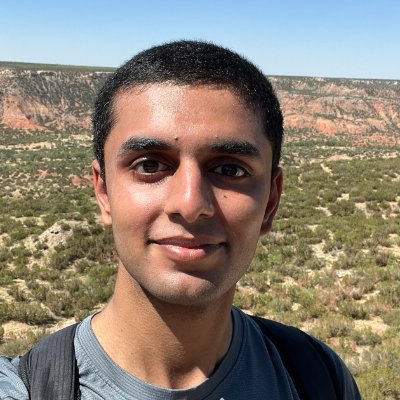
Ashwin Devaraj
@AshwinDevaraj3Training LLMs at @snowflakedb Ex-@neeva, Math+CS at UT Austin Fan of mountain hiking, singing, and other surrogate activities
Similar User

@tanyaagoyal

@prasann_singhal

@xiye_nlp

@LiyanTang4

@ForBo7_

@brunchavecmoi

@sidilu_pluslab

@anuj_diwan

@YatingWu96

@yasumasa_onoe

@hungting_chen

@gkambhat

@albertyu101

@ManyaWadhwa1

@zhang_shujian
There's no way this paragraph is written by a human alone. It's a very informative survey though
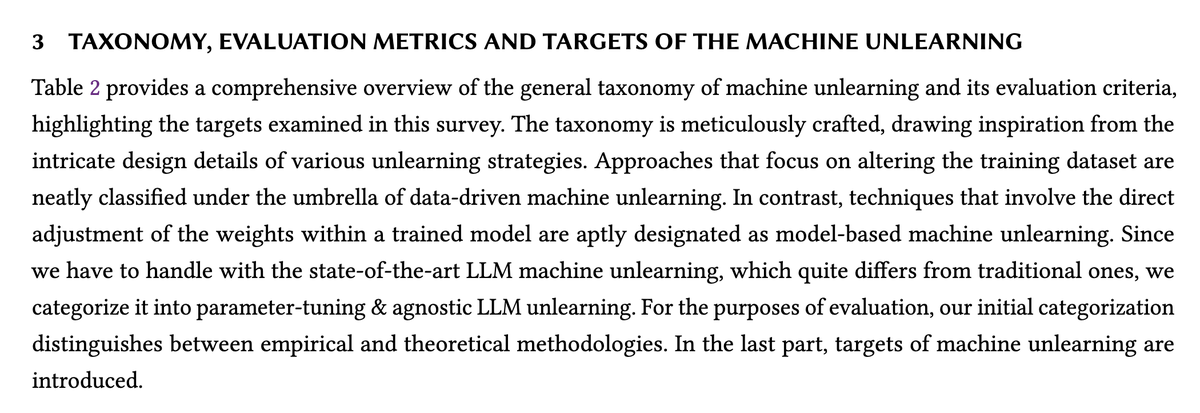
3rd week in a row, 3rd LLM from @SnowflakeDB ... Arctic-TILT is a 800M model that has GPT-4 quality performance on information extraction tasks, as measured by the DocVQA benchmark. And it fits in an A10!
Snowflake’s Arctic-TILT model, powering our Document, Al beats GPT-4 with just 0.8B parameters, securing a top spot in the standard benchmark for document understanding DocQVA.
We just published our next blog post in the Arctic Cookbook series about how we generated and managed our training data for Arctic. Up next, we'll talk about getting the most from your hardware. medium.com/snowflake/snow…
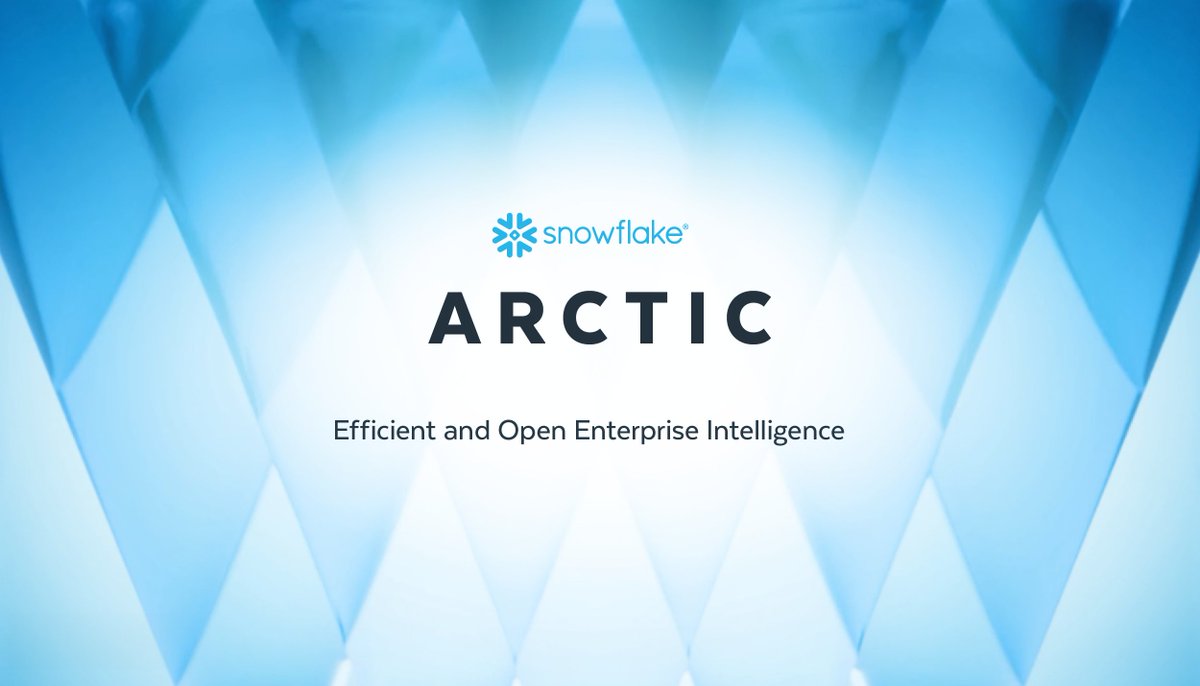
To maximize #SnowflakeArctic's training throughput, we optimized at all levels of the system stack from developing custom cuda kernels to co-designing the model architecture with the system to enable communication overlap. @Reza_LOD offers a glimpse into these optimizations.
1/4 Have you wondered how to optimize sys-perf for training Arctic-like models (MoE arch)? Let’s dive in! Our first technique: custom fused kernels. By crafting these kernels, we streamline irregular and sparse operators, boosting efficiency. #SnowflakeArctic #SystemOptimization
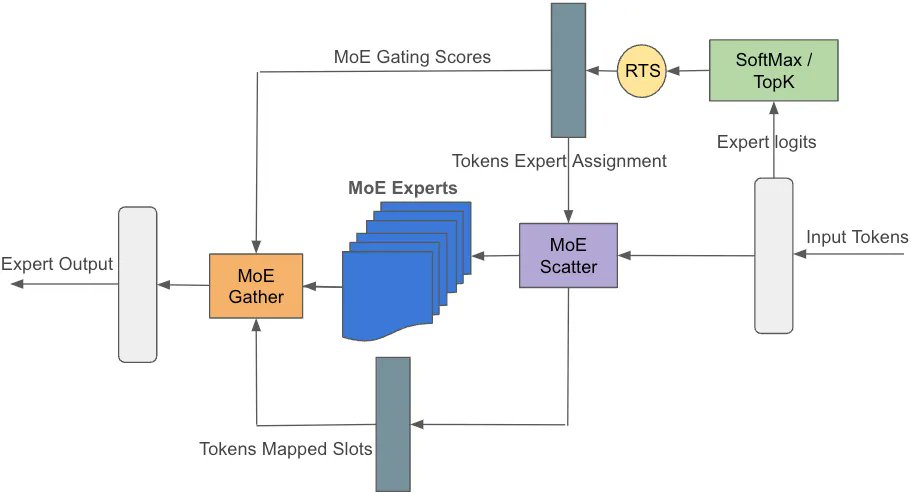
Good morning: @SnowflakeDB’s new 480B parameter #LLM is made of 128 experts! It’s bigger than #Grok and is now the largest *fully open source (Apache 2.0* LLM! 🧵👇 how does it compare to Llama 3, Mixtral, and GPT4?
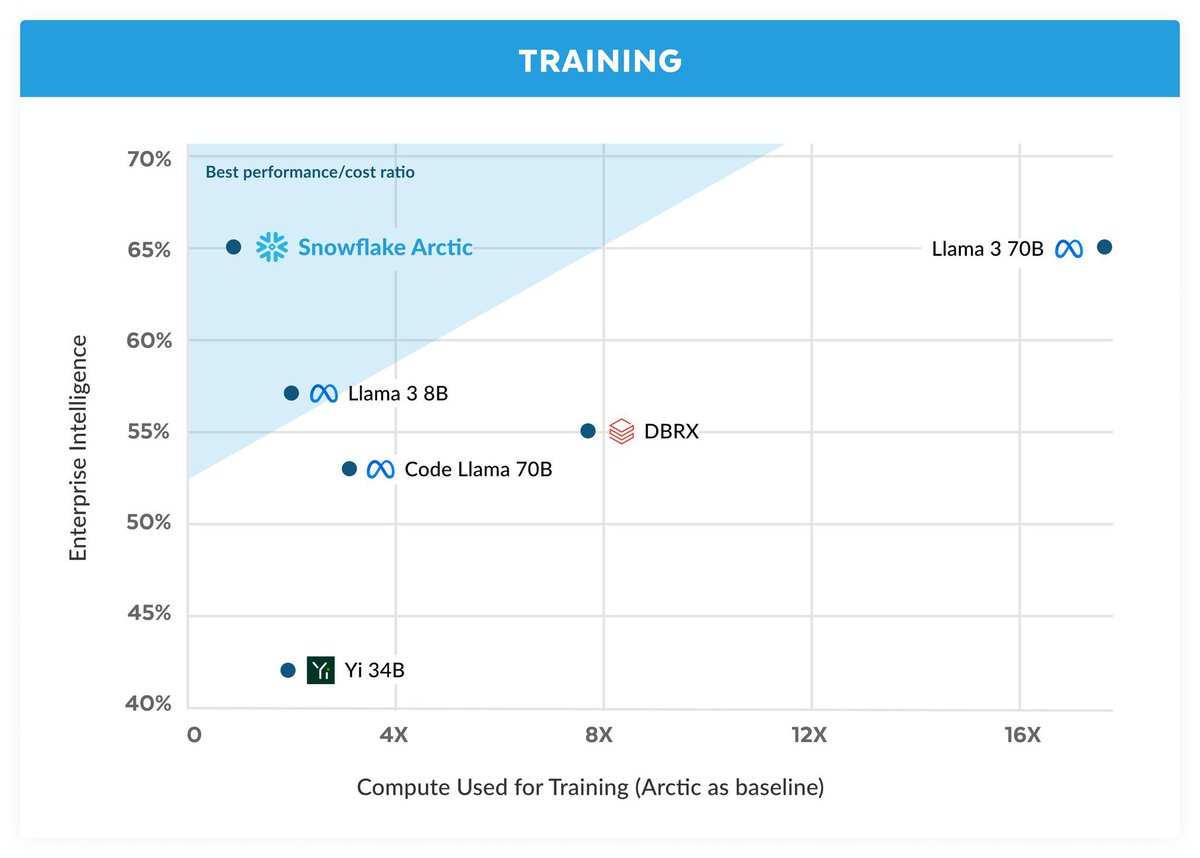
1/n What are the benefits of MoE? Our study shows that MoE models can achieve better quality with less compute. In fact, our MoE-1.6B model outperformed a 6.5B dense model while requiring at least 4x less compute to train! Read on for more findings on MoE ablations 🧵
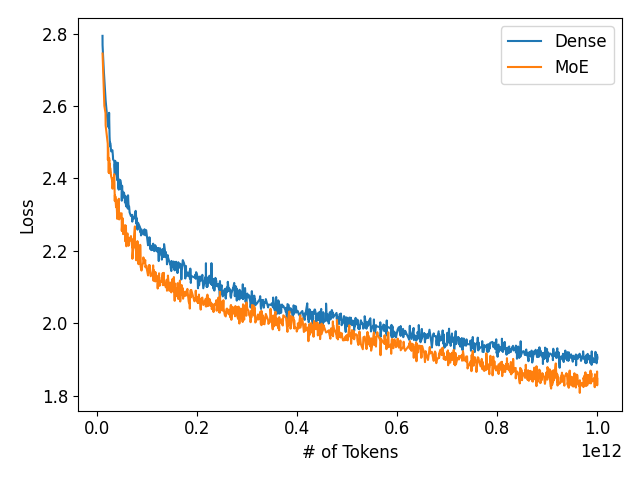
What a roller coaster the past few months have been! I'm excited and grateful for the opportunity to collaborate with such a badass team. Stay tuned for more updates - blog posts, model improvements, and more...
.@SnowflakeDB is thrilled to announce #SnowflakeArctic: A state-of-the-art large language model uniquely designed to be the most open, enterprise-grade LLM on the market. This is a big step forward for open source LLMs. And it’s a big moment for Snowflake in our #AI journey as…
We’re excited to announce Snowflake is acquiring Neeva and its team of talented engineers, to make search even more intelligent at scale across the #DataCloud. See the blog post for more information: okt.to/DYI5Ue #GenAI #LLM
This truck is on the move!! 🚚 Have you seen it around the city? 🏙️ Add your shots here 📸⤵️
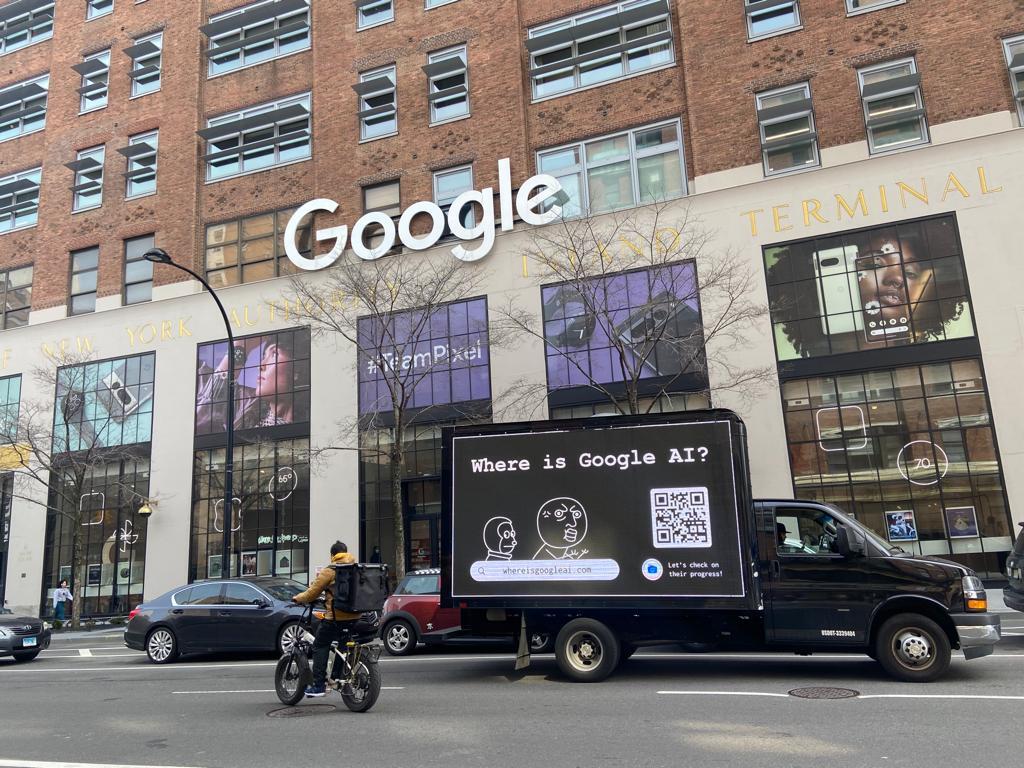
Still waiting on AI search promised by the big guys? Same. We're keeping an eye on them here: 👁️🗨️ Google’s Bard isbardavailable.com 👁️🗨️ Bing’s AI chatbot isbingaiavailable.com Oh, did we mention NeevaAI is available NOW? No ads. No waitlist. neeva.com
1/ Ten blue links headed to a museum near you!! @Neeva is applying cutting edge AI to definitely change up the search experience! Not only are we providing real time, cited AI, because we are making the whole search experience a breeze! 🧵
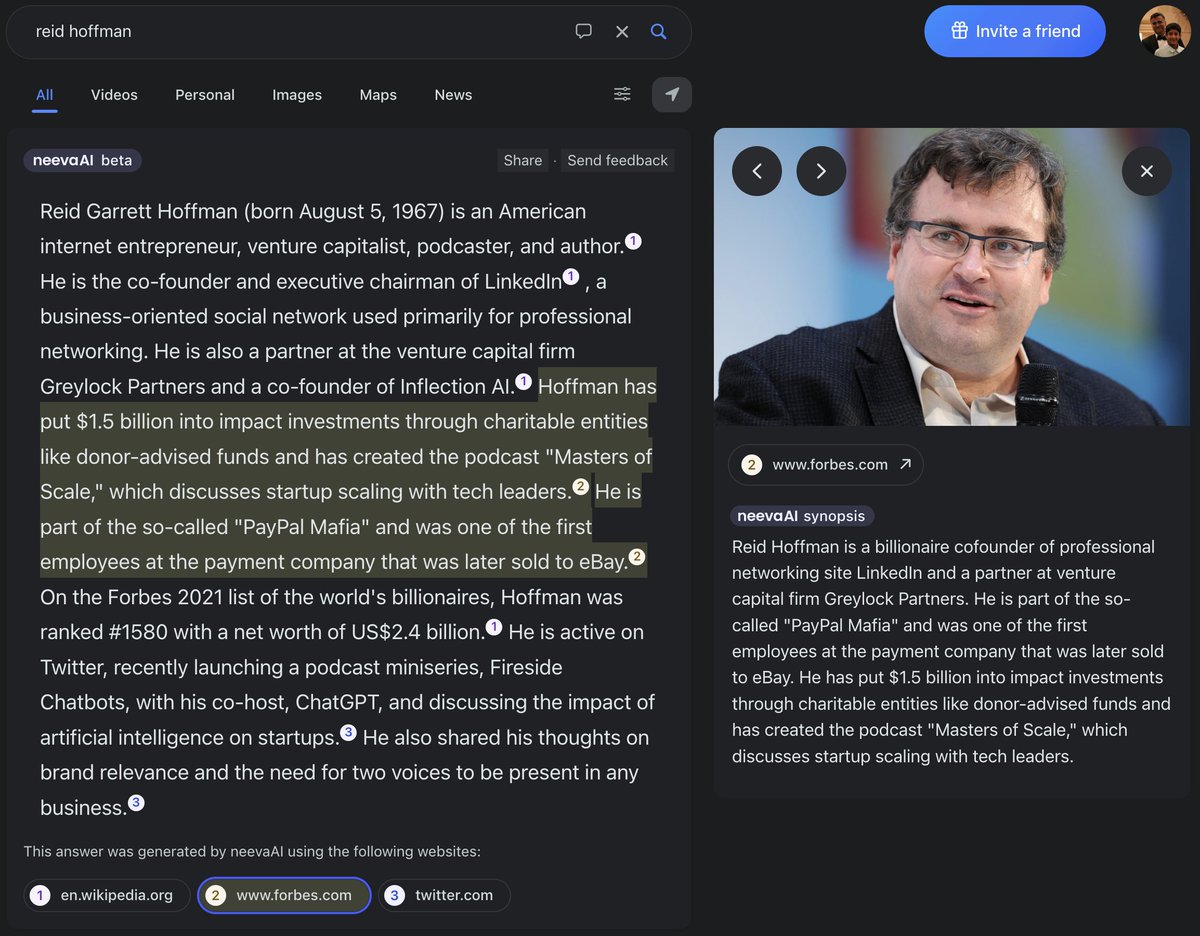
Check out the oral presentation TODAY for our #ACL2022 #acl2022nlp work "Evaluating Factuality in Text Simplification" (done w/ William Sheffield, @jessyjli , and @byron_c_wallace). It's at 5 PM in the Generation 1 section (Wicklow Hall 1)! Please drop by if you're in Dublin!
We're excited to share our #acl2022nlp work on characterizing factual errors in text simplification! We present a new annotation scheme and use it to categorize the kinds of factual errors found in popular simplification datasets and models. arxiv.org/abs/2204.07562 (1/2)
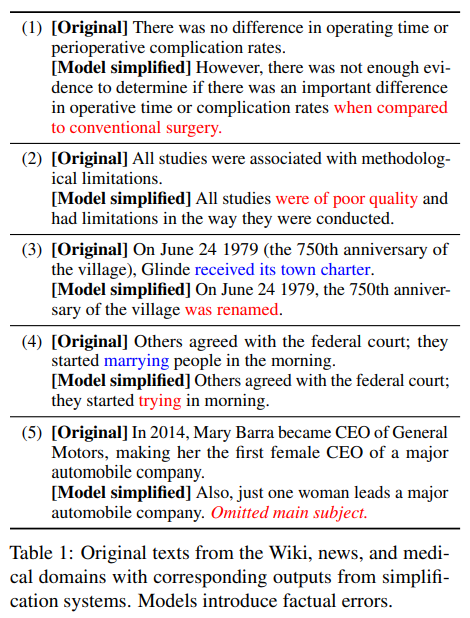
Our work w/ @AshwinDevaraj3, William Sheffield, and @byron_c_wallace on factuality & simplification has been selected as an Outstanding Paper at ACL 2022! #acl2022nlp
We're excited to share our #acl2022nlp work on characterizing factual errors in text simplification! We present a new annotation scheme and use it to categorize the kinds of factual errors found in popular simplification datasets and models. arxiv.org/abs/2204.07562 (1/2)
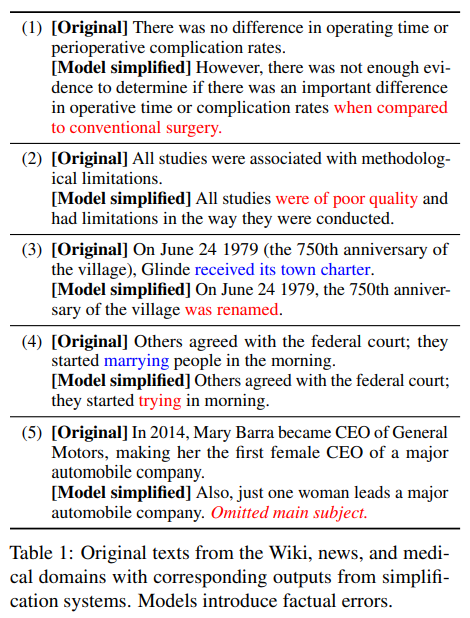
United States Trends
- 1. Celtics 21,4 B posts
- 2. Cavs 21 B posts
- 3. #OnlyKash 70,1 B posts
- 4. #MCADE N/A
- 5. Pat Murphy 2.151 posts
- 6. Nancy Mace 95,2 B posts
- 7. Cenk 18,1 B posts
- 8. Mendoza 9.570 posts
- 9. Jaguar 68,9 B posts
- 10. Joey Galloway N/A
- 11. Linda McMahon 6.345 posts
- 12. Starship 214 B posts
- 13. Medicare and Medicaid 30,3 B posts
- 14. #spitemoney N/A
- 15. College Football Playoff 3.438 posts
- 16. SpaceX 230 B posts
- 17. Lichtman 3.200 posts
- 18. Sweeney 12,2 B posts
- 19. Mobley 2.118 posts
- 20. #LightningStrikes N/A
Who to follow
-
Tanya Goyal
@tanyaagoyal -
Prasann Singhal
@prasann_singhal -
Xi Ye
@xiye_nlp -
Liyan Tang
@LiyanTang4 -
Salman Naqvi
@ForBo7_ -
Fangyuan Xu
@brunchavecmoi -
SIDI LU
@sidilu_pluslab -
Anuj Diwan
@anuj_diwan -
Yating Wu
@YatingWu96 -
Yasumasa Onoe
@yasumasa_onoe -
Hung-Ting Chen
@hungting_chen -
Gauri Kambhatla
@gkambhat -
Albert Yu
@albertyu101 -
Manya Wadhwa
@ManyaWadhwa1 -
Shujian Zhang
@zhang_shujian
Something went wrong.
Something went wrong.