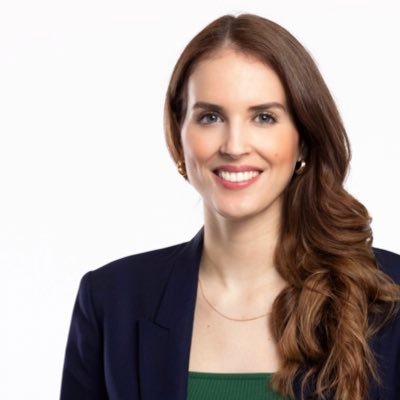
Lisa Adams
@LCAdamsRadRadiology MD & Assistant Professor @TU_Muenchen | Former Postdoctoral Fellow @StanfordMedicine | Focused on #Radiology, #AI, #MolecularImaging
Similar User

@florigo75

@slavaukrtoken

@SwentyTix

@Christian81991S

@asimxq

@COMFORT_EU

@solanomtnez

@ABOMcert

@mbsclips

@k_bressem

@Fel_Busch
@LCAdamsRad @k_bressem discuss prognostic value of CXR-Age model in a large test cohort doi.org/10.1148/ryai.2… #ChestRad #aging #Ageing
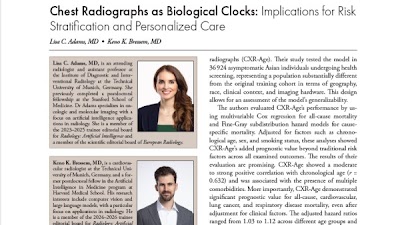
Is there a future for Meta's open source Llama 3 large language model (LLM) in radiology AI applications? Find out in this original research study by @LCAdamsRad, @DanielTruhn, @Fel_Busch, @k_bressem et al. @TU_Muenchen @ChariteBerlin bit.ly/4cpeJwJ
@LCAdamsRad @k_bressem discuss the potential clinical applications of biological age from CXRs doi.org/10.1148/ryai.2… #aging #AI #MachineLearning
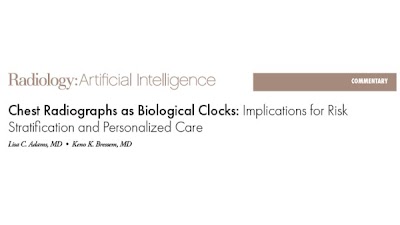
Our new @npjDigitalMed commentary analyzes the EU AI Act's impact on healthcare. We explain the risk-based approach, explore implications for radiology AI, and discuss regulatory challenges for medical AI. Full article here: rdcu.be/dQDBn Thanks to @Fel_Busch @k_bressem…
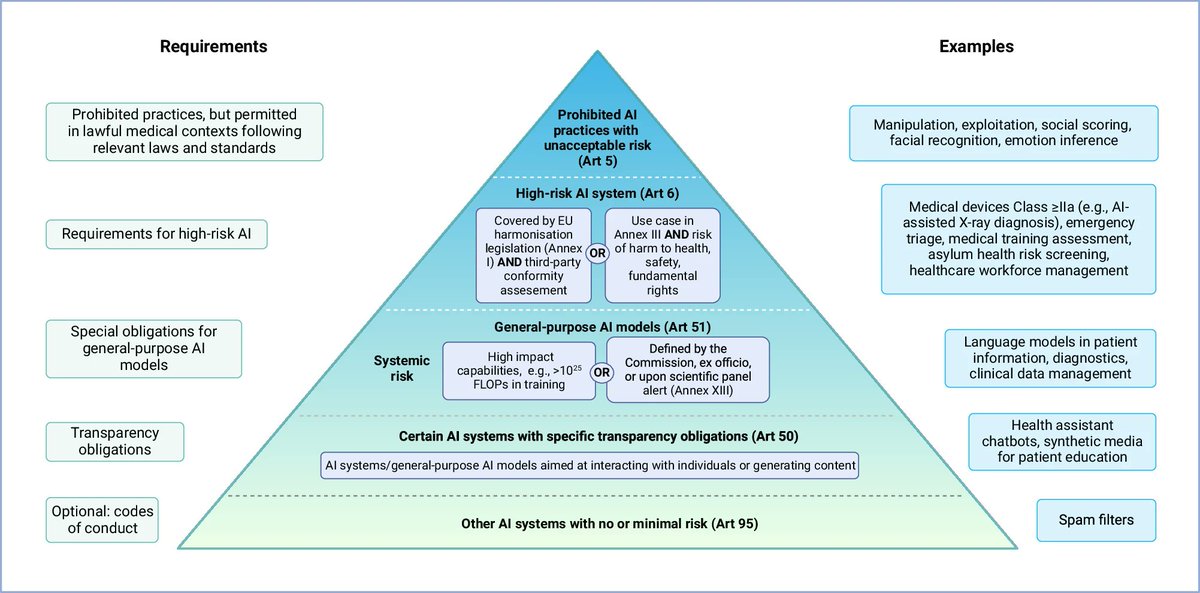
An excellent reference point for the key elements of the @EU_Commission's ground breaking #ArtificialInteligence Act and its relevance to healthcare. Don't forget to bookmark this resource to for easy to access references to the relevant chapters. nature.com/articles/s4174…
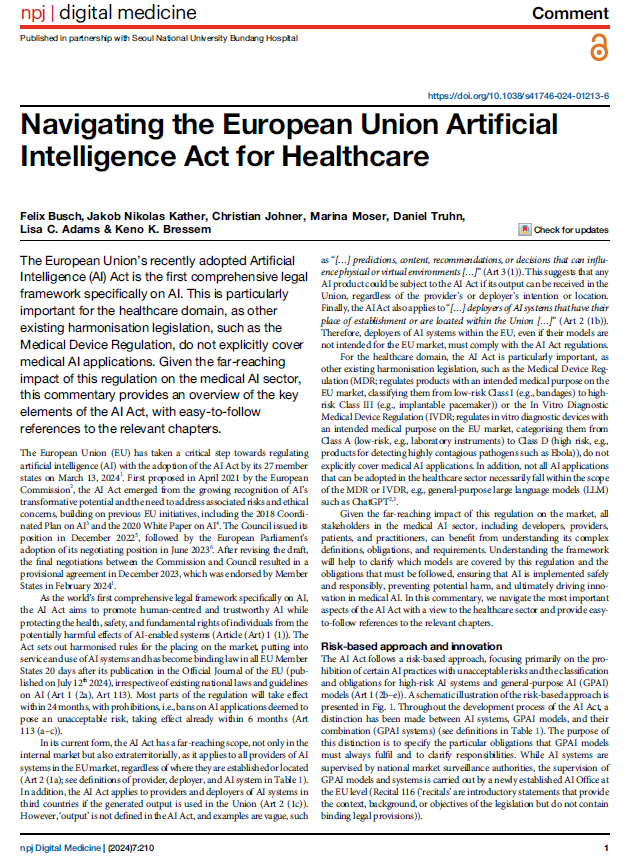
T4. There are numerous resources for AI and informatics from @RSNA, @SIIM_Tweets, @RadiologyACR, and others. It can be overwhelming, especially when first exploring this domain! Here’s a list of compiled resources to help you on your journey: bit.ly/36QhVFz #RadAIChat
T5. Beyond reporting, CLAIM educates on best practices in AI study design and execution. It can inform curriculum development for AI in radiology and promote standardization across the field, improving research quality and clinical translation. #RadAIchat
T5. CLAIM serves as a roadmap for comprehensive AI study reporting in radiology. It guides researchers in study design, helps journals maintain reporting standards, and enables readers to critically assess AI research quality. #RadAIchat
T4. CLAIM 2024 replaces 'ground truth' and 'gold standard' with 'reference standard'. This change acknowledges uncertainty in medical data labeling, aligns with other reporting guidelines like STARD, and avoids implying absolute certainty in benchmarks. #RadAIchat
T4. ‘Ground truth’ implies absolute certainty, while ‘gold standard’ suggests a fixed benchmark. CLAIM 2024 adopts ‘reference standard’ to acknowledge the inherent uncertainty in medical data labeling and align with other reporting guidelines. #RadAIchat
T3. CLAIM 2024 adds ‘not applicable’ option, adopts ‘reference standard’ instead of ‘ground truth’/’gold standard’, includes image acquisition details. Simplifies by removing data element definitions. Not extended to radiomics research, keeping focus on AI in imaging. #RadAIchat
T3. CLAIM 2024 emphasizes precise terminology: ‘internal/external testing’ preferred over ambiguous ‘validation’. Encourages sharing protocols and data/code. Updates aim to standardize AI reporting, increase transparency, and facilitate comparison between studies. #RadAIchat
T2. CLAIM serves multiple stakeholders: authors use it for thorough reporting, reviewers for completeness assessment, and readers to evaluate study quality and reproducibility. #RadAIchat
T2. CLAIM guides authors in clear AI research presentation. It covers the entire manuscript structure, ensuring critical details on data, model architecture, training, and evaluation are reported. #RadAIchat
T1. The recently updated CLAIM guideline is a comprehensive guideline for AI in medical imaging. It covers 44 items across all manuscript sections, ensuring thorough reporting of data, methods, and results. #RadAIchat
T1. Key AI reporting guidelines in radiology include CLAIM, STARD-AI, MI-CLAIM, CONSORT-AI, SPIRIT-AI, FUTURE-AI, MINIMAR, and RQS. Each addresses specific aspects of AI research reporting and reproducibility. #RadAIchat
Hi there! I'm Lisa Adams, a physician scientist and radiologist at Technical University Munich. Excited to discuss CLAIM guidelines and their impact on AI reporting in radiology. #RadAIchat
📅 Mark your calendar for the Aug. 7 #RadAIchat at 8 PM ET on "Checklist for #AI in Medical Imaging (CLAIM): 2024 Update" moderated by @Klonmich and panelists @LCAdamsRad @cekahn @AliTejaniMD #radiomics #AI #DL #radres @RSNA @HElhalawaniMD
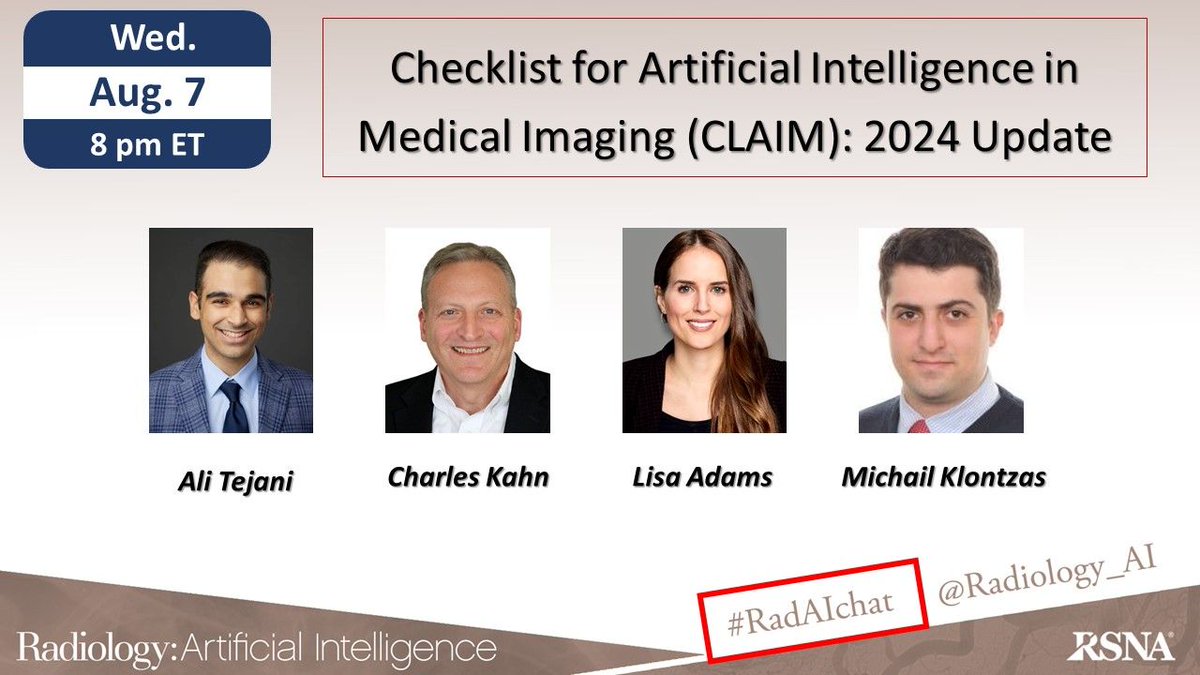
#DeepLearning model highly accurate at classifying cardiac implants chest xrays doi.org/10.1148/ryai.2… @HugoAerts @k_bressem @LCAdamsRad #ChestRad #AI #MachineLearning
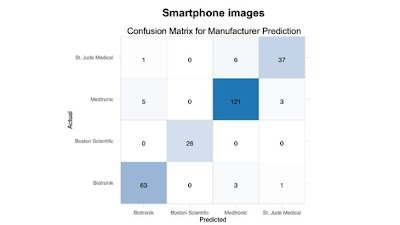
#DeepLearning model highly accurate at classifying cardiac implants chest xrays doi.org/10.1148/ryai.2… @Fel_Busch @k_bressem @LCAdamsRad #CXR #CVRad #DICOM
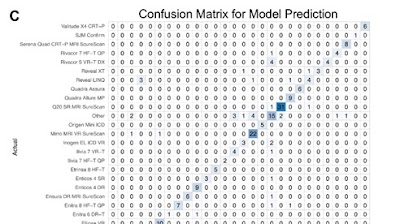
United States Trends
- 1. #Arcane 212 B posts
- 2. Jake Paul 1,04 Mn posts
- 3. Jayce 45,8 B posts
- 4. #SaturdayVibes 2.670 posts
- 5. Serrano 247 B posts
- 6. Vander 14,6 B posts
- 7. Good Saturday 23,6 B posts
- 8. #HappySpecialStage 87,1 B posts
- 9. maddie 19,1 B posts
- 10. #saturdaymorning 1.893 posts
- 11. Jinx 104 B posts
- 12. #SaturdayMood 1.268 posts
- 13. Isha 34,4 B posts
- 14. Rizwan 8.134 posts
- 15. Father Time 10,8 B posts
- 16. Woop Woop 1.275 posts
- 17. Super Tuna 22,5 B posts
- 18. Canelo 17,7 B posts
- 19. The Astronaut 30,5 B posts
- 20. Babar 11,6 B posts
Who to follow
Something went wrong.
Something went wrong.