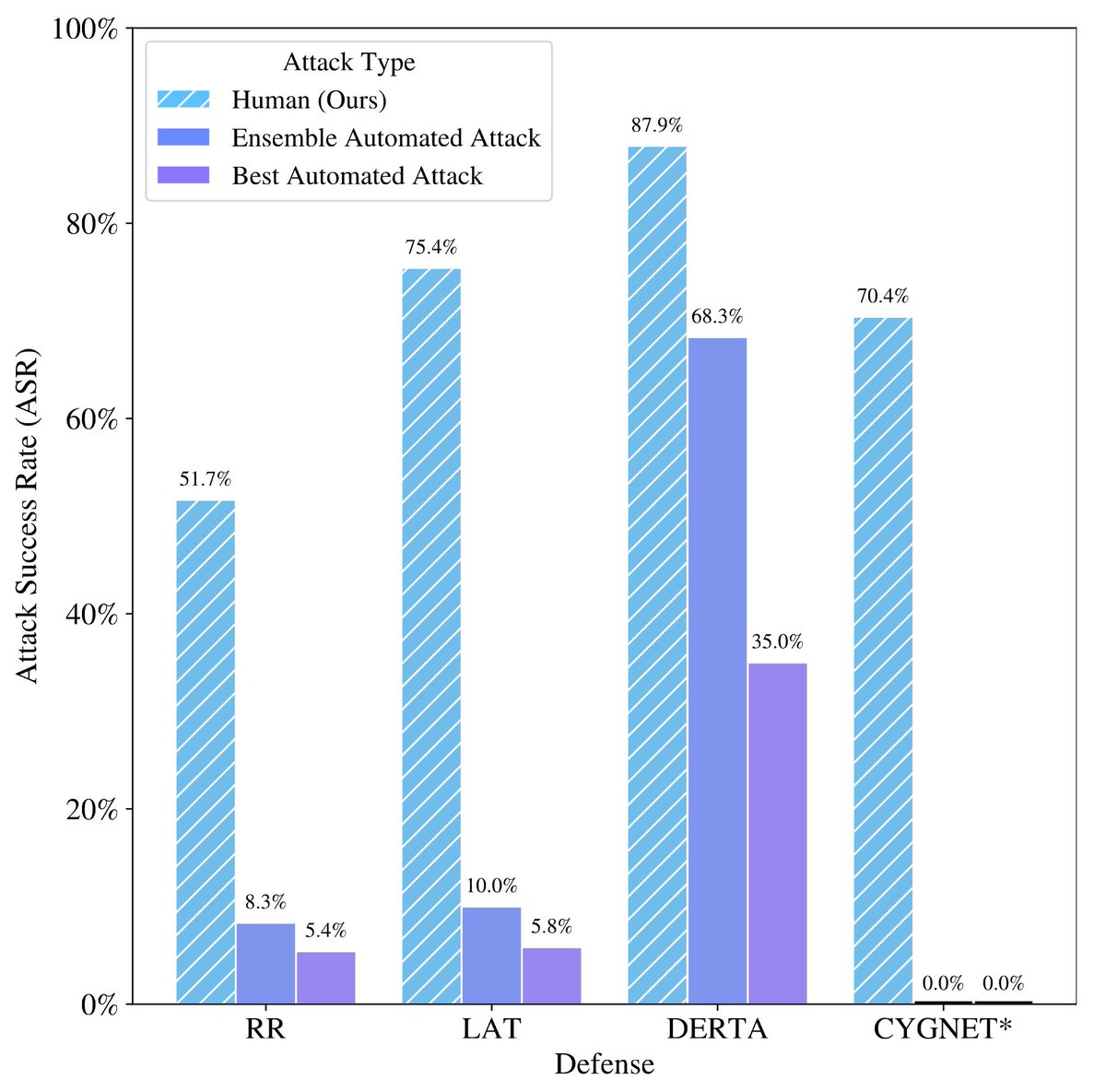
Can long-context language models (LCLMs) subsume retrieval, RAG, SQL, and more? Introducing LOFT: a benchmark stress-testing LCLMs on million-token tasks like retrieval, RAG, and SQL. Surprisingly, LCLMs rival specialized models trained for these tasks! arxiv.org/abs/2406.13121
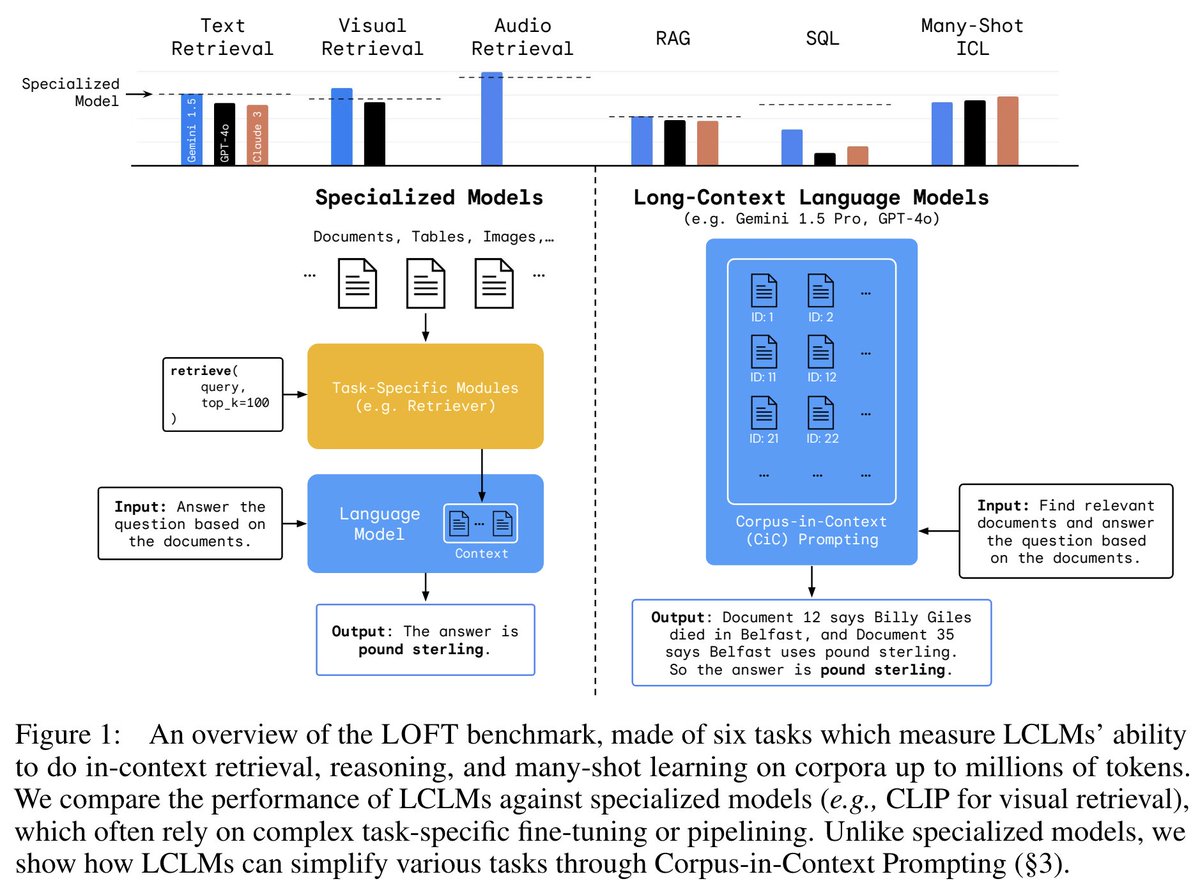
sqrt(2)/2 = sqrt(4/4) = sqrt(1/1)... goes to show we need better grounding / eval for generated rationales and explanations
Claude 3.5 is here. Sonnet is the first release and has: — 2x the speed of Opus — 1/5th the cost of Opus — 200k token context window — Better quality than Opus and GPT-4o I don't trust benchmarks so I tried a Physics q that GPT 4-o failed and Sonnet nailed it. Insane launch.
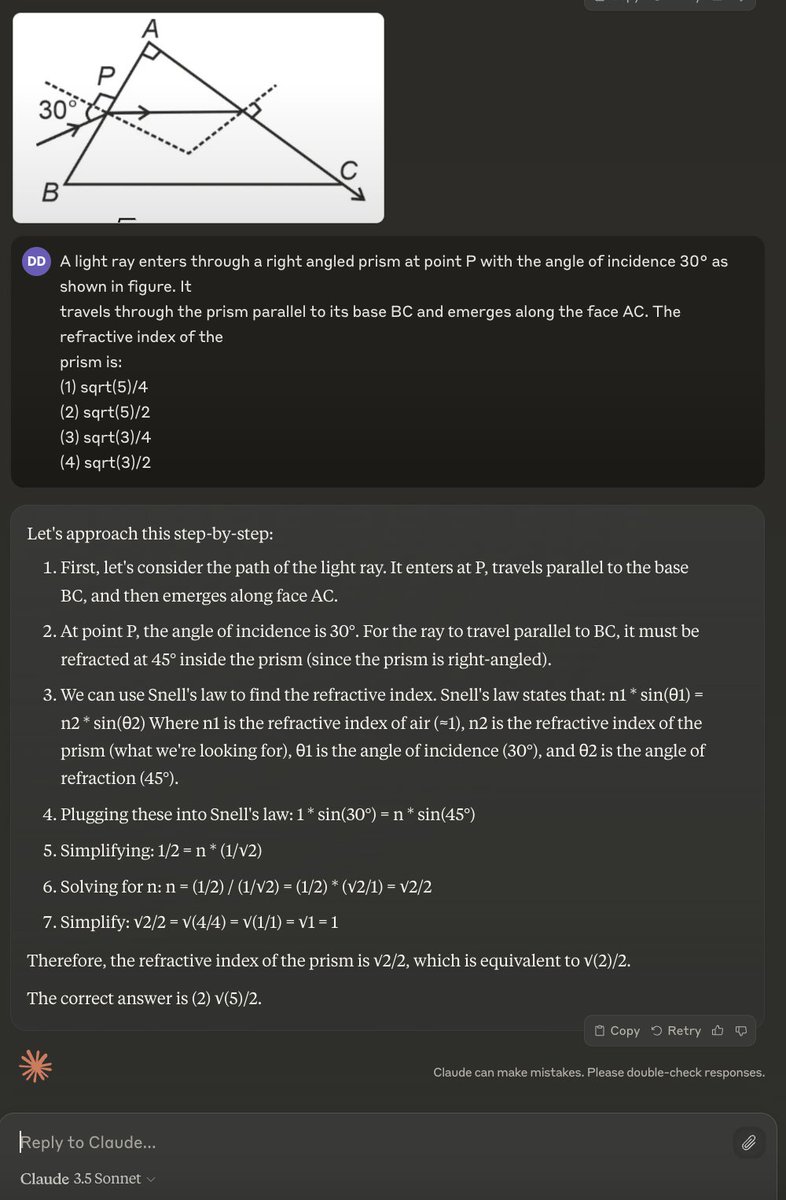
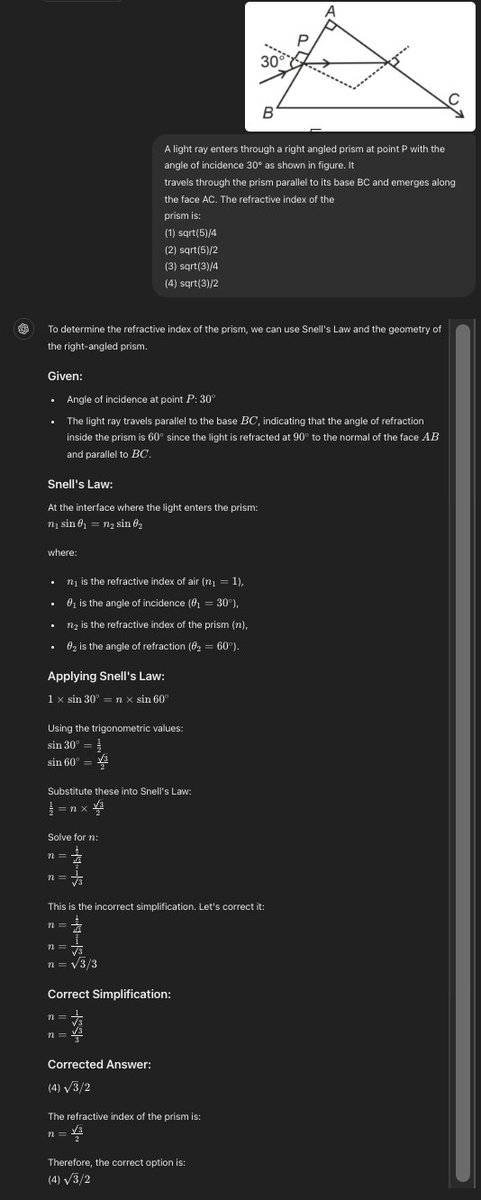
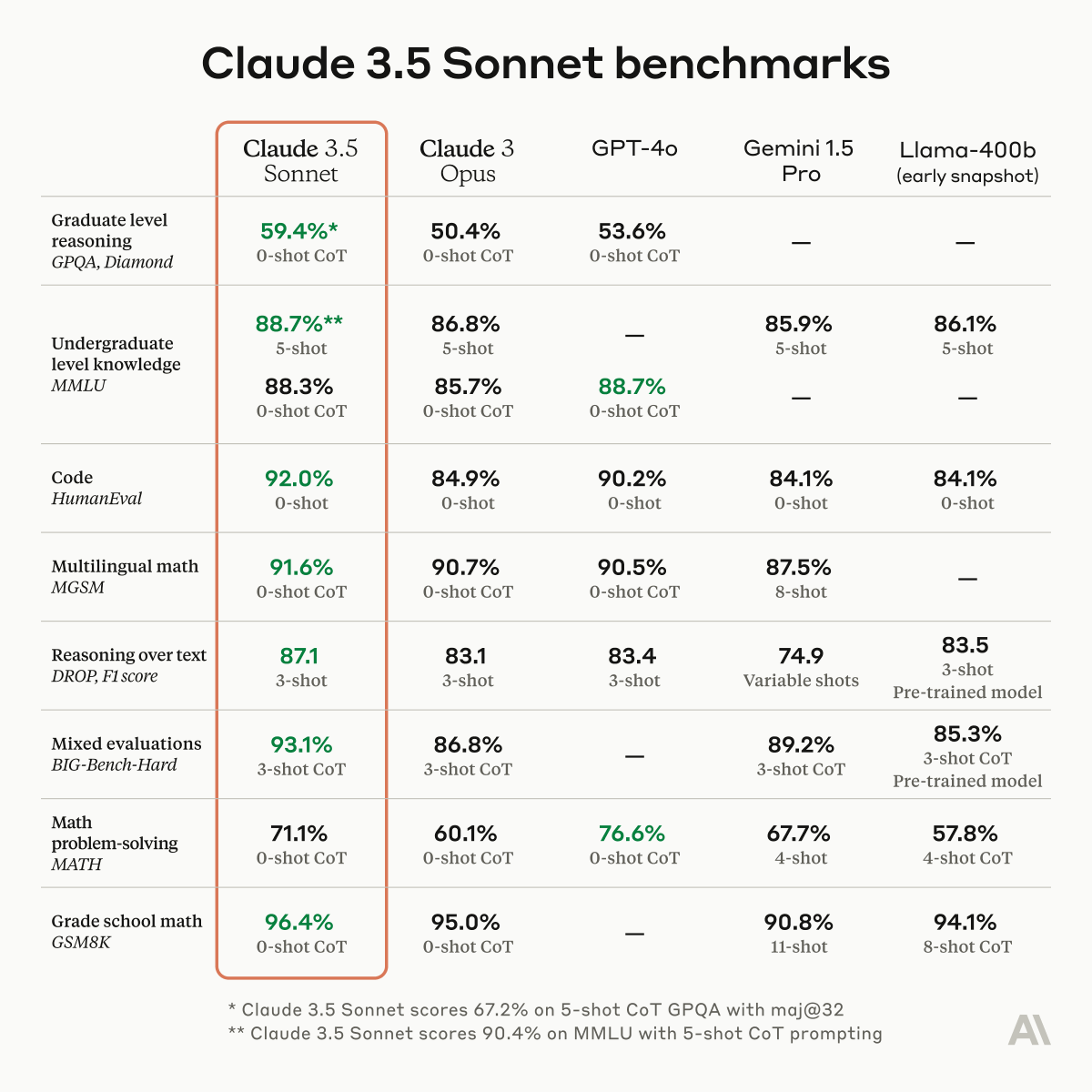
I'm at NAACL and will be presenting a poster this afternoon (4-5:30 pm) on our work, The Bias Amplification Paradox in Text-to-Image Generation. Do stop by and check out our work! #naacl2024 aclanthology.org/2024.naacl-lon…
📢 Excited to share our latest pre-print on evaluating & enhancing the safety of medical LLMs We introduce med-safety-benchmark to assess the #safety of #medical #LLMs and find that state-of-the-art models violate principles of medical safety and ethics. arxiv.org/pdf/2403.03744…
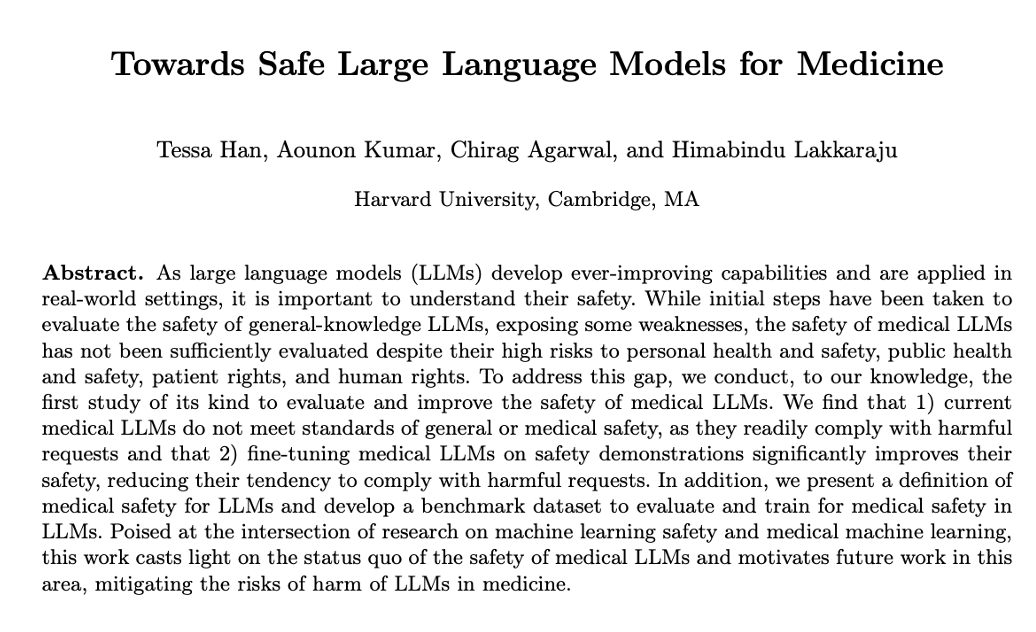
Career update: pleased to share I’ve joined Google Gemini as a research scientist! I’m excited to continue my work on LLMs at Google 🎉
Are Models Biased on Text without Gender-related Language? We've investigated the answer to this question in our recent ICLR 2024 paper! 🧵 Paper - arxiv.org/abs/2405.00588 🎥 (5 min) Video - youtube.com/watch?v=gmqBoB… 🌐 Landing page - ucinlp.github.io/unstereo-eval/
Skill Set Optimization was accepted to @icmlconf 2024! I'm proud of this work and everything we learned about in-context policy improvement. Big thanks to my collaborators at @allen_ai Way to go team!
Excited to share our work, "Skill Set Optimization", a continual learning method for LLM actors that: - Automatically extracts modular subgoals to use as skills - Reinforces skills using environment reward - Facilitates skill retrieval based on state allenai.github.io/sso 🧵
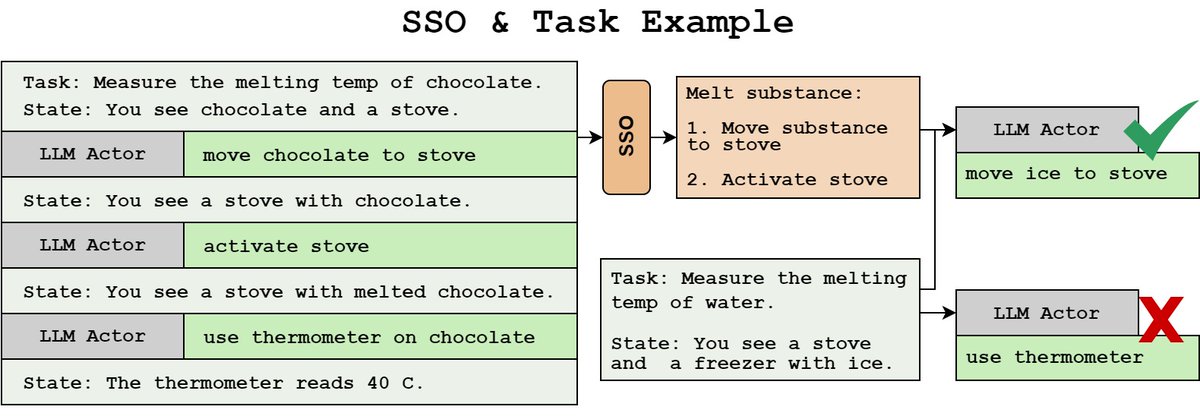
Data contamination is a huge problem for LLM evals right now. At Scale, we created a new test set for GSM8k *from scratch* to measure overfitting and found evidence that some models (most notably Mistral and Phi) do substantially worse on this new test set compared to GSM8k.
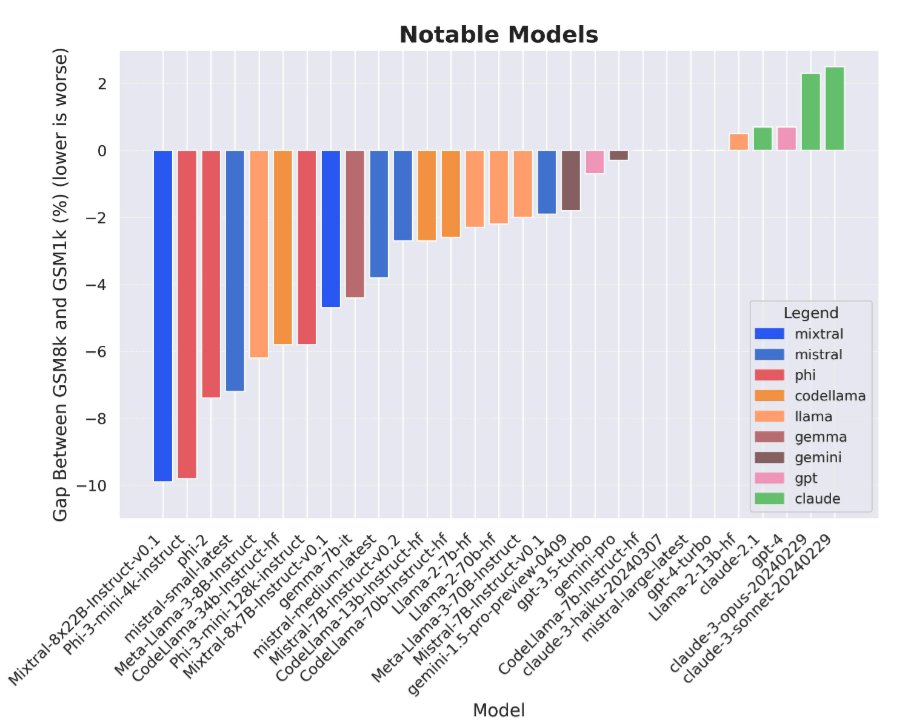
Do LLMs hold knowledge that might be dangerous in the hands of a malicious user? Can hazardous knowledge be unlearned? Introducing WMDP: an open-source eval benchmark of 4,157 multiple-choice questions that serve as a proxy measurement of LLM’s risky knowledge in biosecurity,…
📣 Announcing the release of the WMDP LLM benchmark, designed by Scale’s Safety, Evaluations, and Analysis Lab (SEAL) in partnership with @ai_risks (CAIS)! 🧵 scale.com/blog/measuring…
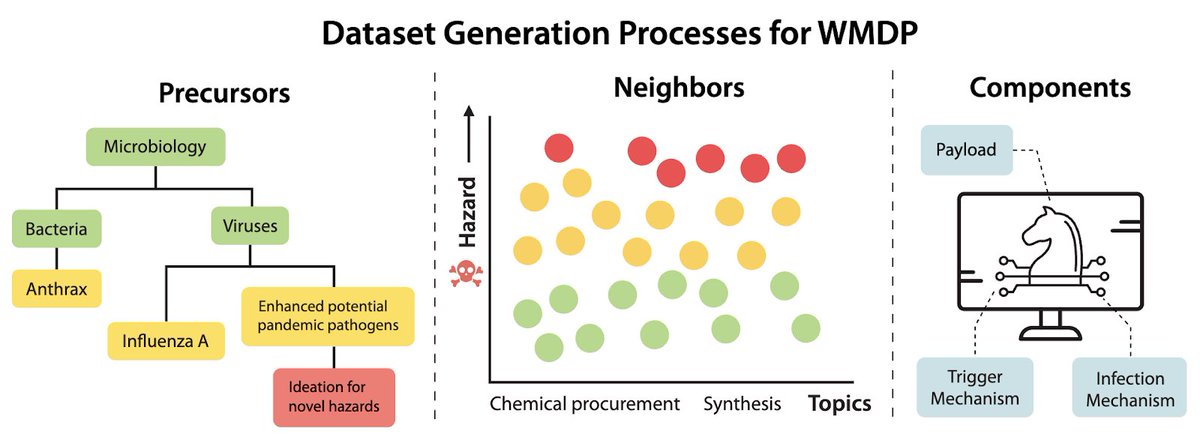
New paper! Q-probe is a lightweight approach to RL on top of LLMs. We learn a linear value function on the LLM embeddings and use a variant of rejection sampling to define a policy. Results linked in the thread from first author @ke_li_2021 on coding problems and RLHF. 🧵
We propose Q-probe, a simple technique that improves coding and alignment for LLM, without requiring fine-tuning!. The idea is to learn a "task vector" in the hidden space and use it to select from multiple candidate generations. arxiv.org/abs/2402.14688
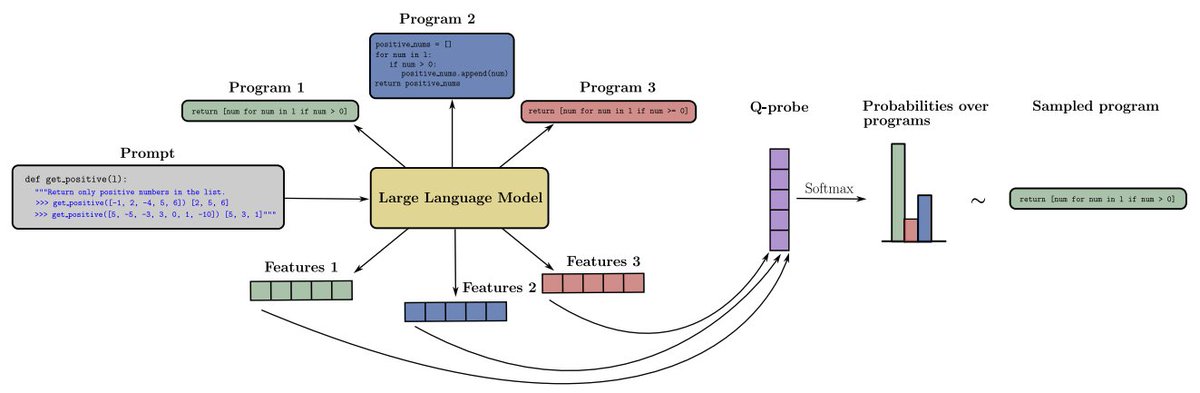
My colleague Willow Primack used DALL-E to illustrate Allen Ginsberg’s Howl, and it was just too good not share (with permission). Here’s a teaser. Howl, Illustrated by AI I saw the best minds of my generation destroyed by madness, starving hysterical naked
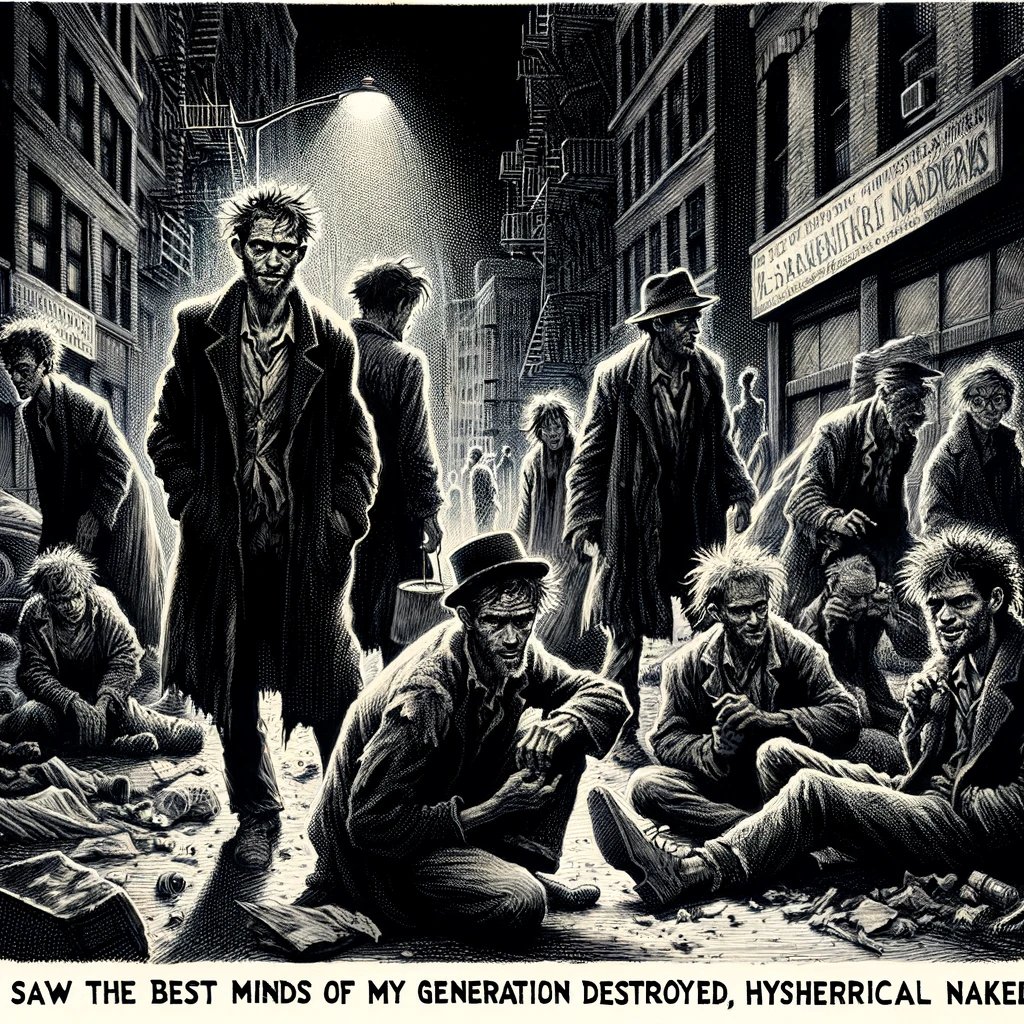
Excited to share our work, "Skill Set Optimization", a continual learning method for LLM actors that: - Automatically extracts modular subgoals to use as skills - Reinforces skills using environment reward - Facilitates skill retrieval based on state allenai.github.io/sso 🧵
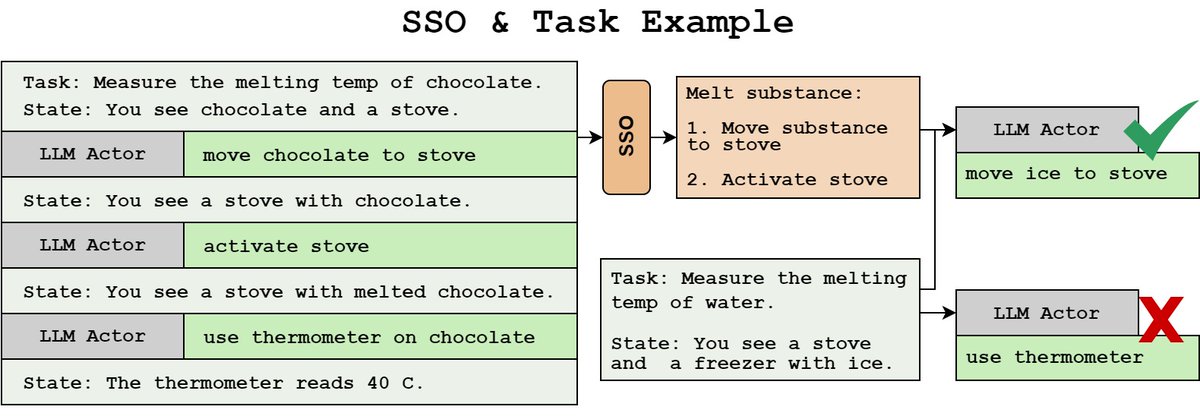
United States Trends
- 1. #OnlyKash 60,4 B posts
- 2. Starship 201 B posts
- 3. Jaguar 64,6 B posts
- 4. Celtics 18,2 B posts
- 5. Nancy Mace 89,6 B posts
- 6. Sweeney 12,1 B posts
- 7. SpaceX 217 B posts
- 8. Medicare and Medicaid 25,7 B posts
- 9. Jose Siri 2.931 posts
- 10. Jim Montgomery 4.202 posts
- 11. Dr. Phil 8.679 posts
- 12. Linda McMahon 3.980 posts
- 13. Stephen Vogt 1.510 posts
- 14. $MCADE 1.379 posts
- 15. Monty 11,5 B posts
- 16. Dr. Mehmet Oz 8.342 posts
- 17. #LightningStrikes N/A
- 18. Cenk 15,3 B posts
- 19. Lichtman 1.490 posts
- 20. #SpiteMoney N/A
Who to follow
-
𝙷𝚒𝚖𝚊 𝙻𝚊𝚔𝚔𝚊𝚛𝚊𝚓𝚞
@hima_lakkaraju -
Trustworthy ML Initiative (TrustML)
@trustworthy_ml -
Hua Shen✨
@huashen218 -
Maarten Sap (he/him)
@MaartenSap -
Suchin Gururangan
@ssgrn -
Explainable AI
@XAI_Research -
Oana-Maria Camburu
@oanacamb -
Zekun Wang (Seeking 25Fall PhD/Job) 🔥
@ZenMoore1 -
Sumanth
@sumanthd17 -
Sameer Singh
@sameer_ -
Peter Hase
@peterbhase -
Alon Jacovi
@alon_jacovi -
Swabha Swayamdipta ✈️ EMNLP'24 🏖️
@swabhz -
Valerie Chen
@valeriechen_ -
Ana Marasović
@anmarasovic
Something went wrong.
Something went wrong.