
Charles Curt
@CharlesCurt2humble attempt at AGI any size - https://t.co/ShDGOYMf6F
Reddit has fallen so far. The front page has 7! Direct pictures of tweets from X.
Scaling laws 100% will work just fine. The real question is, can we continue it economically. We are so many orders of magnitude away from agents replacing humans in all but the most encapsulated jobs.
If you actually spent time studying how these systems handle depth, you would have seen well over a year ago that this was going to happen. Exponential compute requirements can not go on forever. I did a study on the original LLama models and found this effect in early 2023.…
Ilya Sutskever, perhaps the most influential proponent of the AI "scaling hypothesis," just told Reuters that scaling has plateaued. This is a big deal! This comes on the heels of a big report that OpenAI's in-development Orion model had disappointing results. 🧵
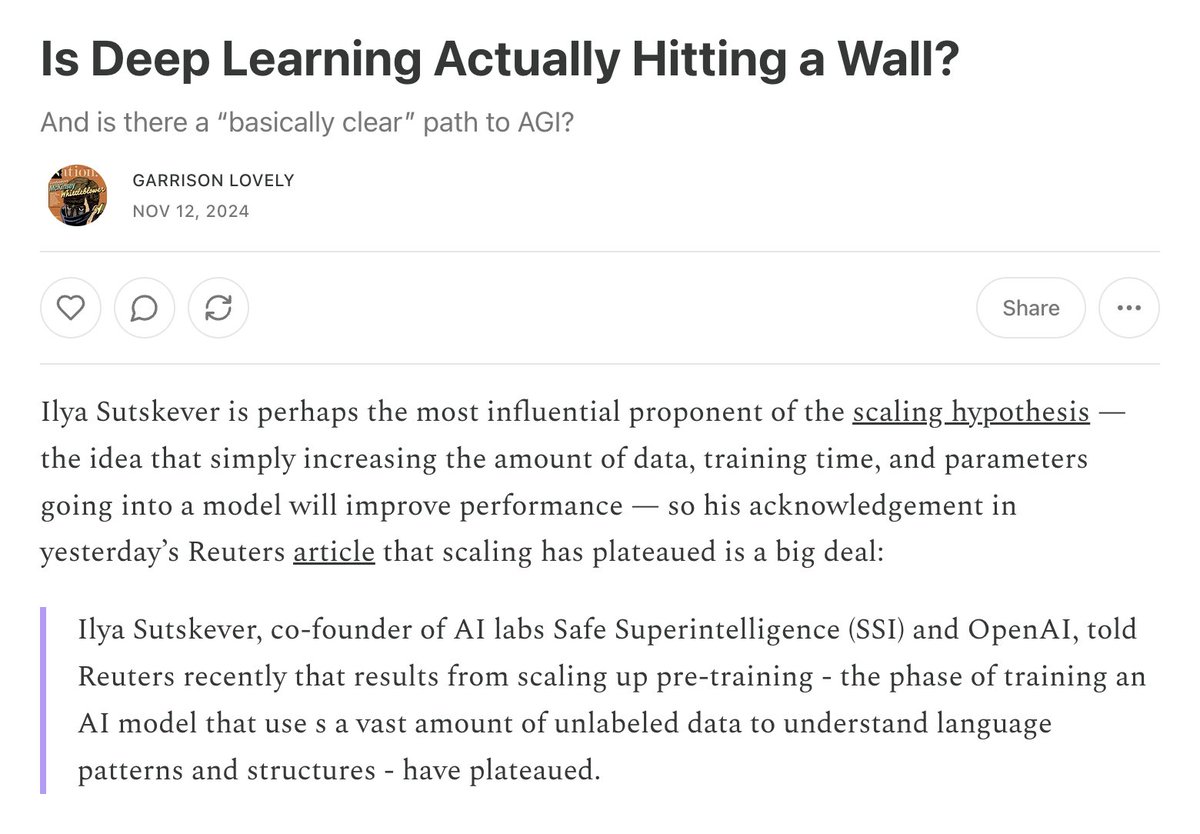
How far are we to AGI? Go here select a problem: huggingface.co/datasets/gaia-… Time yourself (4mins) Our best methods are right around 1/3 of the time. A lazy workday may be ~4 hours of similar tasks, ~60 tasks. So 0.33^60 = 1.3^-29 chance AI could do your job for a single day.
Open AI's o1 is an incredible advancement. I generated this guide for Adam Optimizers with three prompts. 1. information (4 secs) 2. Latex (couple secs) 3. Detailed formatting (128secs) archive.org/details/adam_2…
Studying how learning rates and memory formation occur in LLMs is a nuanced area. I studied rare memory formations in LLMs and found that LLMs can remember things even in minimal training numbers. (sometimes remembering < 0.2% of the time) sliced-ai.com/papers/lr_nad_…
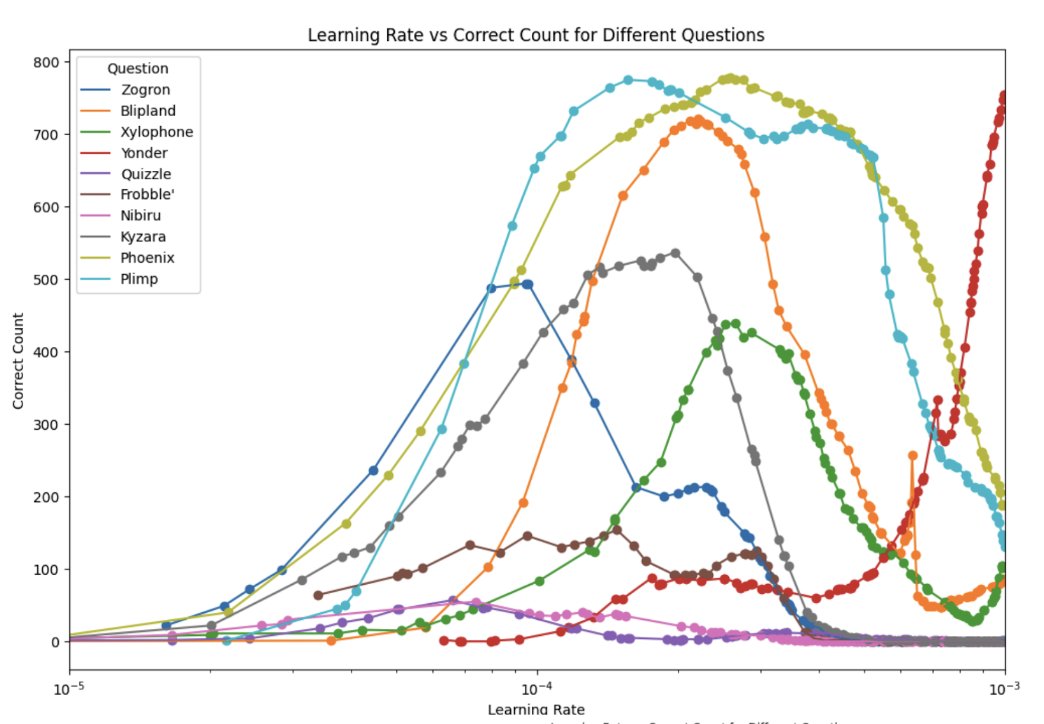
I ran thousands of inferences on different models on the same prompt, varying the temperature, top_p, and generation length. I found that these variables do far less than you think they do. sliced-ai.com/papers/thousan…
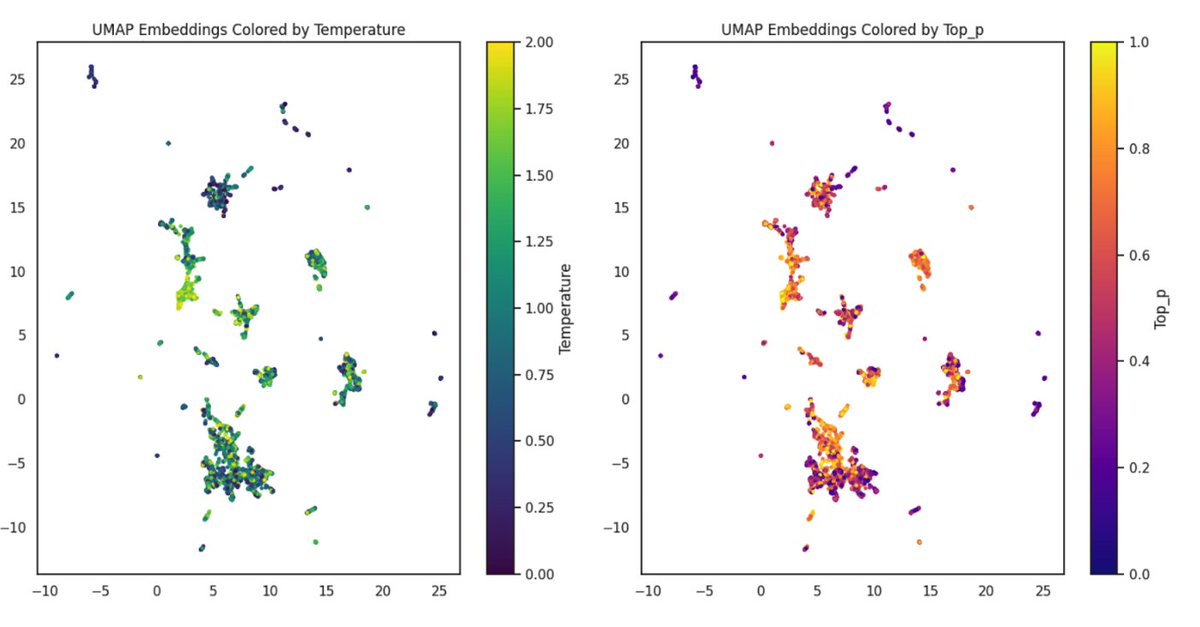
Expanding Embedding Spaces: How do we build long-running processes for LLMs using either RAG or CL when the internal spaces of our models are fixed? This work focuses on methods to expand that space to allow longer-running systems. sliced-ai.com/papers/ae_embe…
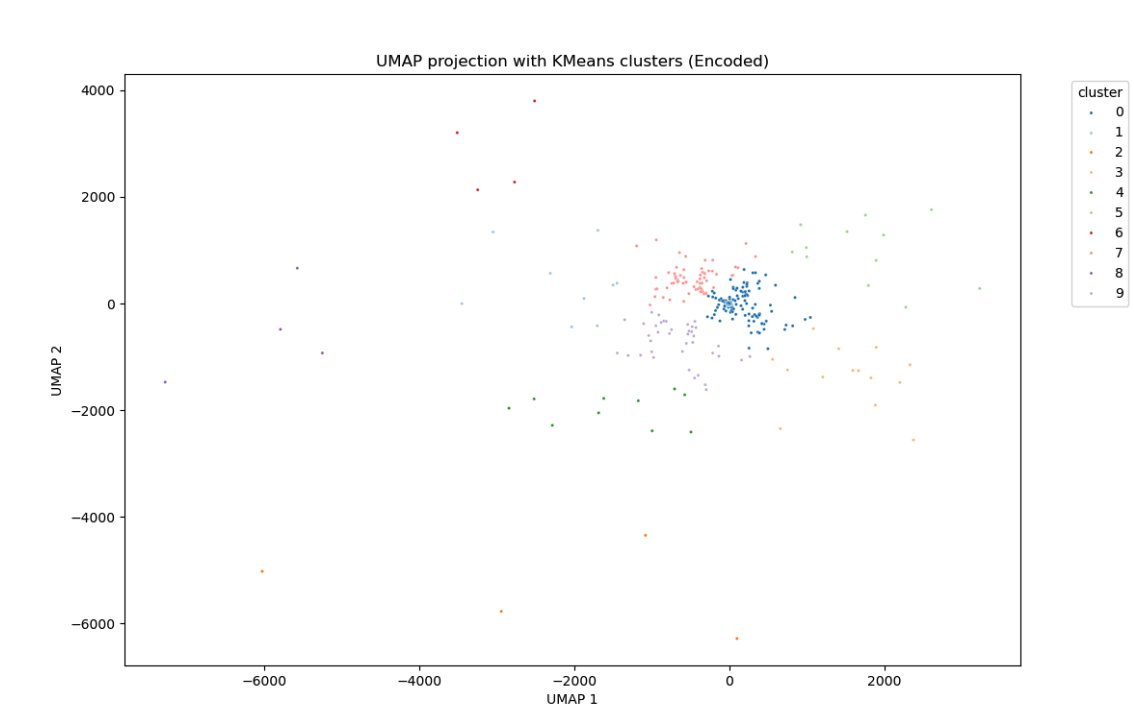
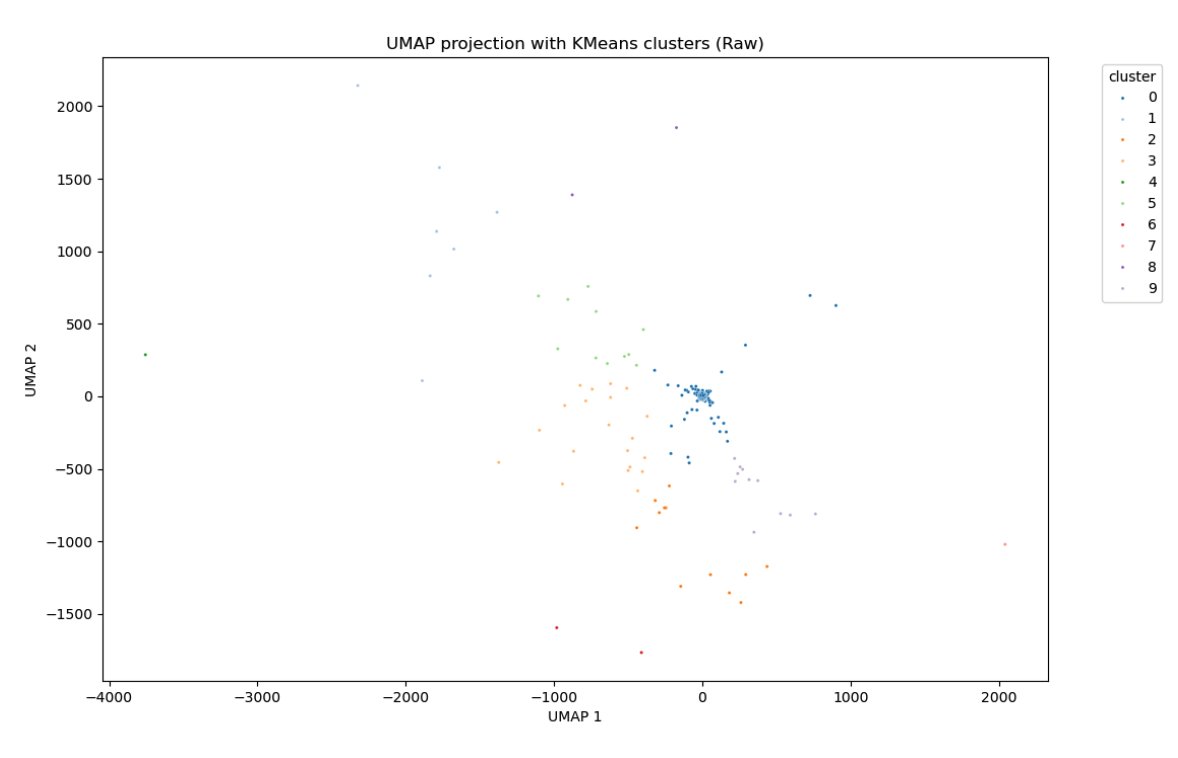
Ok, so I'm not crazy GPT4o is really good on larger problems. Everyone is saying it's worse, but I'm seeing massive improvement. nian.llmonpy.ai
Is it a strategy of large AI companies to say that only they can work on AGI, preventing competition? Only they can do it because it requires infinite computing. I believe real AGI will be at all sizes.
we're like one single development away from recursively self-improving AI. It doesn't even seem super difficult to set up an agent which can run training batches on itself + modify synthetic training data. Is anyone doing this? Would I be irresponsible to try?
Will infinite context injection or continual learning win the long-term AI value race? My prediction: Infinite context wins for massive low context problems. (Solve x) CL wins for agent/edge high context problems (Become x)
Been working on a new company with the core arguments based on these recent articles I wrote. I'm trying to develop a new method for AI agents, give it a read! medium.com/@charles.curt/…
United States Trends
- 1. Mike 1,73 Mn posts
- 2. #Arcane 174 B posts
- 3. Serrano 243 B posts
- 4. Jayce 30,4 B posts
- 5. vander 8.909 posts
- 6. Canelo 17,2 B posts
- 7. maddie 14,3 B posts
- 8. #NetflixFight 74,7 B posts
- 9. Jinx 85,6 B posts
- 10. Logan 78,8 B posts
- 11. Father Time 10,5 B posts
- 12. He's 58 28,6 B posts
- 13. #netflixcrash 16,7 B posts
- 14. Boxing 308 B posts
- 15. ROBBED 99,7 B posts
- 16. Rosie Perez 15,2 B posts
- 17. #buffering 11,2 B posts
- 18. Tori Kelly 5.425 posts
- 19. Isha 22,5 B posts
- 20. Shaq 16,7 B posts
Something went wrong.
Something went wrong.