
Mu Cai @ Industry Job Market
@MuCai7Ph.D. student @WisconsinCS, Multimodal Large Language Models Will graduate around 2025 May, looking for Research Scientist position around multimodal models.
Similar User

@xuefeng_du

@ZifengWang315

@Yang_Liuu

@jiefengchen1

@zihengh1

@yzeng58

@YiyouSun

@jzhao326

@blue75525366

@Changho_Shin_

@JiachenSun5

@Minghao__Yan

@zhmeishi

@jifan_zhang

@myhakureimu
Thanks for @_akhaliq 's sharing! (1/N) We propose M3: Matryoshka Multimodal Models, arxiv.org/abs/2405.17430 which (1) reduces the number of visual tokens significantly while maintaining as good performance as vanilla LMM (2) organizes visual tokens in a coarse-to-fine nested way.
Matryoshka Multimodal Models Large Multimodal Models (LMMs) such as LLaVA have shown strong performance in visual-linguistic reasoning. These models first embed images into a fixed large number of visual tokens and then feed them into a Large Language Model (LLM).
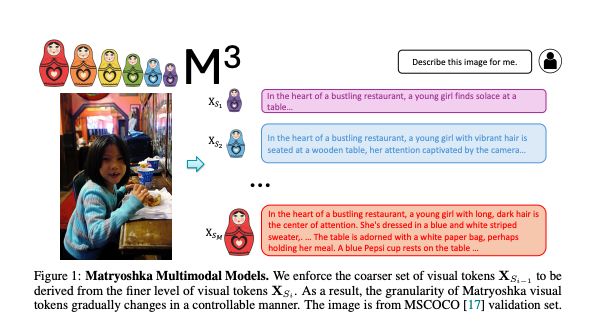
I am not in #EMNLP2024 but @bochengzou is in Florida! Go checkout vector graphics, a promising format that is completely different from pixels for visual representation. Thanks to LLMs, vector graphics are more powerful now! Go chat with @bochengzou if you are interested!
VGBench is accepted to EMNLP main conference! Congratulations to the team @bochengzou @HyperStorm9682 @yong_jae_lee The first work for "Evaluating Large Language Models on Vector Graphics Understanding and Generation" as a comprehensive benchmark!
Now TemporalBench is fully public! See how your video understanding model performs on TemporalBench before CVPR! 🤗 Dataset: huggingface.co/datasets/micro… 📎 Integrated to lmms-eval (systematic eval): github.com/EvolvingLMMs-L… (great work by @ChunyuanLi @zhang_yuanhan ) 📗 Our…
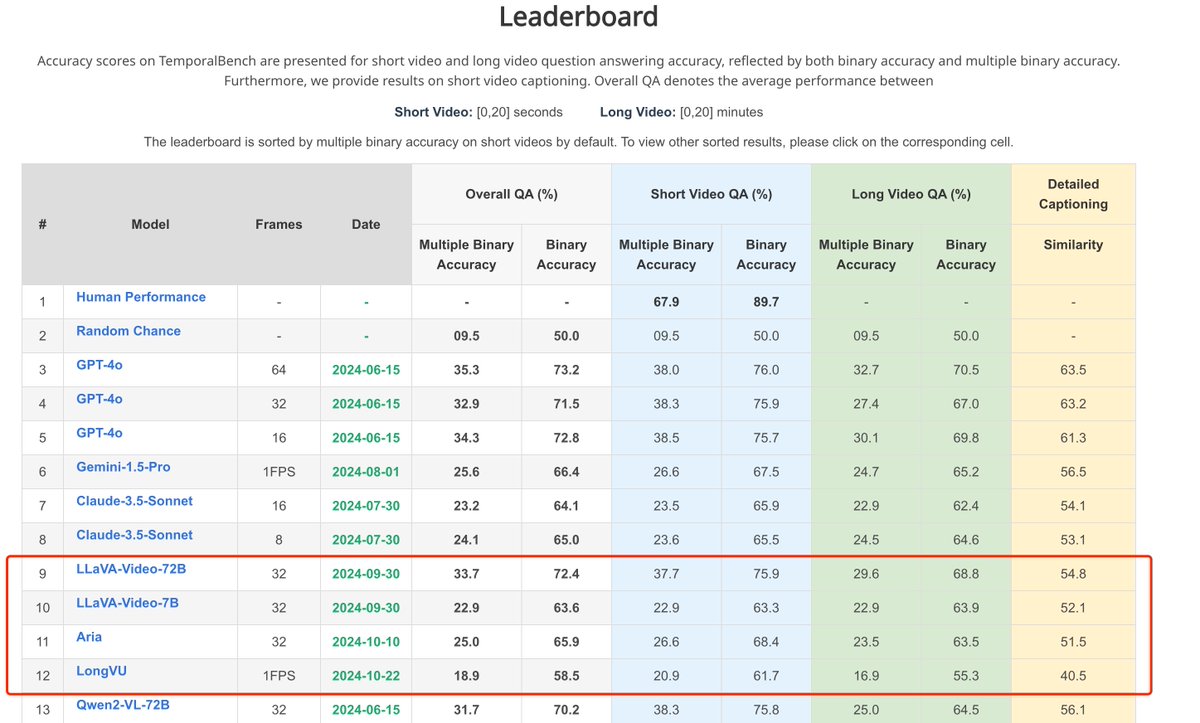
Personal update: After 5.5 yrs at @MSFTResearch , I will join @williamandmary in 2025 to be an assistant professor. Welcome to apply for my PhD/interns. Interest: ML with foundation models, LLM understanding, and AI for social sciences. More information: jd92wang.notion.site/Professor-Jind…
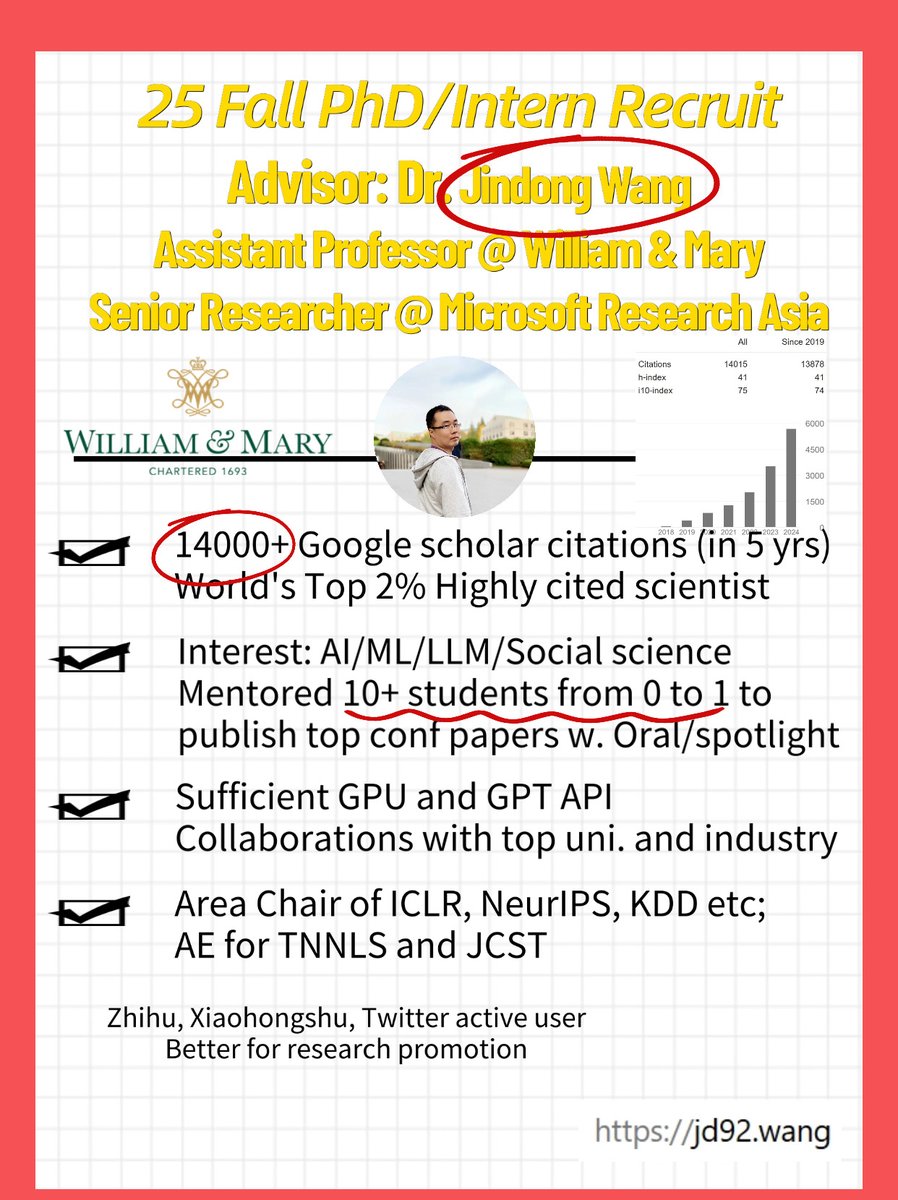
Fine-grained temporal understanding is fundamental for any video understanding model. Excited to see LLaVA-Video showing promising results on TemporalBench, @MuCai7! Yet, there remains a significant gap between the best model and human-level performance. The journey continues!
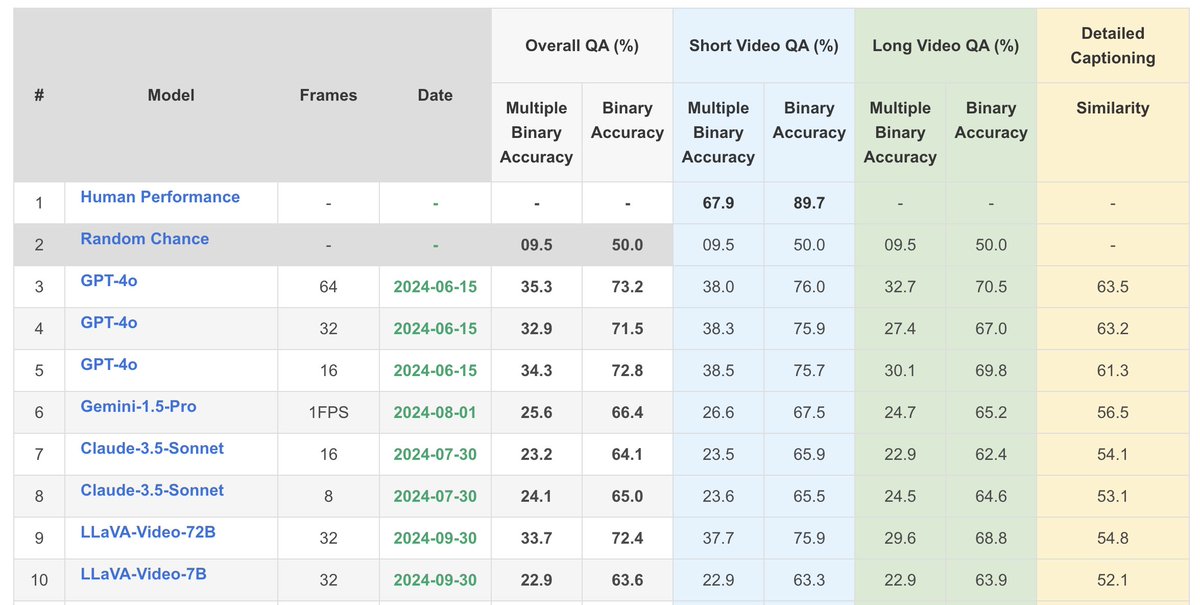
🔥Check out our new LMM benchmark TemporalBench! Our world is temporal, dynamic and physical, which can be only captured in videos. To move forward, we do need LMMs to understand the fine-grained changes and motions to really benefit the downstream applications such as video…
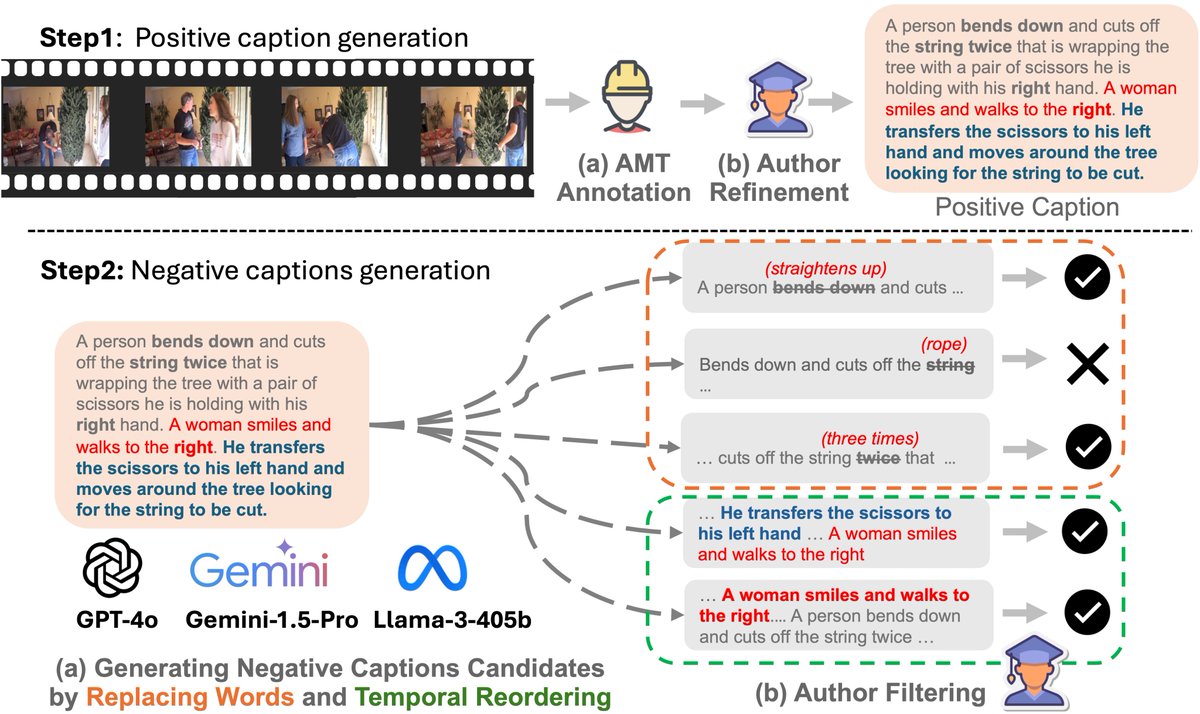
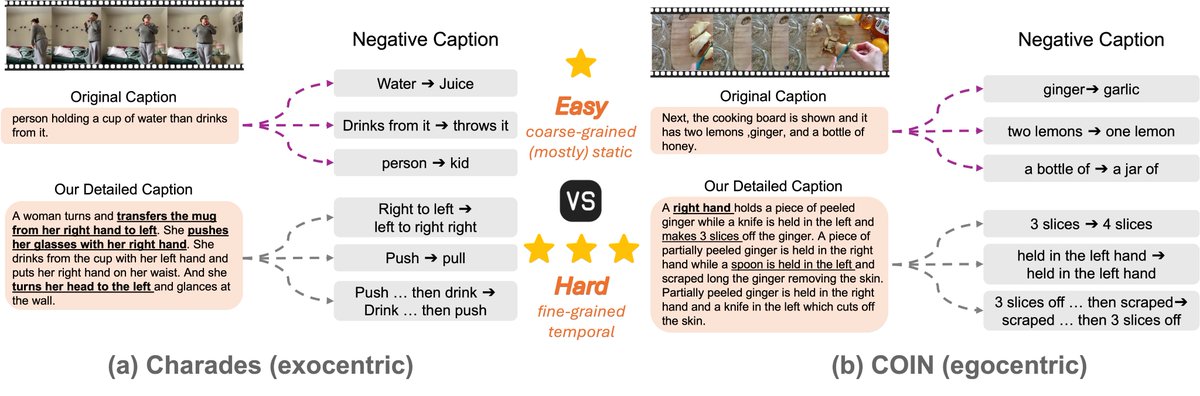
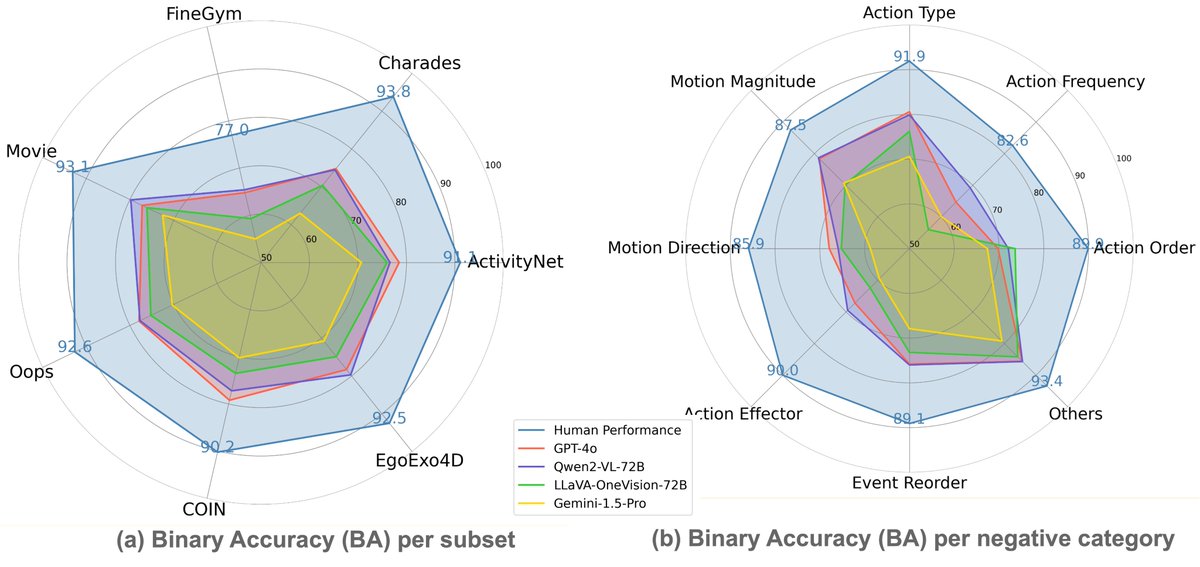
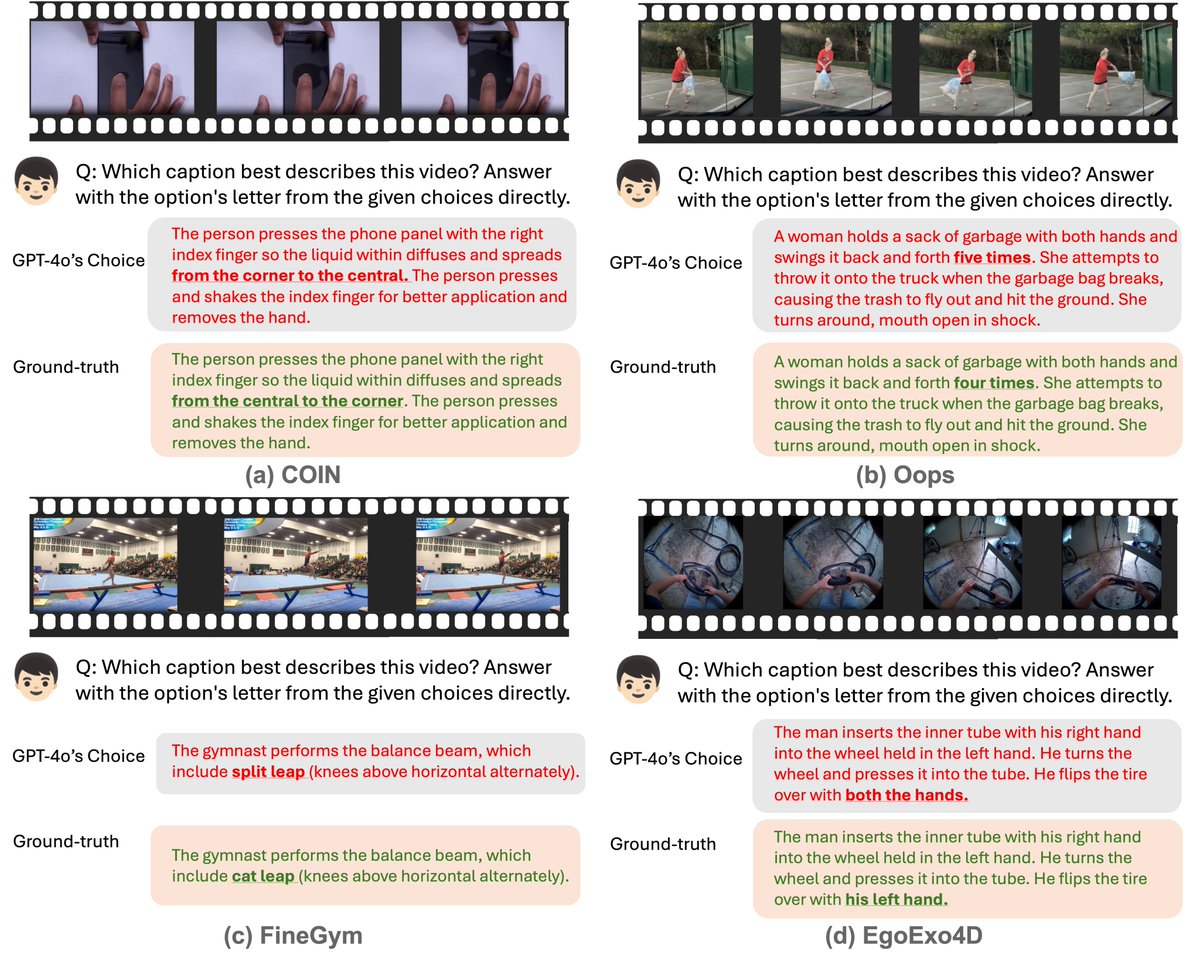
Great work on apply multi-granularity idea on image generation/manipulation! This share the same visual encoding design as our earlier work Matryoshka Multimodal Models (matryoshka-mm.github.io), where pooling is used to control visual granularity, leading to a multi…
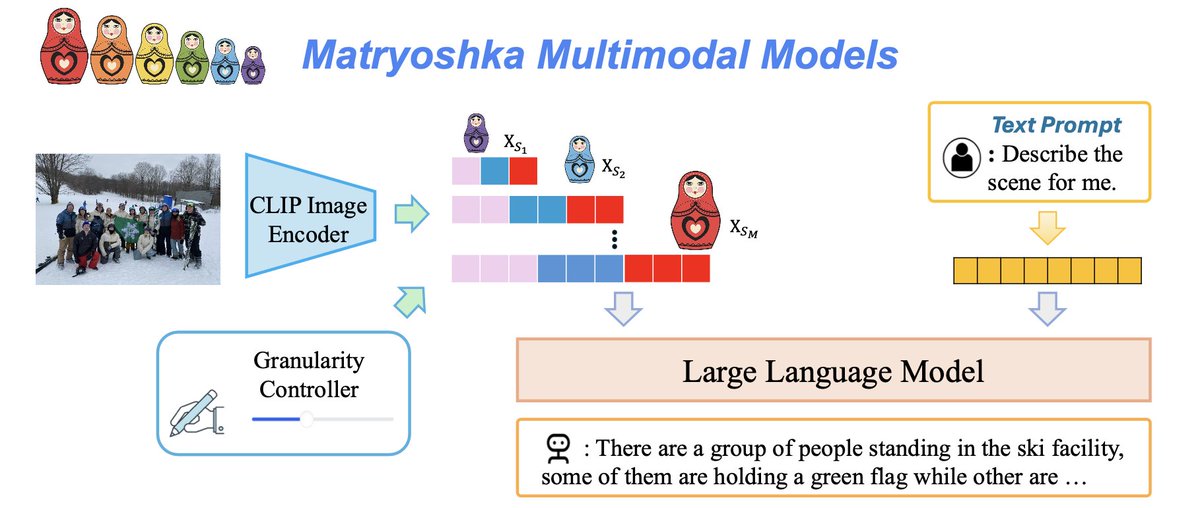
Introducing PUMA: a new MLLM for unified vision-language understanding and visual content generation at various granularities, from diverse text-to-image generation to precise image manipulation. rongyaofang.github.io/puma/ arxiv.org/abs/2410.13861 huggingface.co/papers/2410.13…
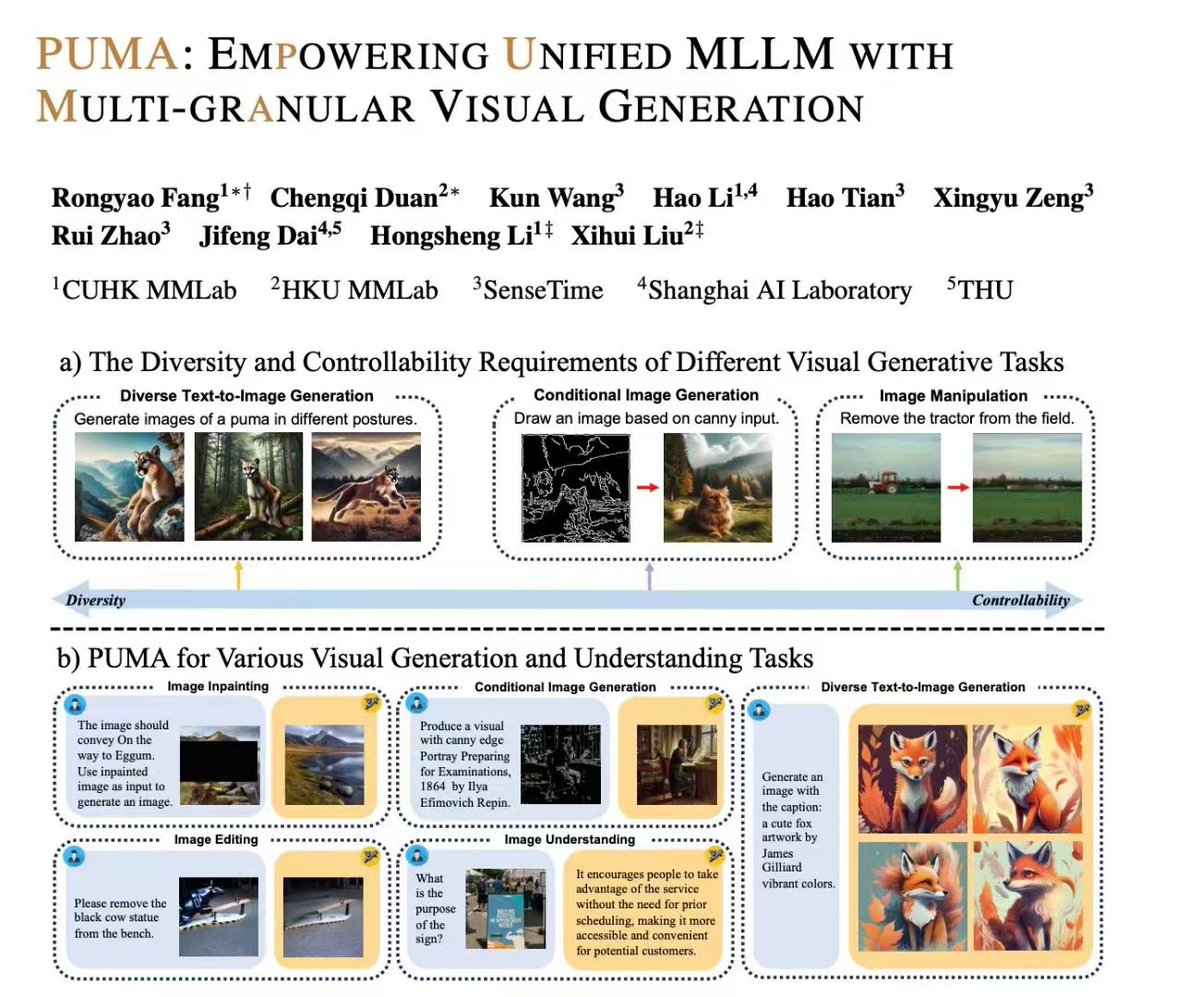
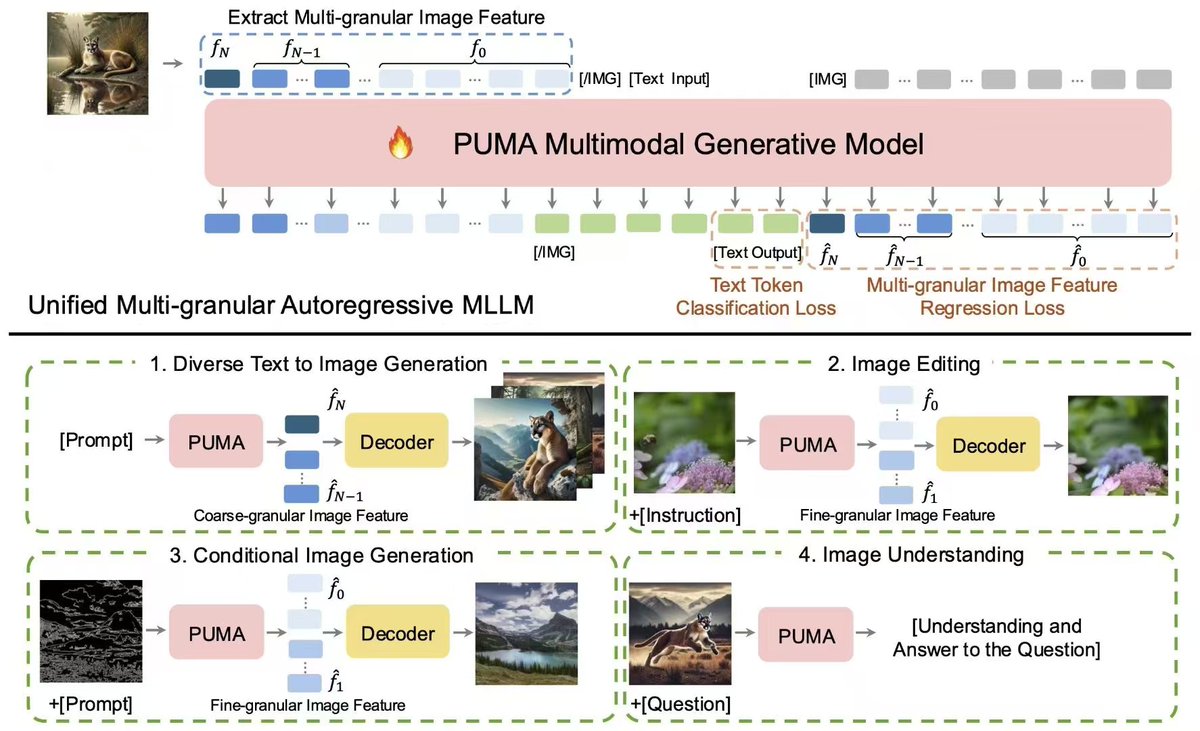
🔥Check out our new LMM benchmark TemporalBench! Our world is temporal, dynamic and physical, which can be only captured in videos. To move forward, we do need LMMs to understand the fine-grained changes and motions to really benefit the downstream applications such as video…
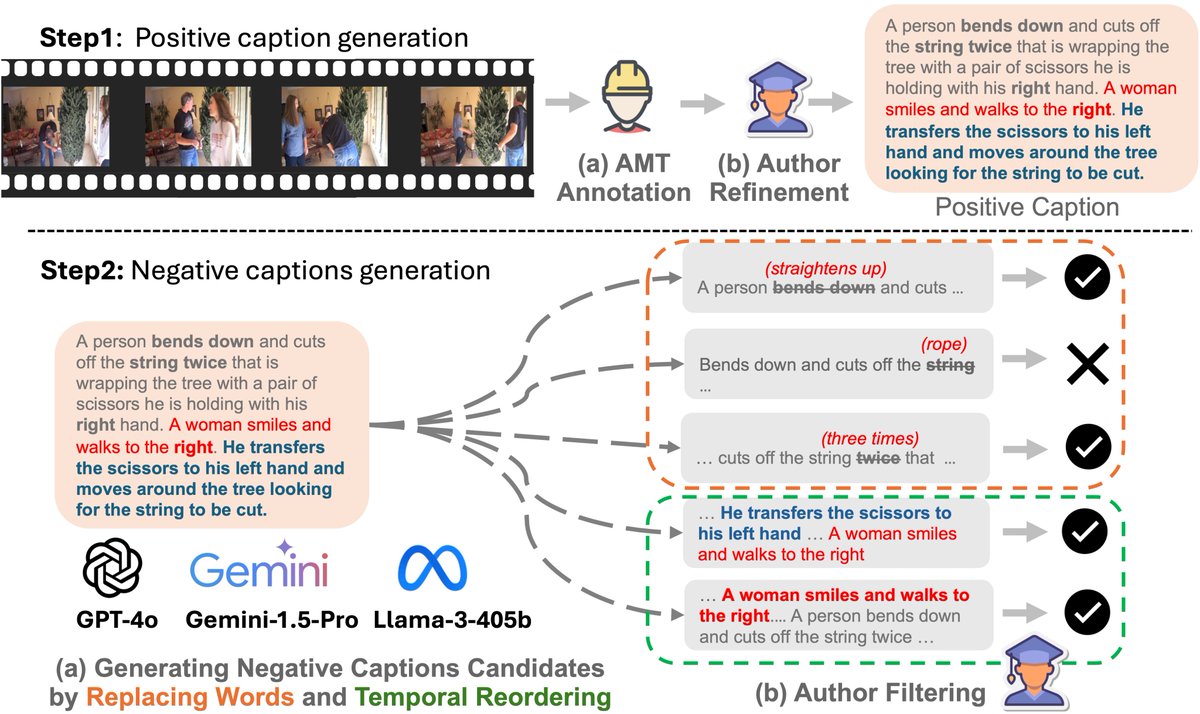
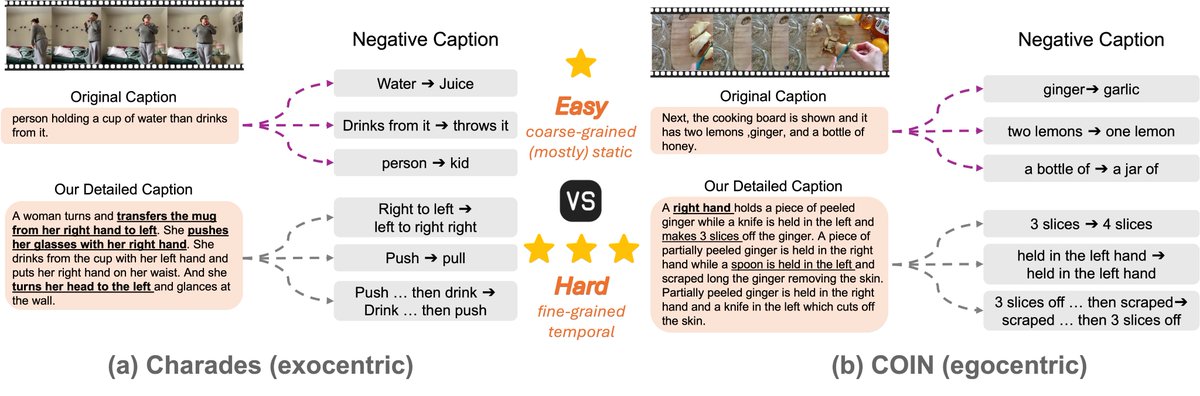
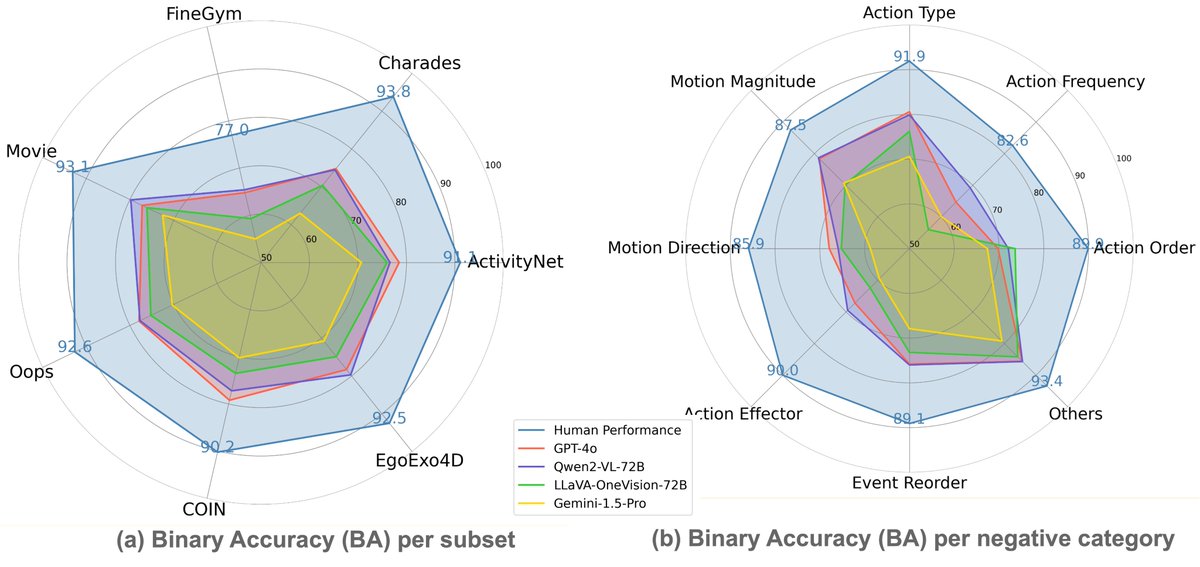
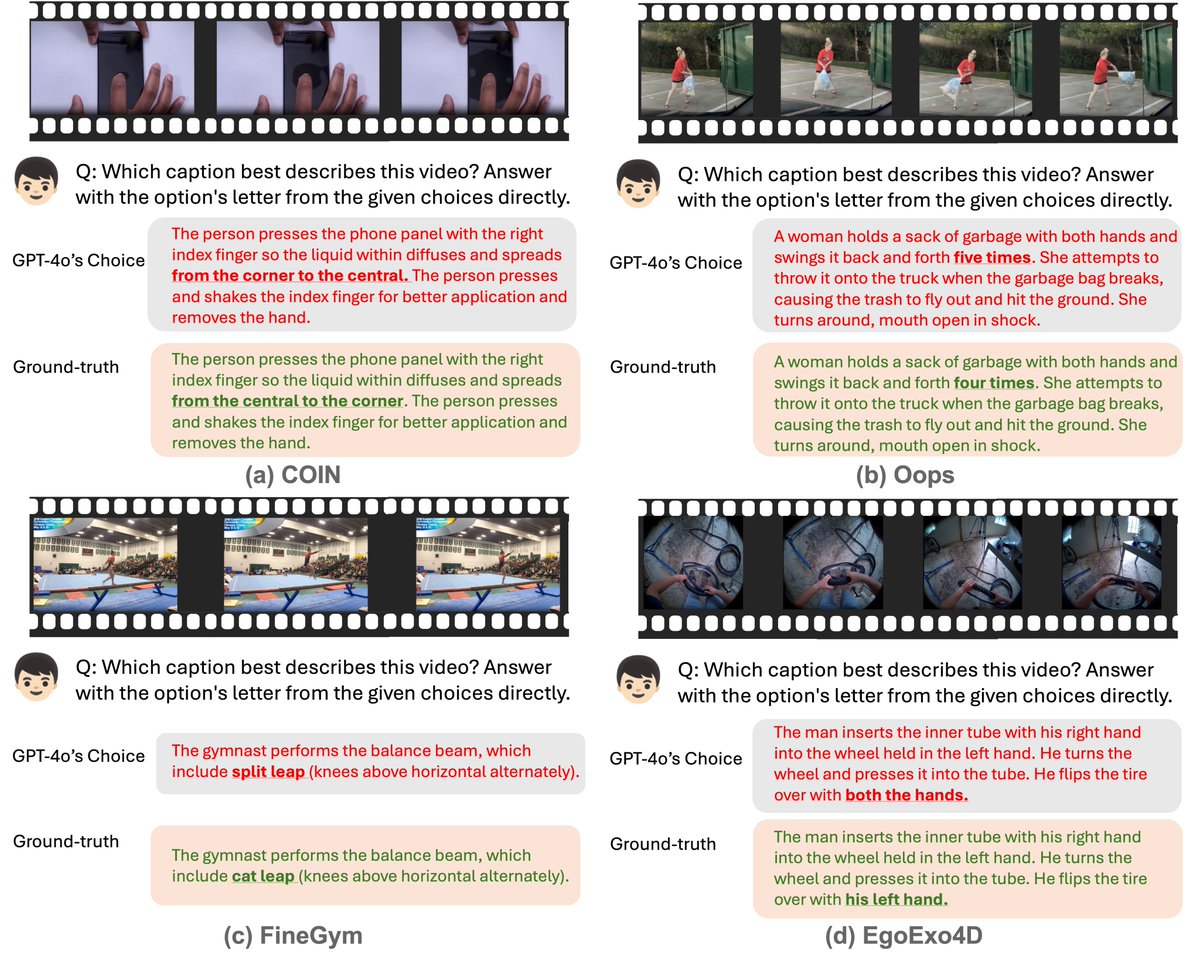
🚀 Excited to share our latest research: "Parameter-Efficient Fine-Tuning of SSMs" Summary: 🧵
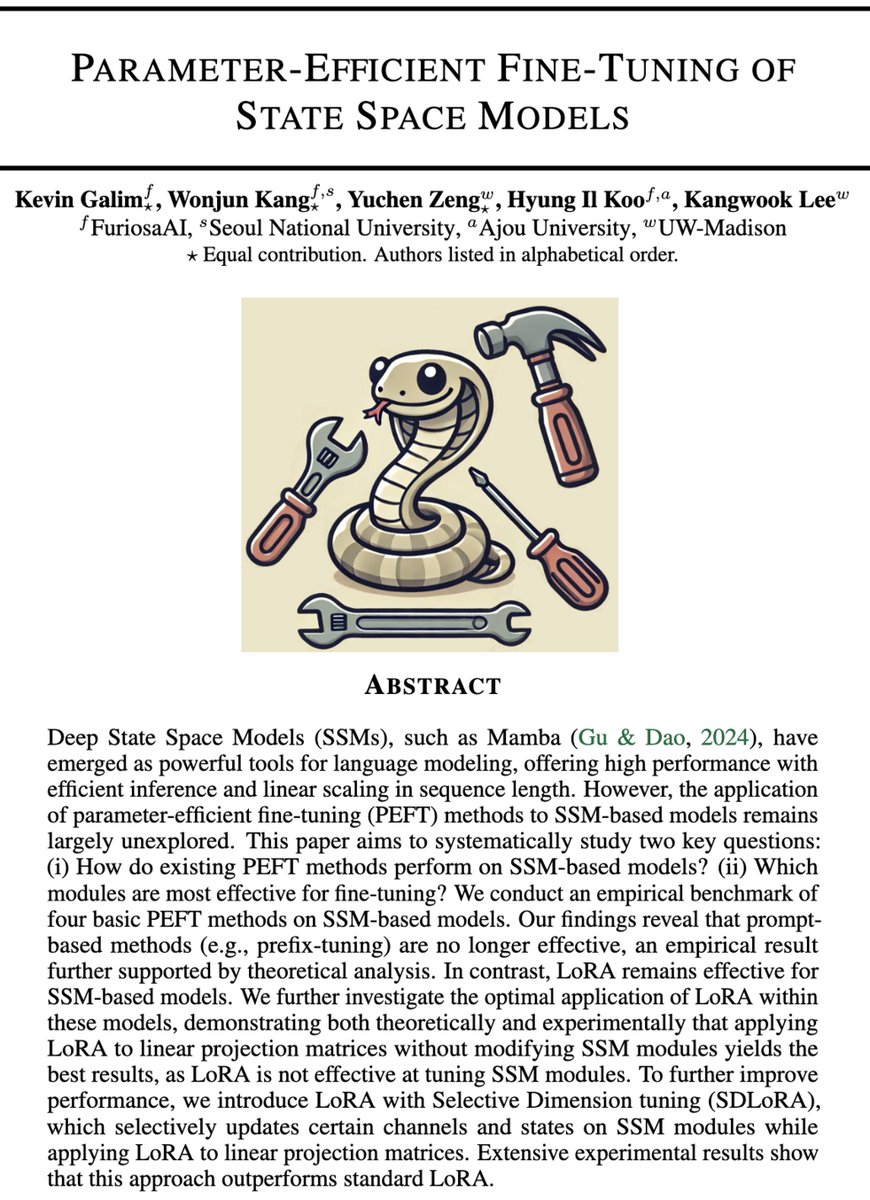
United States Trends
- 1. Travis Hunter 15,7 B posts
- 2. Clemson 7.176 posts
- 3. Colorado 70,7 B posts
- 4. Arkansas 28,3 B posts
- 5. Quinn 14,7 B posts
- 6. Dabo 1.216 posts
- 7. Cam Coleman 1.062 posts
- 8. Isaac Wilson N/A
- 9. #HookEm 3.194 posts
- 10. Northwestern 6.838 posts
- 11. #SkoBuffs 4.272 posts
- 12. $CUTO 8.261 posts
- 13. Sean McDonough N/A
- 14. Sark 1.948 posts
- 15. Tulane 2.807 posts
- 16. #NWSL N/A
- 17. Zepeda 1.813 posts
- 18. Mercer 4.278 posts
- 19. Pentagon 94,3 B posts
- 20. #iubb N/A
Who to follow
-
Sean Xuefeng Du (on academic job market)
@xuefeng_du -
Zifeng Wang
@ZifengWang315 -
Liu Yang
@Yang_Liuu -
Jiefeng Chen
@jiefengchen1 -
Tzu-Heng Huang
@zihengh1 -
Yuchen Zeng
@yzeng58 -
Yiyou Sun
@YiyouSun -
Jitian Zhao
@jzhao326 -
Boru Chen
@blue75525366 -
Changho Shin
@Changho_Shin_ -
Jiachen Sun
@JiachenSun5 -
Minghao Yan
@Minghao__Yan -
Zhenmei SHI
@zhmeishi -
Jifan Zhang
@jifan_zhang -
Ziqian Lin
@myhakureimu
Something went wrong.
Something went wrong.