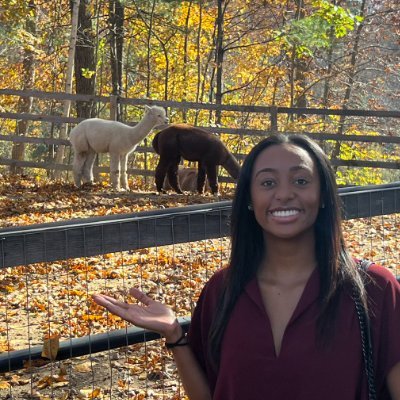
Erika Cardenas
@ecardenas300Partnerships @weaviate_io | Diary about agents, LLM frameworks, and vector databases 🤪
Similar User

@jessie_groot

@etiennedi

@qdrant_engine

@ekzhang1

@tuanacelik

@weaviate_io

@ZainHasan6

@lateinteraction

@lucia_auth

@abidlabs

@jobergum

@bobvanluijt

@reach_vb

@CerebrasSystems

@Avra_b
LLM + Memory + Planning + Tools = Agents 🤖 Last month, Job and I discussed how generative AI is shifting how companies offer customer support. How can we add more layers to our RAG apps to make it more agentic? LLM: Large language model alone Memory: Short-term and long-term…
Adding only a single AI agent to your RAG pipeline can already make a huge difference in performace. By replacing the retrieval component with an retrieval agent, you can supercharge your vanilla RAG pipeline. A retrieval agent can: 1. Decide whether to retrieve data at all 2.…
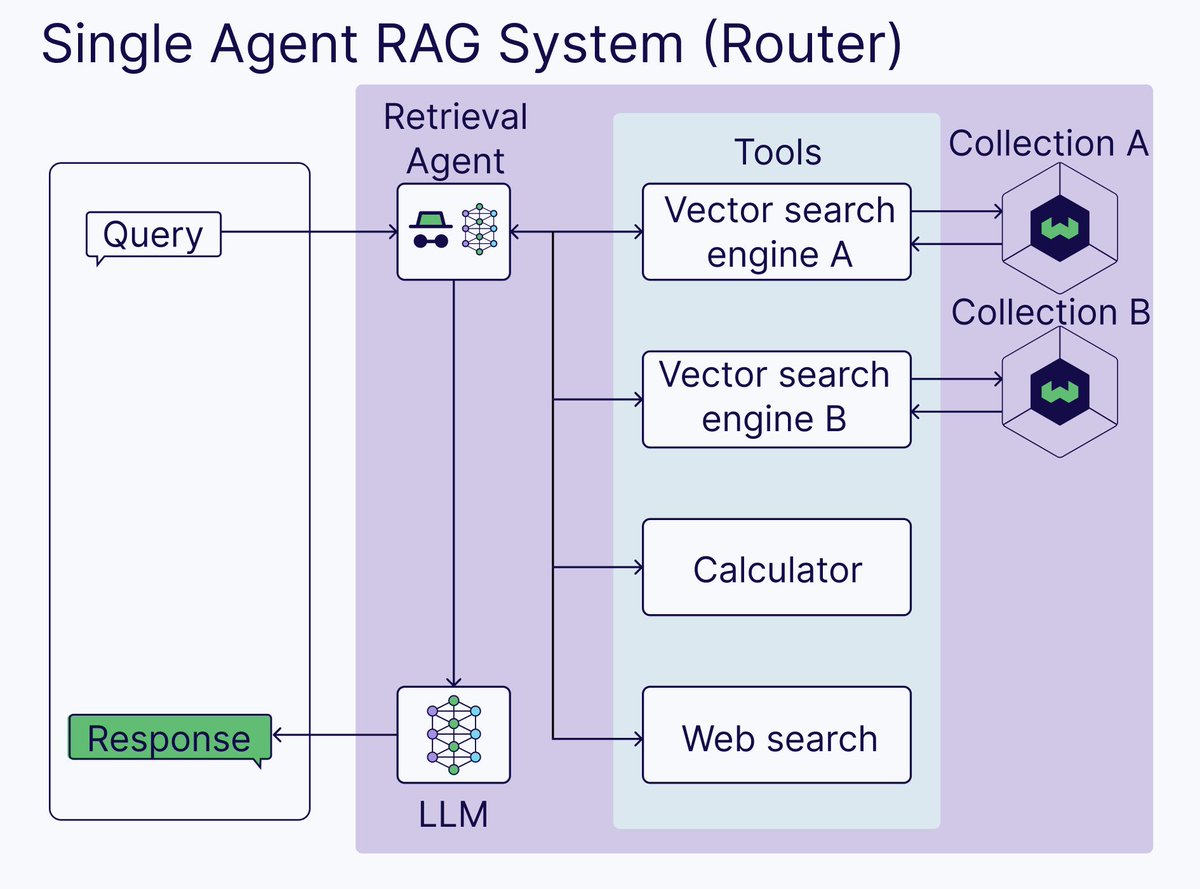
Massive News from Chatbot Arena🔥 @GoogleDeepMind's latest Gemini (Exp 1114), tested with 6K+ community votes over the past week, now ranks joint #1 overall with an impressive 40+ score leap — matching 4o-latest in and surpassing o1-preview! It also claims #1 on Vision…
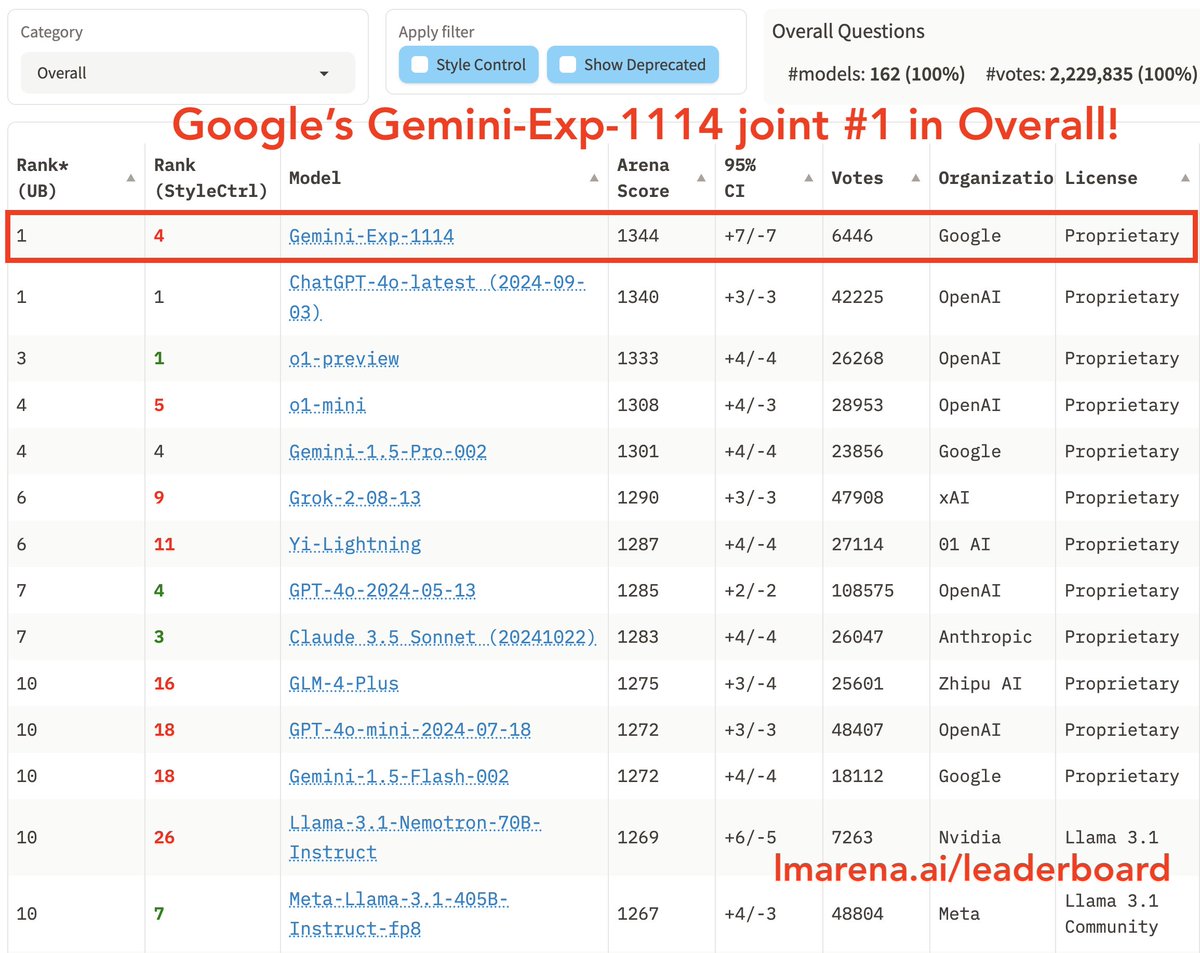
gemini-exp-1114…. available in Google AI Studio right now, enjoy : ) aistudio.google.com
🚀 Big News: AutoGen is Now AG2! 🚀 We’re evolving! With support from the #OSS community, AutoGen is becoming #AG2, a new home for next-gen agentic #AI. Same mission, bigger goals. → Repo: github.com/ag2ai/ag2 ⭐️ → Docs: ag2ai.github.io/ag2 → Same Discord:…
the new frontier: AI agent hosting/serving 👾🛸 the AI/LLM agents stack is a significant departure from the standard LLM stack. the key difference between the two lies in managing state: LLM serving platforms are generally stateless, whereas agent serving platforms need to be…
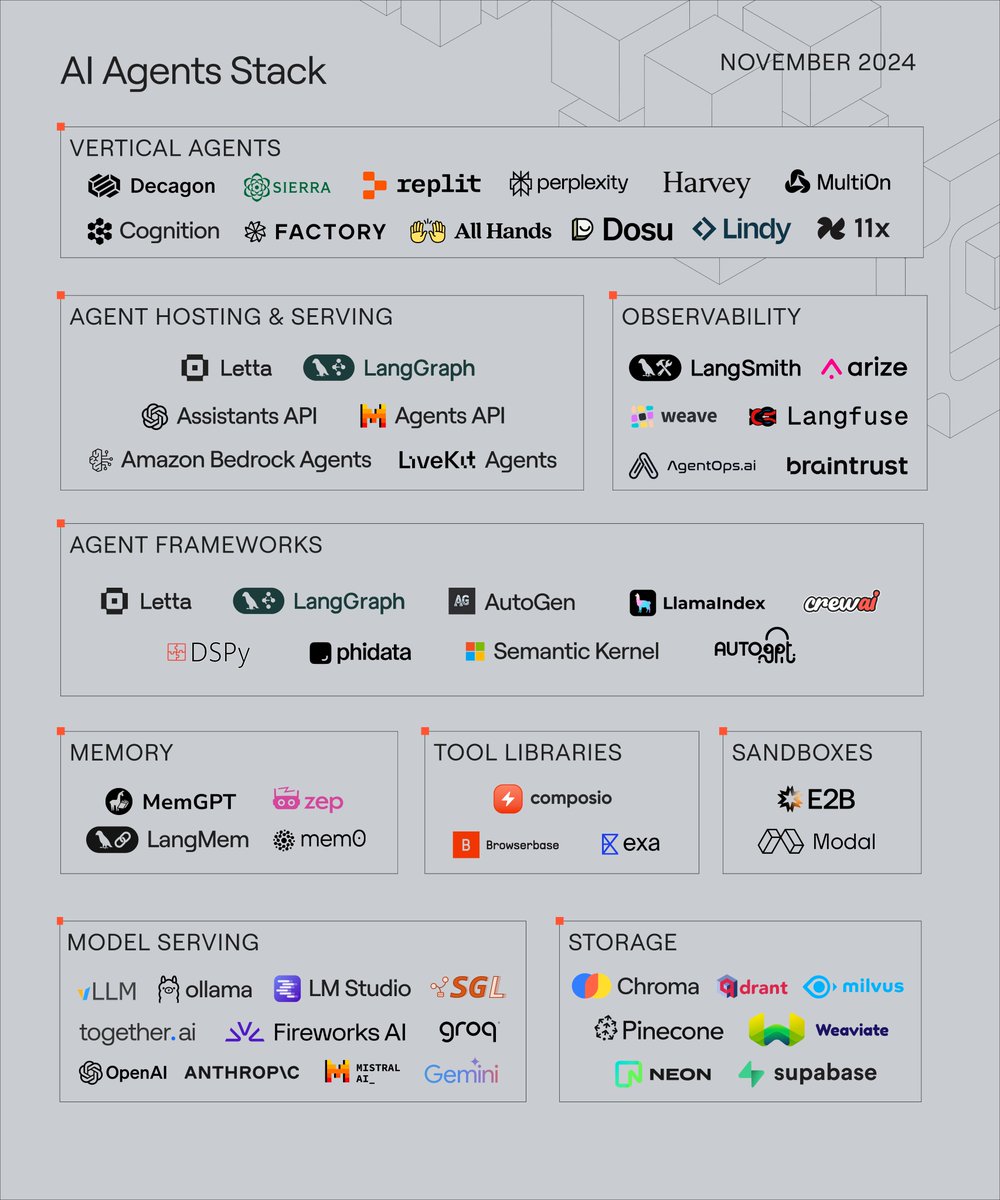
The Agentic RAG party continues! 🎉 I am SUPER EXCITED to publish the 109th Weaviate Podcast with Erika Cardenas (@ecardenas300)! Erika, in collaboration with Leonie Monigatti (@helloiamleonie), have recently published "What is Agentic RAG"! This blog has even been covered in…
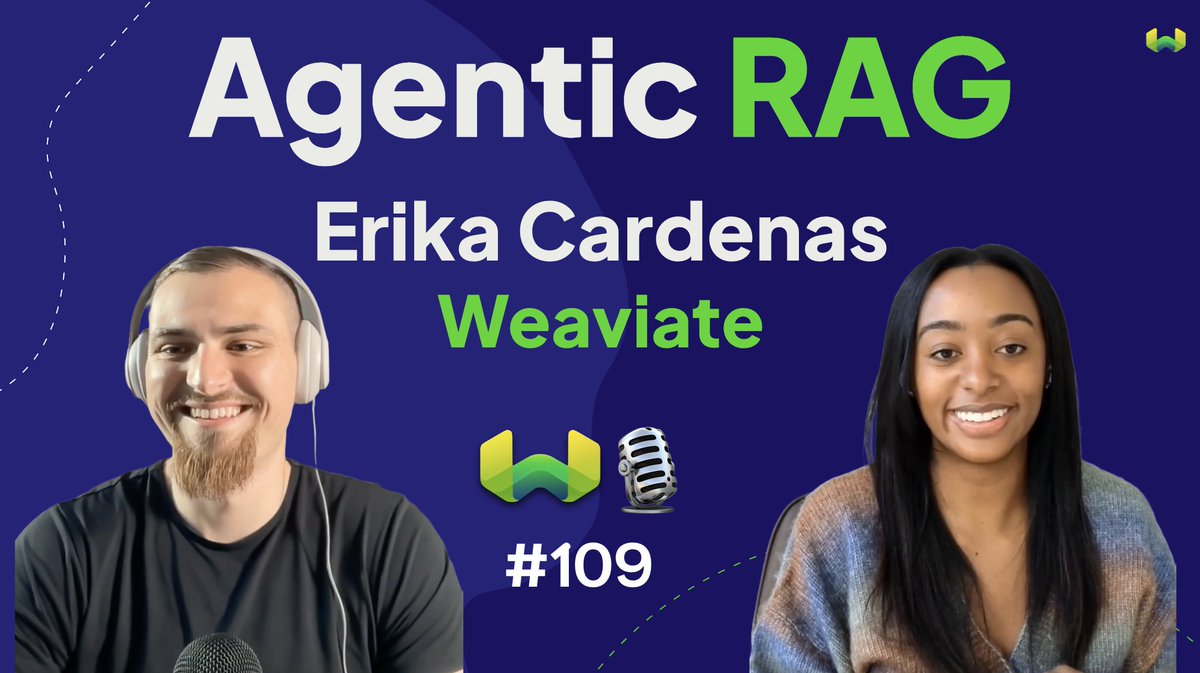
Battle of the RAGs 🤺 We put Agentic RAG and Vanilla RAG to the test in answering questions about Weaviate. How the pipelines differ: Vanilla RAG: Simple retrieve, augment, and generate pipeline Agentic RAG: The LLM controls its own search strategy in a function calling loop…
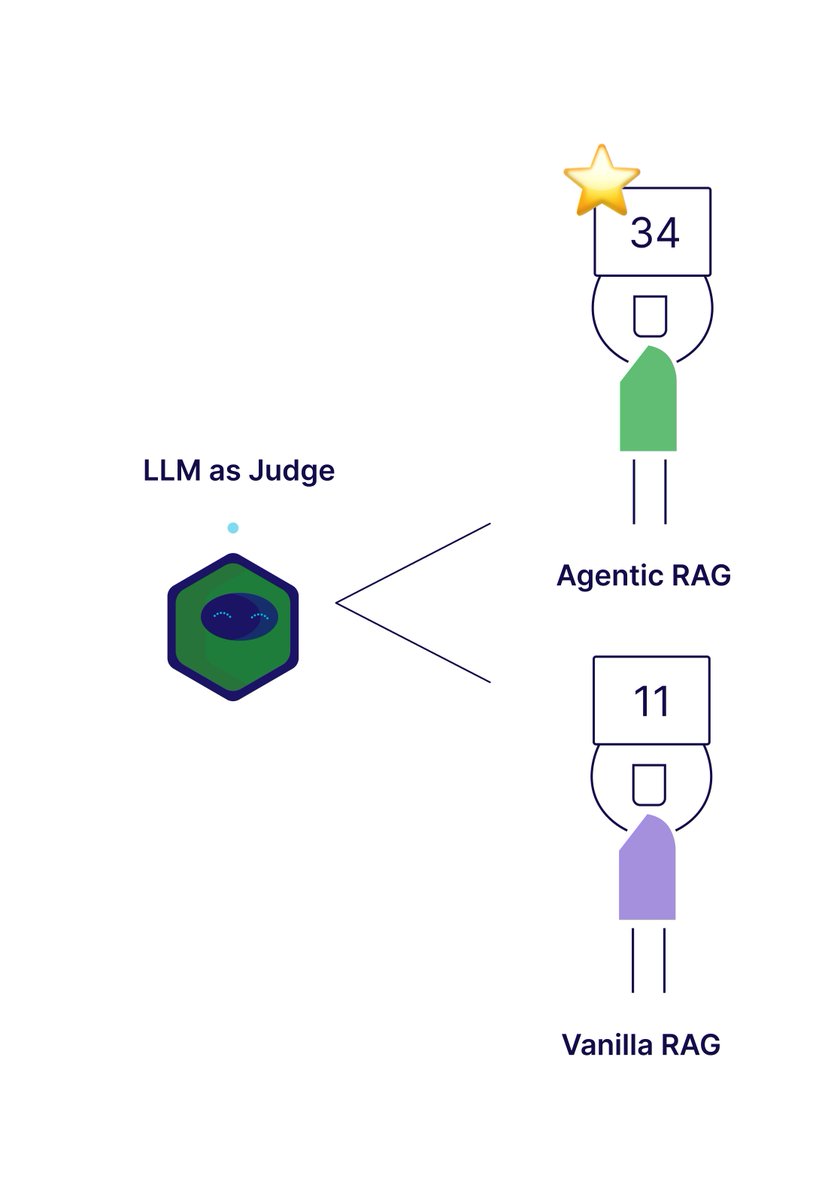
Dense but excellent. I literally have Spotify on my phone just for this series.
The Agentic RAG party continues! 🎉 I am SUPER EXCITED to publish the 109th Weaviate Podcast with Erika Cardenas (@ecardenas300)! Erika, in collaboration with Leonie Monigatti (@helloiamleonie), have recently published "What is Agentic RAG"! This blog has even been covered in…
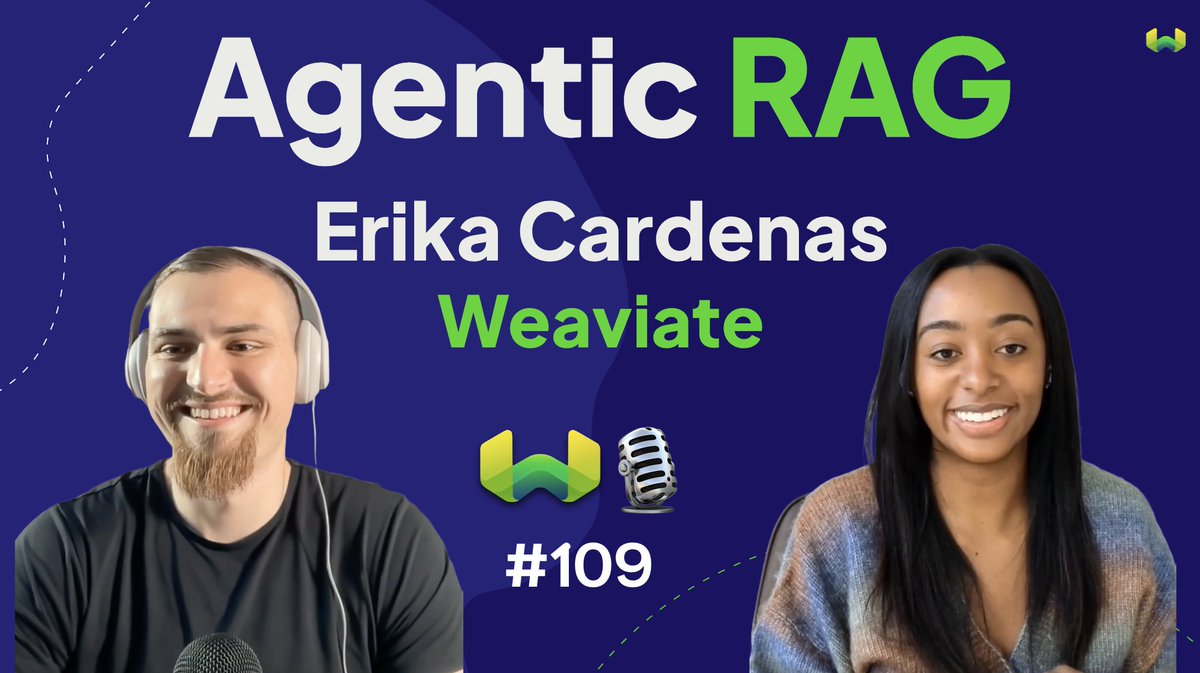
YouTube: youtube.com/watch?v=Eh4uQq… Spotify: podcasters.spotify.com/pod/show/weavi…
I love you @CShorten30 for making a video for JSON mode based on “Let Me Speak Freely” paper! ❤️ Must Watch! 🔥
JSON mode has been one of the biggest enablers for working with Large Language Models! JSON mode is even expanding into Multimodal Foundation models! But how exactly is JSON mode achieved? 🛠️ There are generally 3 paths to JSON mode: 🗺️ 1. Constrained generation (such as…
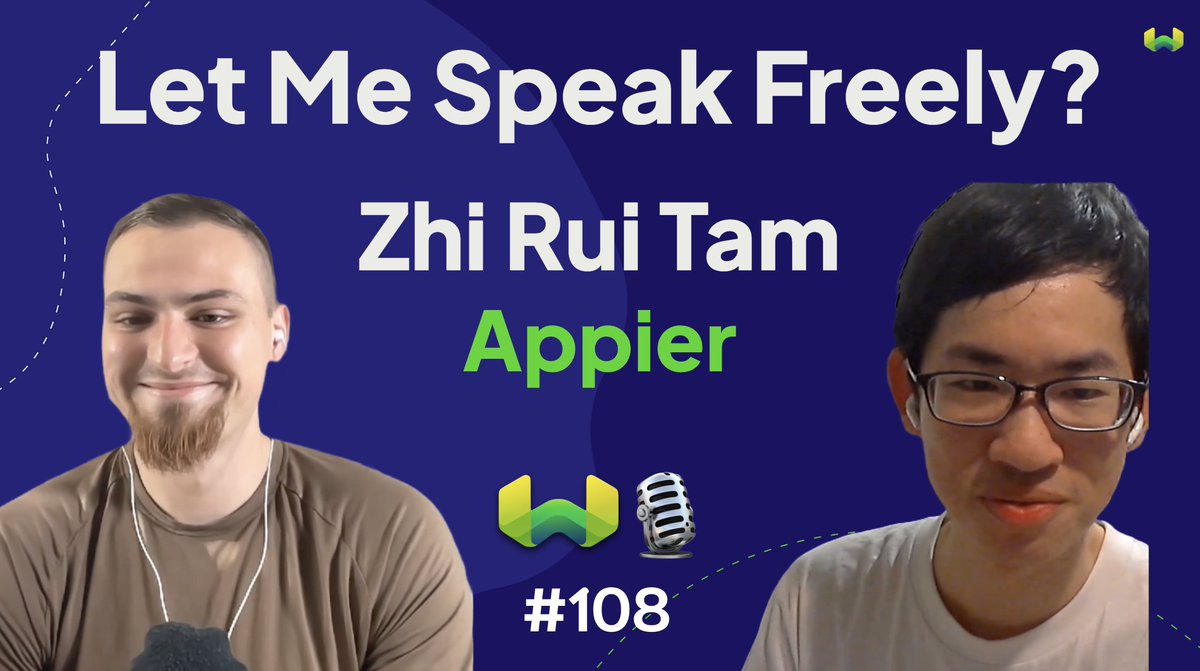
Swapping out RAG for Agentic RAG Systems 🤖 Agents differ from the standard retrieve and generate because of their access to tools, memory, and planning. Why is this game-changing? It allows us to build systems with complete autonomy to reason and execute specific tools when…
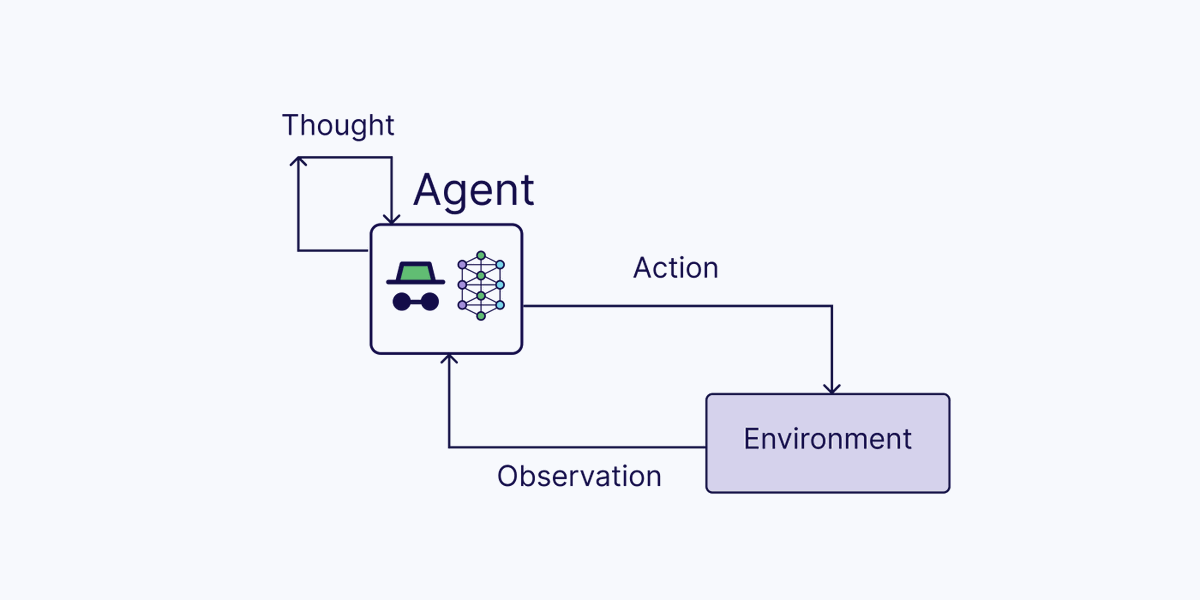
Goodbye, vanilla RAG. Hello, Agentic RAG! 𝗩𝗮𝗻𝗶𝗹𝗹𝗮 𝗥𝗔𝗚 The common vanilla RAG implementation processed the user query through a retrieval and generation pipeline to generate a response grounded in external knowledge. Advanced vanilla RAG techniques include e.g.,…
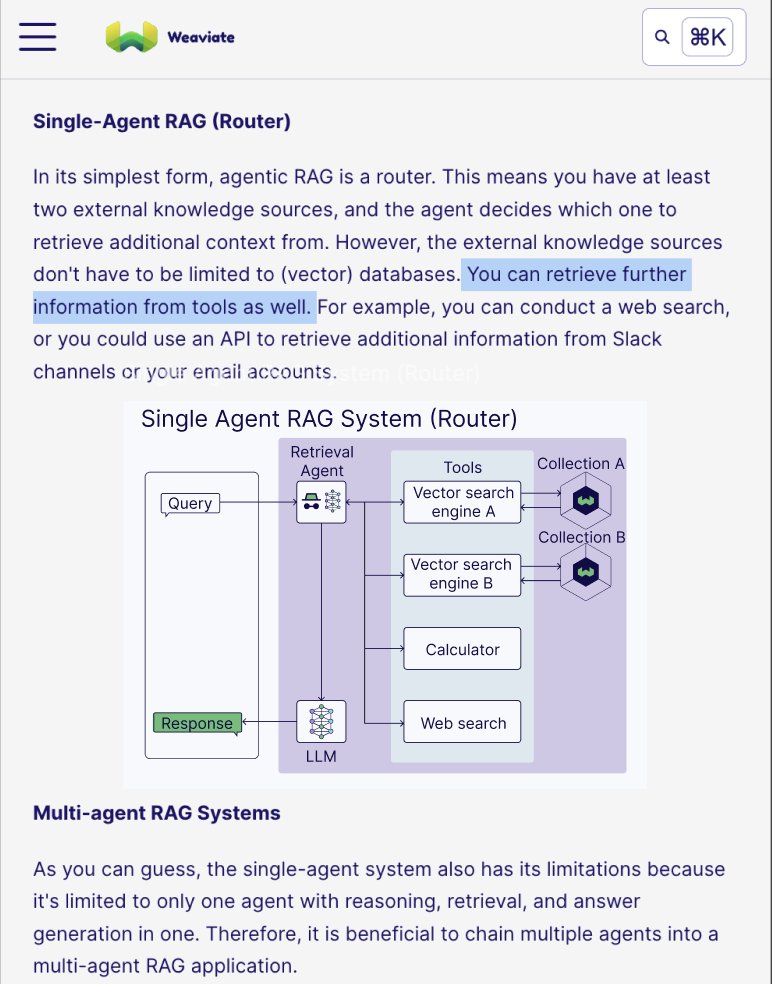
On Nov 19th 👉 Erika Cardenas @weaviate_io will be at our NYC event to share methods for optimizing agent systems. We also have experts from @Google @priceline @llama_index & AutoGen. Discover best practices for iterating on agent behavior through continuous evaluation and…
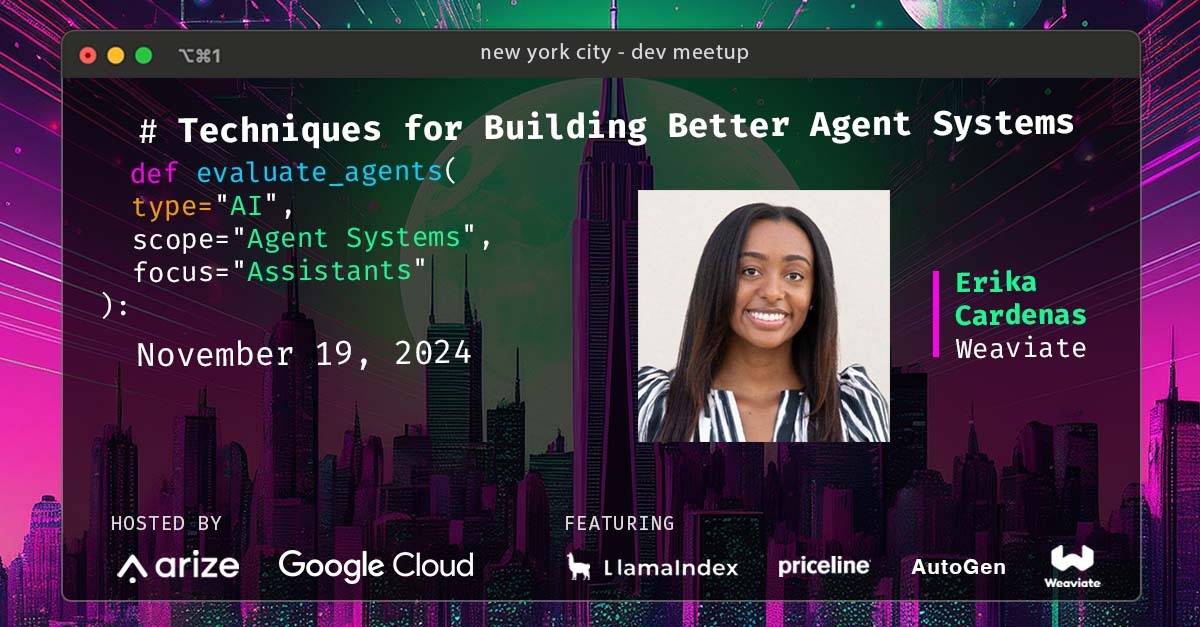
JSON mode has been one of the biggest enablers for working with Large Language Models! JSON mode is even expanding into Multimodal Foundation models! But how exactly is JSON mode achieved? 🛠️ There are generally 3 paths to JSON mode: 🗺️ 1. Constrained generation (such as…
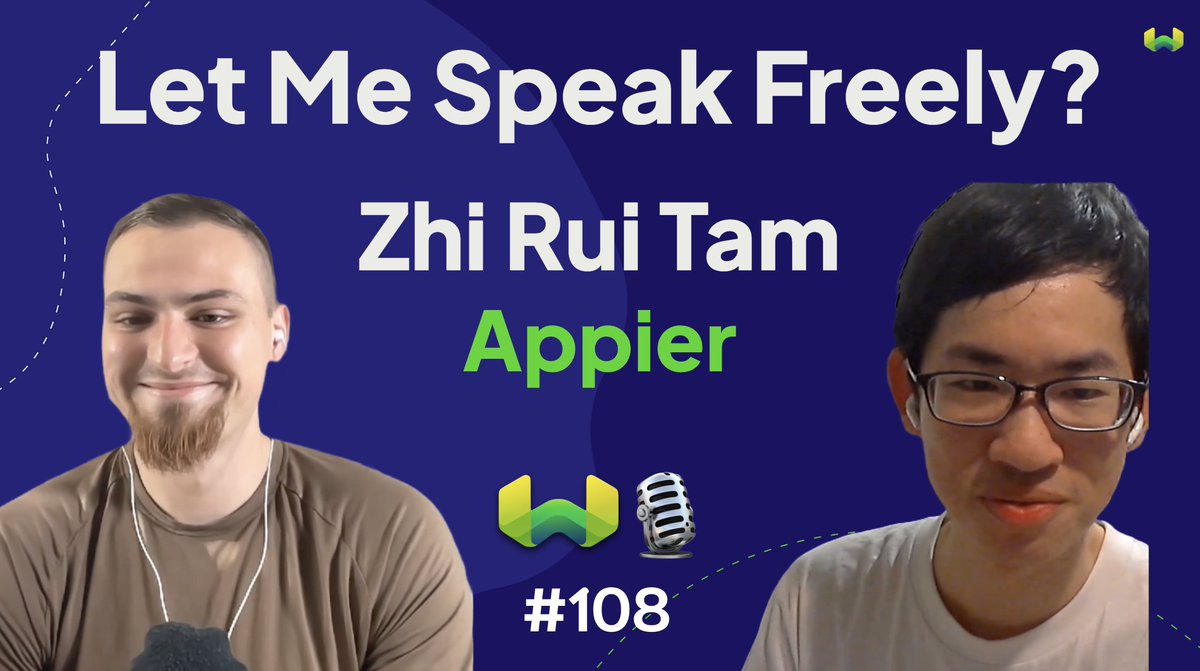
If interested, here is some research from the Weaviate team on this topic: StructuredRAG (Paper) - arxiv.org/abs/2408.11061 StructuredRAG (Repo) - github.com/weaviate/struc…
Long Context RAG Performance of Large Language Models Databricks analyzes 20 LLMs and reveals that only recent state-of-the-art models maintain consistent RAG accuracy above 64k tokens, with most models' performance declining at longer contexts. arxiv.org/abs/2411.03538
Phew… there are sooo many resources on RAG! It’s overwhelming 😩✊ Thankfully, my amazing colleagues ❤️ from @weaviate_io wrote down everything you need to know about Retrieval Augmented Generation! We have two great blog posts. The first one, by Mary, is all about the basics…
What makes an agentic system different from vanilla RAG? It’s access to memory and external tools. The building blocks of an agentic RAG system are: • LLM (with a role and a task) • Memory (short-term and long-term) • Planning (e.g., reflection, self-critics, query routing,…
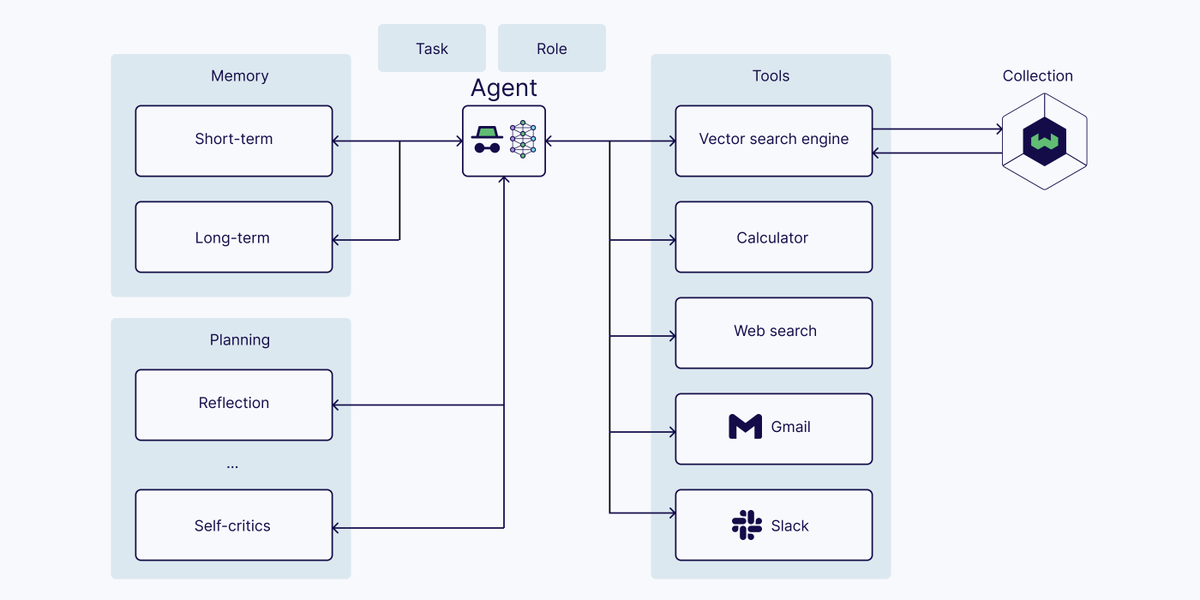
United States Trends
- 1. Tyson 528 B posts
- 2. Karoline 21 B posts
- 3. #wompwomp 6.925 posts
- 4. Syracuse 22,2 B posts
- 5. Paige 7.802 posts
- 6. Kiyan 26,6 B posts
- 7. Pence 61,7 B posts
- 8. Kash 107 B posts
- 9. The FBI 274 B posts
- 10. Debbie 35,8 B posts
- 11. Jarry N/A
- 12. Whoopi 117 B posts
- 13. #LetsBONK 16,6 B posts
- 14. Dora 24,7 B posts
- 15. #TOKKIVSWORLD 1.775 posts
- 16. Villanova 1.848 posts
- 17. White House Press Secretary 21,2 B posts
- 18. Ace Bailey N/A
- 19. Kyle Neptune N/A
- 20. Bucks County 98,2 B posts
Who to follow
-
Jessie de Groot
@jessie_groot -
Etienne Dilocker
@etiennedi -
Qdrant
@qdrant_engine -
Eric Zhang
@ekzhang1 -
Tuana
@tuanacelik -
Weaviate • vector database
@weaviate_io -
Zain
@ZainHasan6 -
Omar Khattab
@lateinteraction -
Lucia
@lucia_auth -
Abubakar Abid
@abidlabs -
Jo Kristian Bergum
@jobergum -
Bob van Luijt
@bobvanluijt -
Vaibhav (VB) Srivastav
@reach_vb -
Cerebras
@CerebrasSystems -
Avra
@Avra_b
Something went wrong.
Something went wrong.