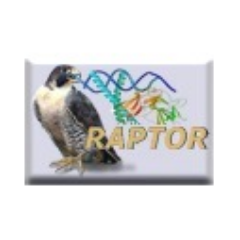
Jinbo Xu
@jinboxu_chicagoInterested in Machine Learning, Optimization and Bioinformatics
Similar User

@KathyYWei1

@ProteinBoston

@minkbaek

@generate_biomed

@proteinrosh

@ml4proteins

@MoAlQuraishi

@AI_Proteins

@JustasDauparas

@bahl_lab

@BrianHie

@deboramarks

@_JosephWatson

@sokrypton

@namrata_anand2
very honored to be recognized by the community.
Congratulations to @jinboxu_chicago, who was selected as an ISCB Fellow for the Class of 2023 for the discovery of computational methods and development of software programs that have revolutionized protein structure prediction! Read more about it here: buff.ly/3Pn6k5c
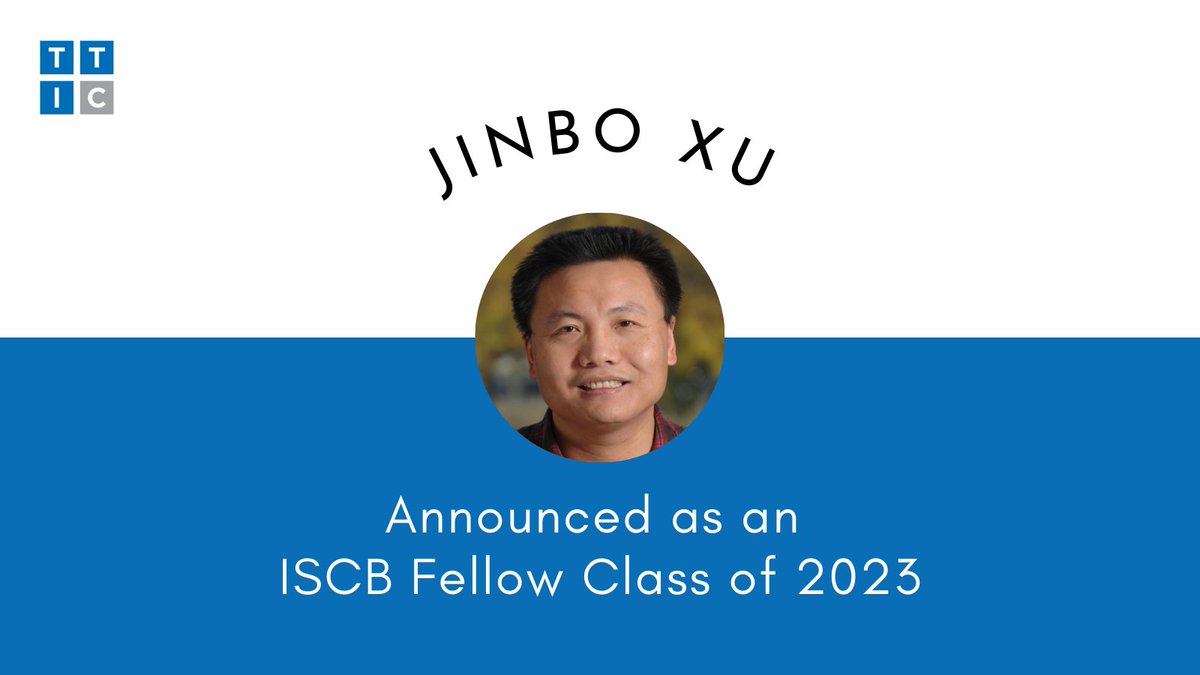
An end-to-end deep learning method for protein side-chain packing and inverse folding | PNAS pnas.org/doi/10.1073/pn… In this paper we present an end-to-end deep learning algorithm that may design amino acid sequences while taking into consideration side-chain conformations.
Deep Learning for Flexible and Site-Specific Protein Docking and Design biorxiv.org/content/10.110… This end-to-end learning algorithm not only produces accurate prediction of antigen-antibody binding pose, but also can design antibody CDRs and even a binder.
An end-to-end deep learning method for rotamer-free protein side-chain packing biorxiv.org/content/10.110…
A Deep SE(3)-Equivariant Model for Learning Inverse Protein Folding biorxiv.org/content/10.110… Our latest work on fixed-backbone protein sequence design.
Protein-protein interfacial contact prediction using ESM-MSA and graph convolution. Ziwei Xie @jinboxu_chicago biorxiv.org/content/10.110…
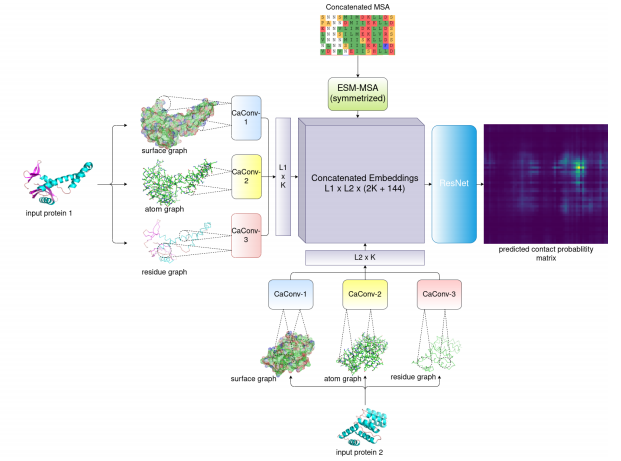
Our July issue is now live! Highlights include an accurate and efficient protein model refinement with deep learning, our editorial on reusability, and an evidence-based recommender system for high-entropy alloys. go.nature.com/3rlyqQ7
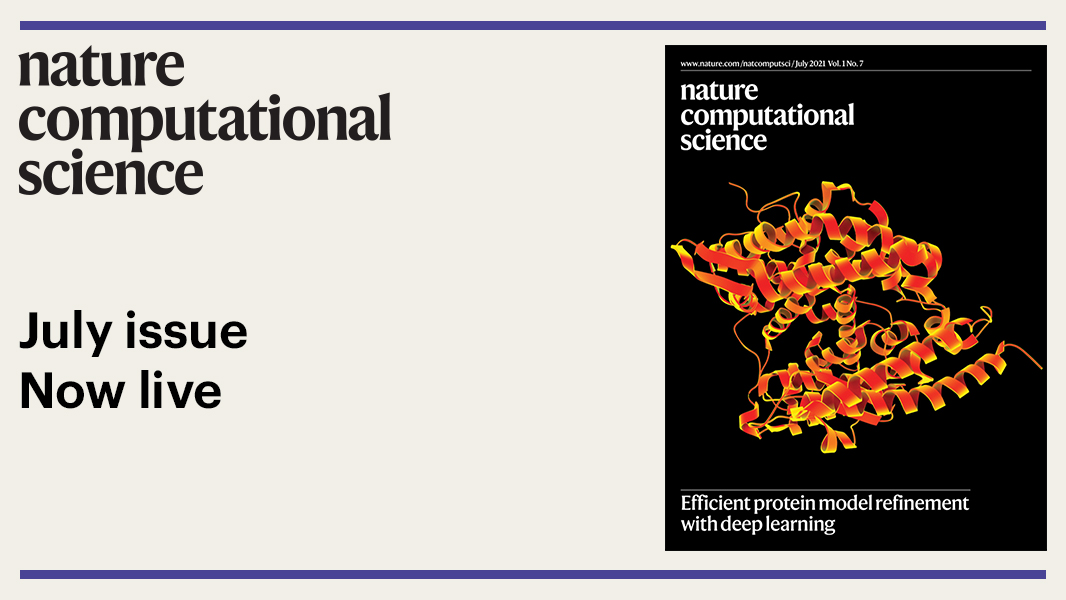
Don't miss a graph neural network approach for protein structure refinement that is substantially faster than other methods, allowing researchers to refine protein models quickly without sacrificing accuracy: go.nature.com/3isGV7R
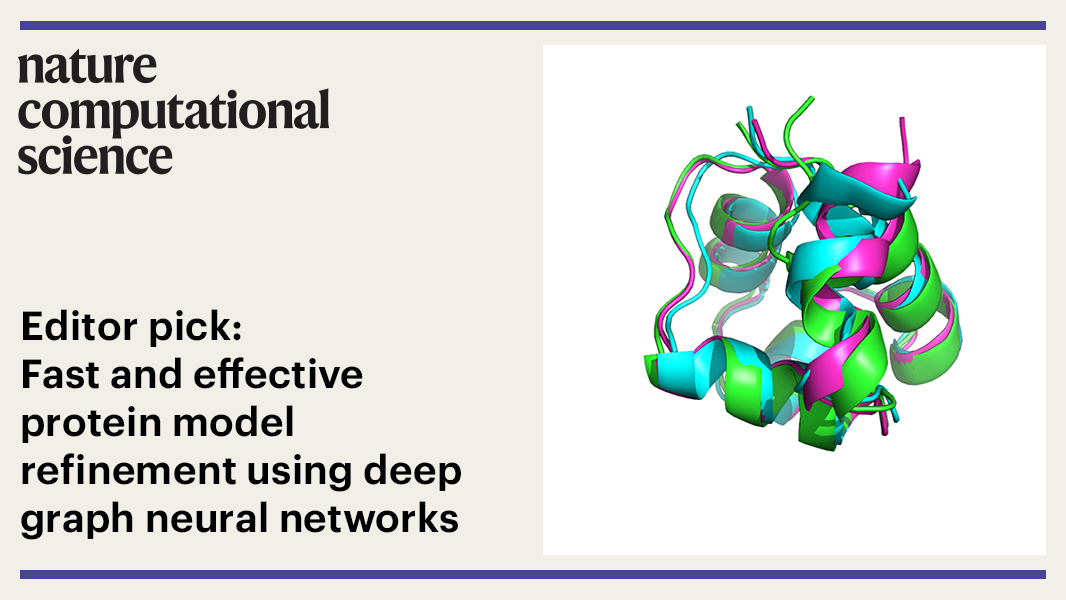
In a recent Article, Xiaoyang Jing and Jinbo Xu (@jinboxu_chicago) demonstrate that graph neural networks can be utilized to achieve fast and effective protein model refinement (rdcu.be/coKsz). nature.com/articles/s4358…
The breakthrough in protein structure prediction | Biochemical Journal | Portland Press portlandpress.com/biochemj/artic…
template-free modeling has been greatly improved by deep learning. Wonder how well we may improve template-based modeling by DL?
Graph neural networks enable protein model refinement within minutes biorxiv.org/content/10.110…
Fixed the GIF! Turns out I missed a NN. Apologies to @jinboxu_chicago for excluding his DNN for TBM!
AI/ML tweeps, here is a must read. It’s a wonderful article questioning whether we are spending our valuable brain cycles on the right research questions. If you disagree, I’d love to hear why. If you agree, what can be done to accelerate this? technologyreview.com/2020/08/18/100…
My article is one of the @PLOSCompBiol most-cited papers of 2017! #plosmostcited #PLOSCompBiolAuthor journals.plos.org/ploscompbiol/a… https://t.co/CPUZ17YBcE . This is the paper that initiated the revolution of protein structure prediction by deep learning.
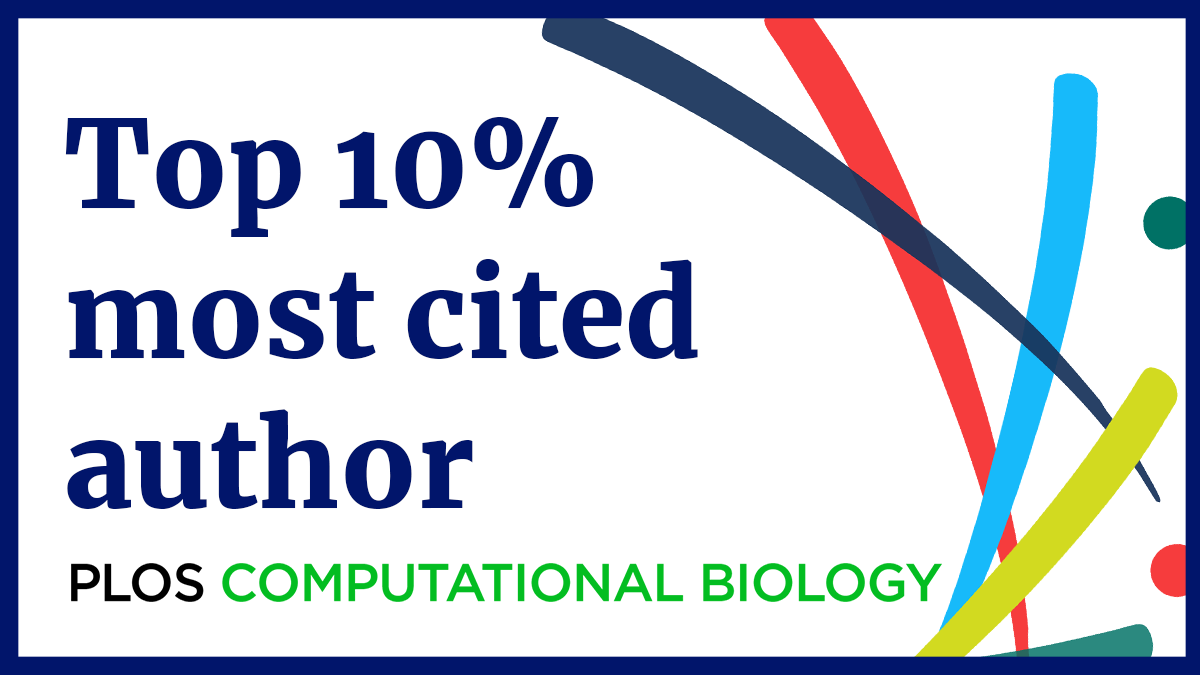
Chinese-Americans, Facing Abuse, Unite to Aid Hospitals in Coronavirus Battle - The New York Times nytimes.com/2020/04/05/nyr…
Great job! Hopefully these models will be useful for fighting the Coronavirus.
United States Trends
- 1. Chiefs 130 B posts
- 2. Josh Allen 52,2 B posts
- 3. 49ers 40 B posts
- 4. Niners 7.898 posts
- 5. Mahomes 36 B posts
- 6. Geno 33,7 B posts
- 7. Super Bowl 18,3 B posts
- 8. WWIII 104 B posts
- 9. Bo Nix 16,1 B posts
- 10. #KCvsBUF 21,1 B posts
- 11. Falcons 20,4 B posts
- 12. Seahawks 27,8 B posts
- 13. Broncos 34,5 B posts
- 14. Chargers 18,2 B posts
- 15. Kyle 46,7 B posts
- 16. Paige 19,4 B posts
- 17. 72 Dolphins 1.216 posts
- 18. Steelers 130 B posts
- 19. Ravens 88,1 B posts
- 20. Lamar 37,5 B posts
Who to follow
-
Kathy Y. Wei, Ph.D.
@KathyYWei1 -
Boston Protein Design and Modeling Club
@ProteinBoston -
Minkyung Baek
@minkbaek -
Generate:Biomedicines
@generate_biomed -
Roshan Rao
@proteinrosh -
Machine learning for protein engineering seminar
@ml4proteins -
Mohammed AlQuraishi
@MoAlQuraishi -
AI Proteins
@AI_Proteins -
Justas Dauparas
@JustasDauparas -
chris bahl
@bahl_lab -
Brian Hie
@BrianHie -
Debora Marks
@deboramarks -
Joseph Watson
@_JosephWatson -
Sergey Ovchinnikov
@sokrypton -
Namrata Anand
@namrata_anand2
Something went wrong.
Something went wrong.